Using Machine Learning to Distinguish Infected from Non-infected Subjects at an Early Stage Based on Viral Inoculation.
DILS(2018)
摘要
Gene expression profiles help to capture the functional state in the body and to determine dysfunctional conditions in individuals. In principle, respiratory and other viral infections can be judged from blood samples; however, it has not yet been determined which genetic expression levels are predictive, in particular for the early transition states of the disease onset. For these reasons, we analyse the expression levels of infected and non-infected individuals to determine genes (potential biomarkers) which are active during the progression of the disease. We use machine learning (ML) classification algorithms to determine the state of respiratory viral infections in humans exploiting time-dependent gene expression measurements; the study comprises four respiratory viruses (H1N1, H3N2, RSV, and HRV), seven distinct clinical studies and 104 healthy test candidates involved overall. From the overall set of 12,023 genes, we identified the 10 top-ranked genes which proved to be most discriminatory with regards to prediction of the infection state. Our two models focus on the time stamp nearest to (t = 48) hours and nearest to (t =) “Onset Time” denoting the symptom onset (at different time points) according to the candidate’s specific immune system response to the viral infection. We evaluated algorithms including k-Nearest Neighbour (k-NN), Random Forest, linear Support Vector Machine (SVM), and SVM with radial basis function (RBF) kernel, in order to classify whether the gene expression sample collected at early time point t is infected or not infected. The “Onset Time” appears to play a vital role in prediction and identification of ten most discriminatory genes.
更多查看译文
关键词
Machine learning, Respiratory viral infection, Prediction, Deferentially expressed genes
AI 理解论文
溯源树
样例
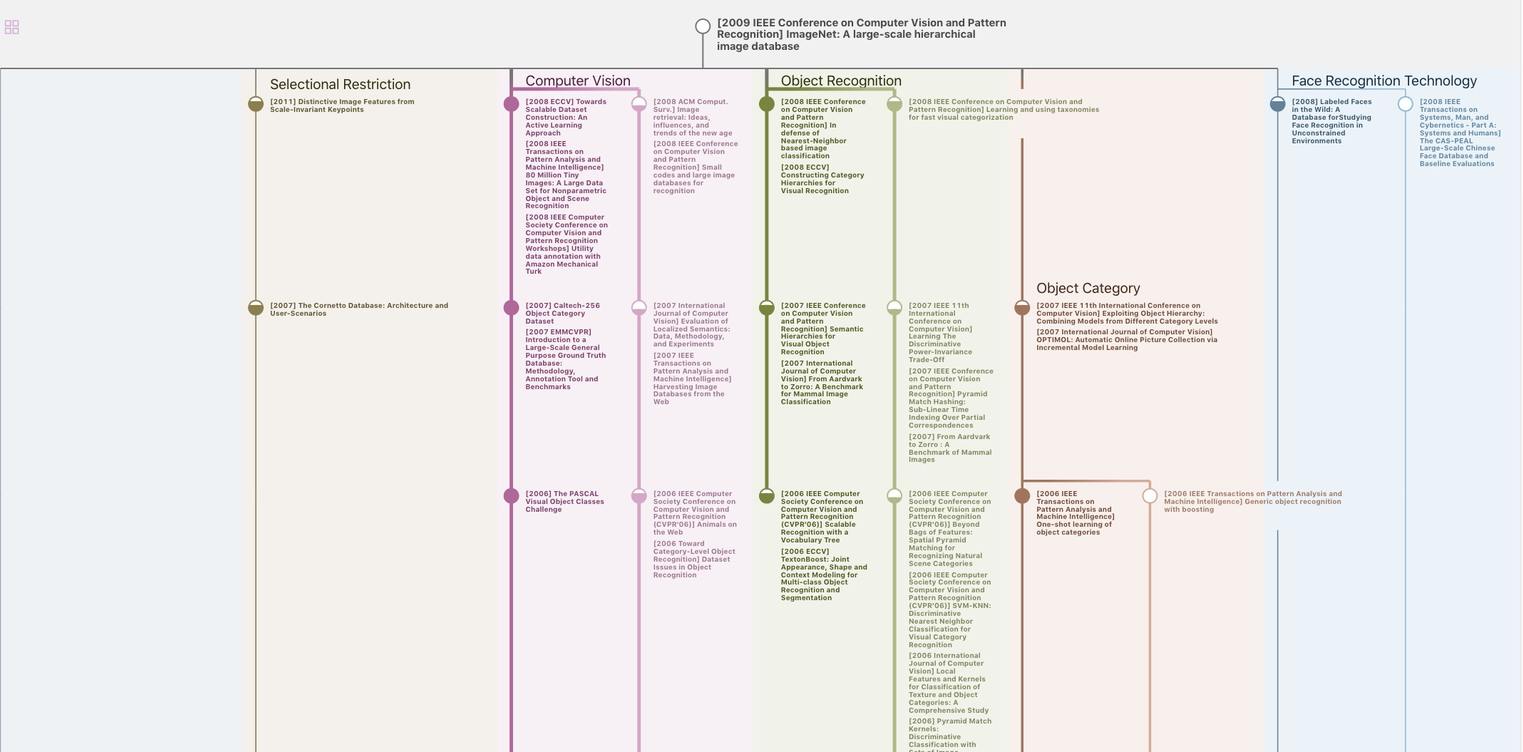
生成溯源树,研究论文发展脉络
Chat Paper
正在生成论文摘要