Imbalanced Augmented Class Learning with Unlabeled Data by Label Confidence Propagation
2018 IEEE International Conference on Data Mining (ICDM)(2018)
摘要
As a practical problem in open and dynamic environments, class-incremental learning has attracted much attention from many fields. Learning with augmented class (LAC) problem formulates one of the core difficulties of class-incremental learning: instances of augmented class need to be predicted with the restriction that only examples from seen classes are observed in training phase. LACU framework advances the study of LAC problem by exploiting unlabeled data, while it does not take into account an important practical problem widely-existing in real-world applications of LAC - imbalanced class distributions among seen classes, which will further increase the learning difficulties of LAC problem. We propose a novel approach Label Confidence Propagation (LCP) to tackle the problem of imbalanced augmented class learning with unlabeled data. LCP enlarges the labeled training data set by estimating class labels for unlabeled data, to meet the challenge of lacking supervision information of augmented classes via identifying some of their instances, and to alleviate the damage of class-imbalance via identifying more instances for each seen class. LCP firstly initializes label confidence, i.e., the posterior probability distributions of all classes (including augmented classes) for unlabeled data, then iteratively propagates label confidence to identify a valid label for each unlabeled instance to enlarge the labeled training data set. Finally, LCP predicts for unseen instances by linear neighborhood reconstruction to be robust to potential noise. Results on abundant experiments show that LCP is significantly superior to many state-of-the-art methods, and robust to high imbalance ratio and high open level. LCP can sufficiently unleash its strength especially when there are abundant unlabeled data available.
更多查看译文
关键词
augmented class learning,LACU framework,class imbalance,unlabeled data
AI 理解论文
溯源树
样例
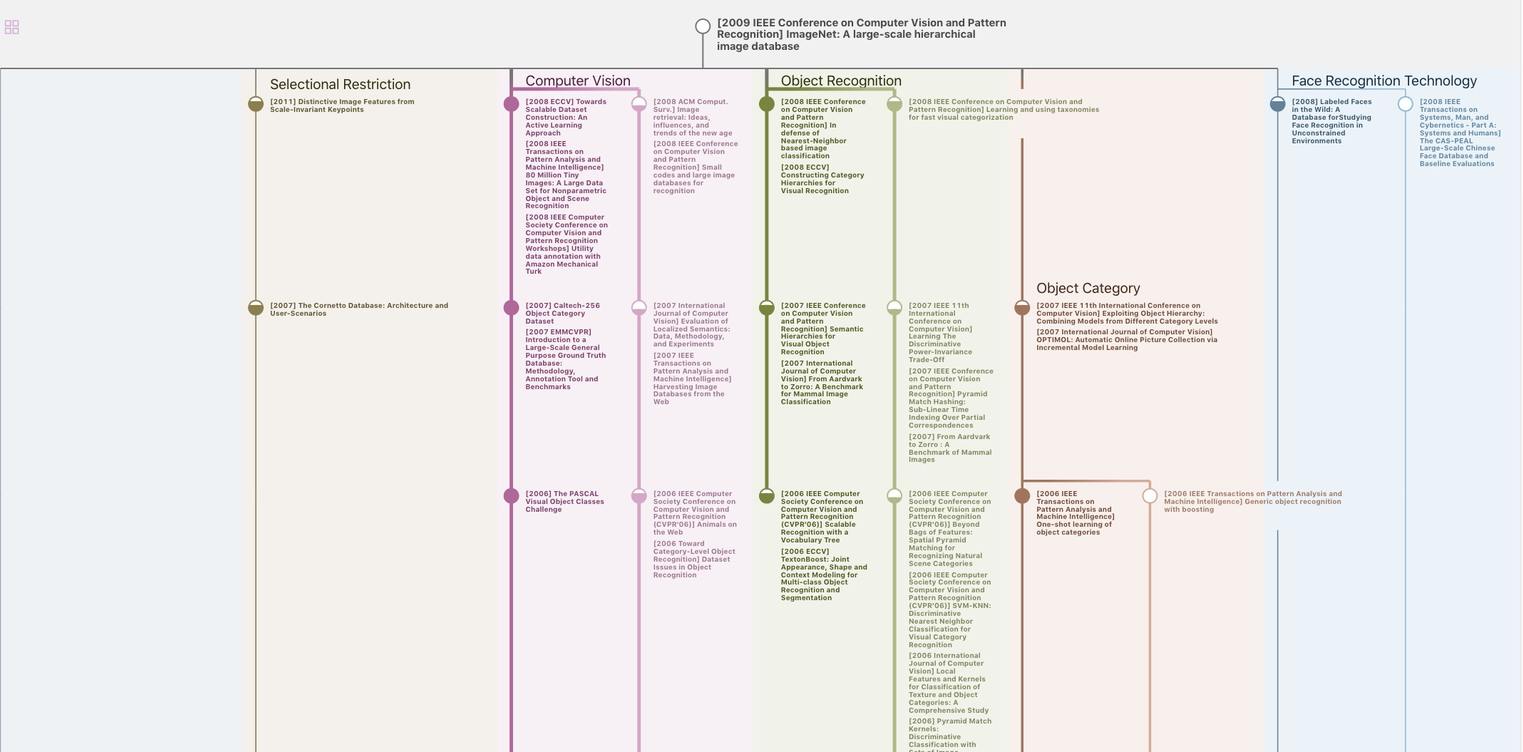
生成溯源树,研究论文发展脉络
Chat Paper
正在生成论文摘要