Optimized Vehicle Localization Based On Surveyed-Maps In Urban Environment
2018 15TH INTERNATIONAL CONFERENCE ON CONTROL, AUTOMATION, ROBOTICS AND VISION (ICARCV)(2018)
摘要
With the development of high reliability sensors, autonomous vehicles (AV) operating in real urban environments have been made possible. However, there still remain many challenges in improving the robustness and accuracy of AV localization in urban environments. In this paper, we propose a group of optimization techniques to make reliable map-based localization possible, using sparse point clouds from low cost light detection and ranging (LIDAR) sensors and odometry sensors. The map based Monte Carlo Localization (MCL) makes use of vertical and intensity features in it's observation model along with Heuristic Resampling to achieve robust localization. The proposed Heuristic Resampling picks the best candidate particle for localization output and resamples the remaining particles based on a defined heuristic. In addition, the LIDAR Calibration and Motion Compensation methods further improve localization accuracy. Experiments have been carried out to validate the effectiveness of the proposed techniques.
更多查看译文
关键词
sparse point clouds,low cost light detection,odometry sensors,robust localization,localization output,localization accuracy,surveyed-maps,urban environment,autonomous vehicles,AV localization,optimization techniques,vehicle localization,heuristic resampling,Monte Carlo localization,motion compensation methods,LIDAR calibration method
AI 理解论文
溯源树
样例
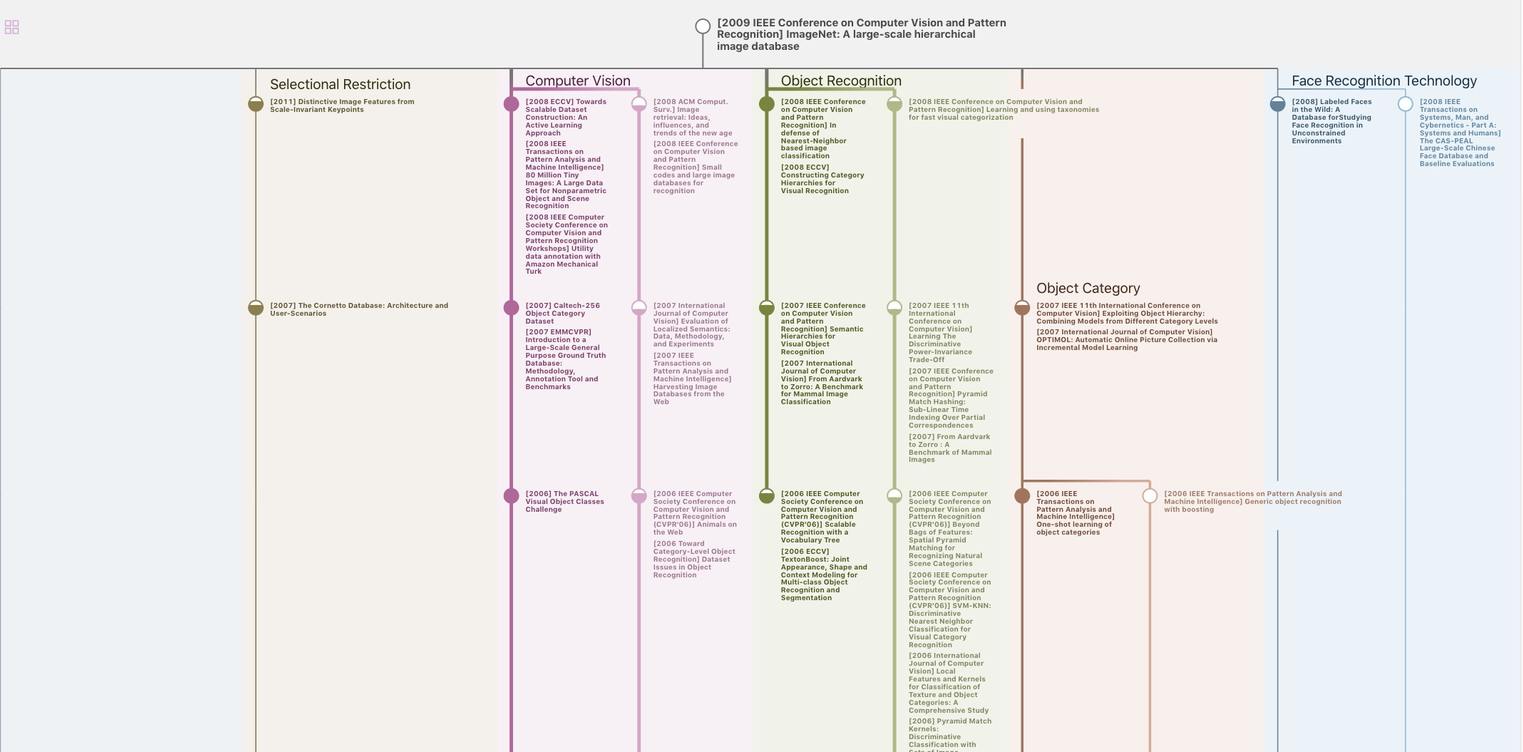
生成溯源树,研究论文发展脉络
Chat Paper
正在生成论文摘要