Predicted Edit Distance Based Clustering Of Gene Sequences
2018 IEEE INTERNATIONAL CONFERENCE ON DATA MINING (ICDM)(2018)
摘要
Effective mining of huge amount of DNA and RNA fragments generated by next generation sequencing (NGS) technologies is facilitated by developing efficient tools to partition these sequence fragments (reads) based on their level of similarities using edit distance. However, edit distance calculation for all pairwise sequence fragments to cluster these huge data sets is a significant performance bottleneck. In this paper we propose a predicted Edit distance based clustering to significantly lower clustering time. Existing clustering methods for sequence fragments, such as, k-mer based VSEARCH and Locality Sensitive Hash based LSH-Div achieve much reduced clustering time but at the cost of significantly lower cluster quality. We show, through extensive performance analysis, clustering based on this predicted Edit distance provides more than 99% accurate clusters while providing an order of magnitude faster clustering time than actual Edit distance based clustering.
更多查看译文
关键词
Edit distance prediction,OTU clustering
AI 理解论文
溯源树
样例
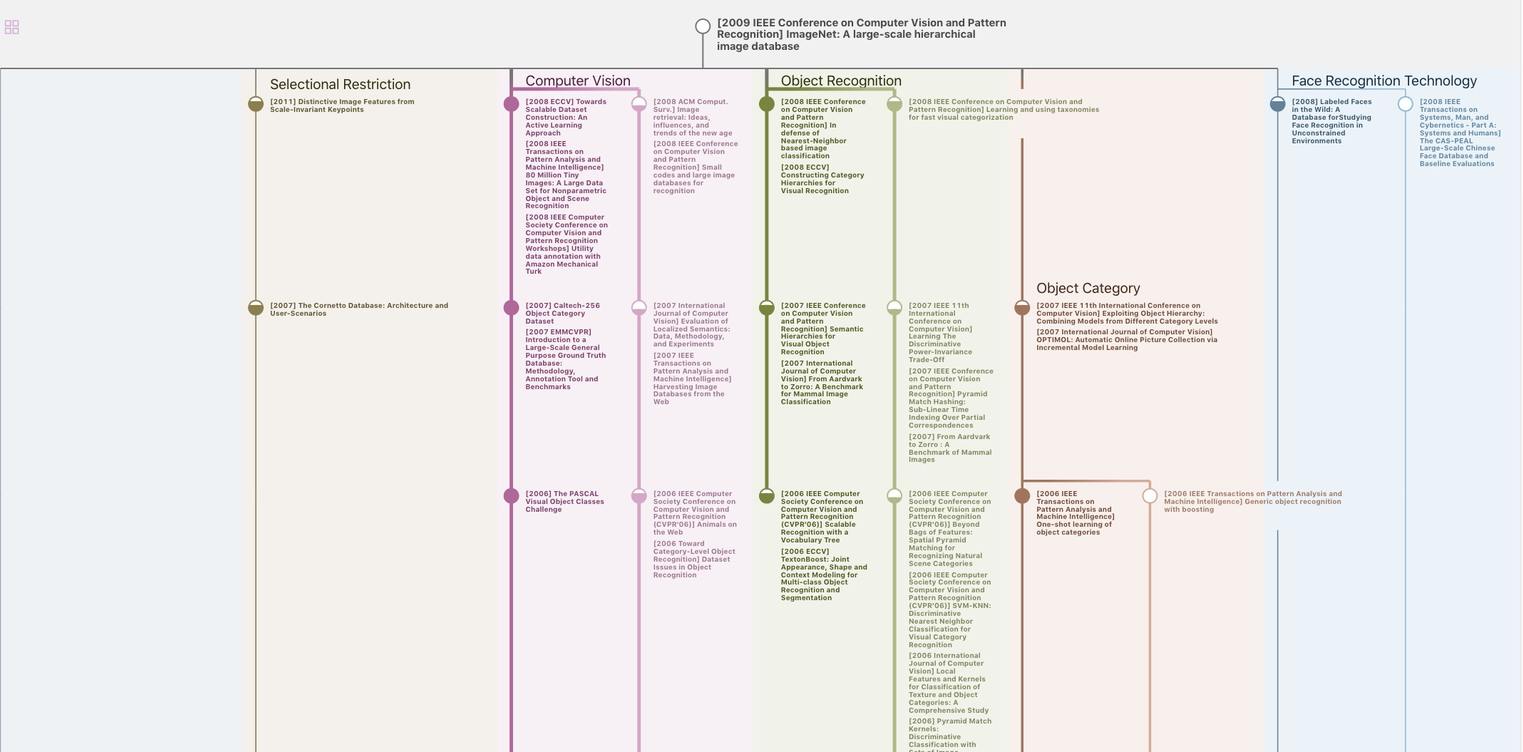
生成溯源树,研究论文发展脉络
Chat Paper
正在生成论文摘要