An Approximately-Zero-Gradient-Sum Algorithm For Consensus Optimization
2018 15TH INTERNATIONAL CONFERENCE ON CONTROL, AUTOMATION, ROBOTICS AND VISION (ICARCV)(2018)
摘要
This paper presents a set of distributed second-order methods for addressing unconstrained, smooth convex optimization over fixed networks. The proposed second-order methods, referred to as Approximately-Zero-Gradient-Sum (AZGS) algorithms, allow each node to update by combining the Hessian inverse of its local objective and the estimates of its neighbors, so that the gradient sum of the local objectives can be sufficiently close to zero at each iteration. We show that the AZGS algorithms, with properly selected parameters, enable all the nodes to asymptotically reach a consensus that can be arbitrarily close to the optimal solution. Finally, simulation results demonstrate the effectiveness of the AZGS algorithms.
更多查看译文
关键词
unconstrained convex optimization,smooth convex optimization,Hessian inverse,AZGS algorithms,optimal solution,consensus optimization,distributed second-order methods,approximately-zero-gradient-sum algorithm
AI 理解论文
溯源树
样例
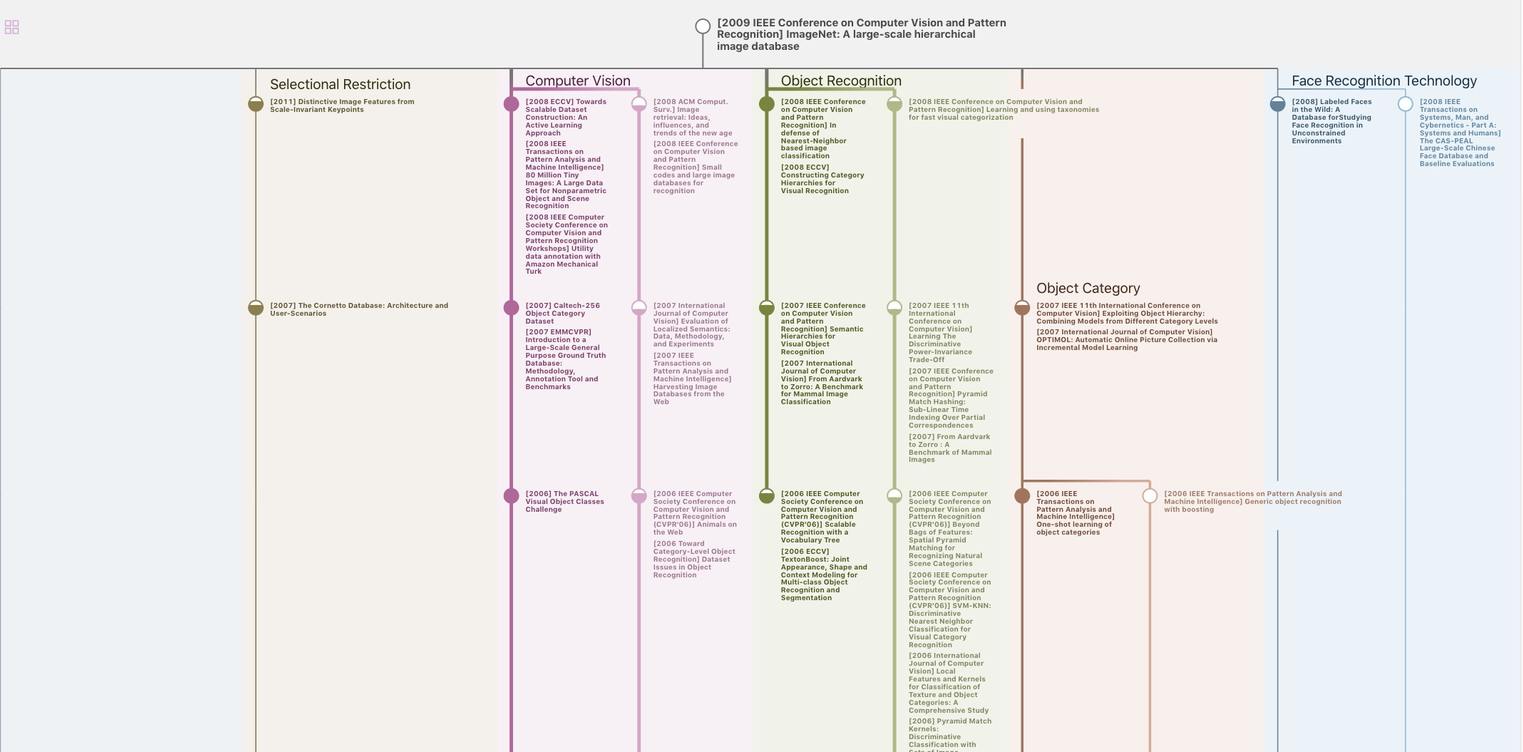
生成溯源树,研究论文发展脉络
Chat Paper
正在生成论文摘要