Simultaneous Perturbation Stochastic Approximation For Clustering Of A Gaussian Mixture Model Under Unknown But Bounded Disturbances
2017 IEEE CONFERENCE ON CONTROL TECHNOLOGY AND APPLICATIONS (CCTA 2017)(2017)
摘要
Multidimensional optimization holds a central role in many machine learning problems. When a model quality functional is measured with an almost arbitrary external noise, it makes sense to use randomized optimization techniques. This paper deals with the problem of clustering of a Gaussian mixture model under unknown but bounded disturbances. We introduce a stochastic approximation algorithm with randomly perturbed input (like SPSA) to solve this problem. The proposed method is appropriate for the online learning with streaming data, and it has a high speed of convergence. We study the conditions of the SPSA clustering algorithm applicability and show illustrative examples.
更多查看译文
关键词
Clustering, Gaussian mixture model, randomized algorithm, SPSA, unknown but bounded disturbances
AI 理解论文
溯源树
样例
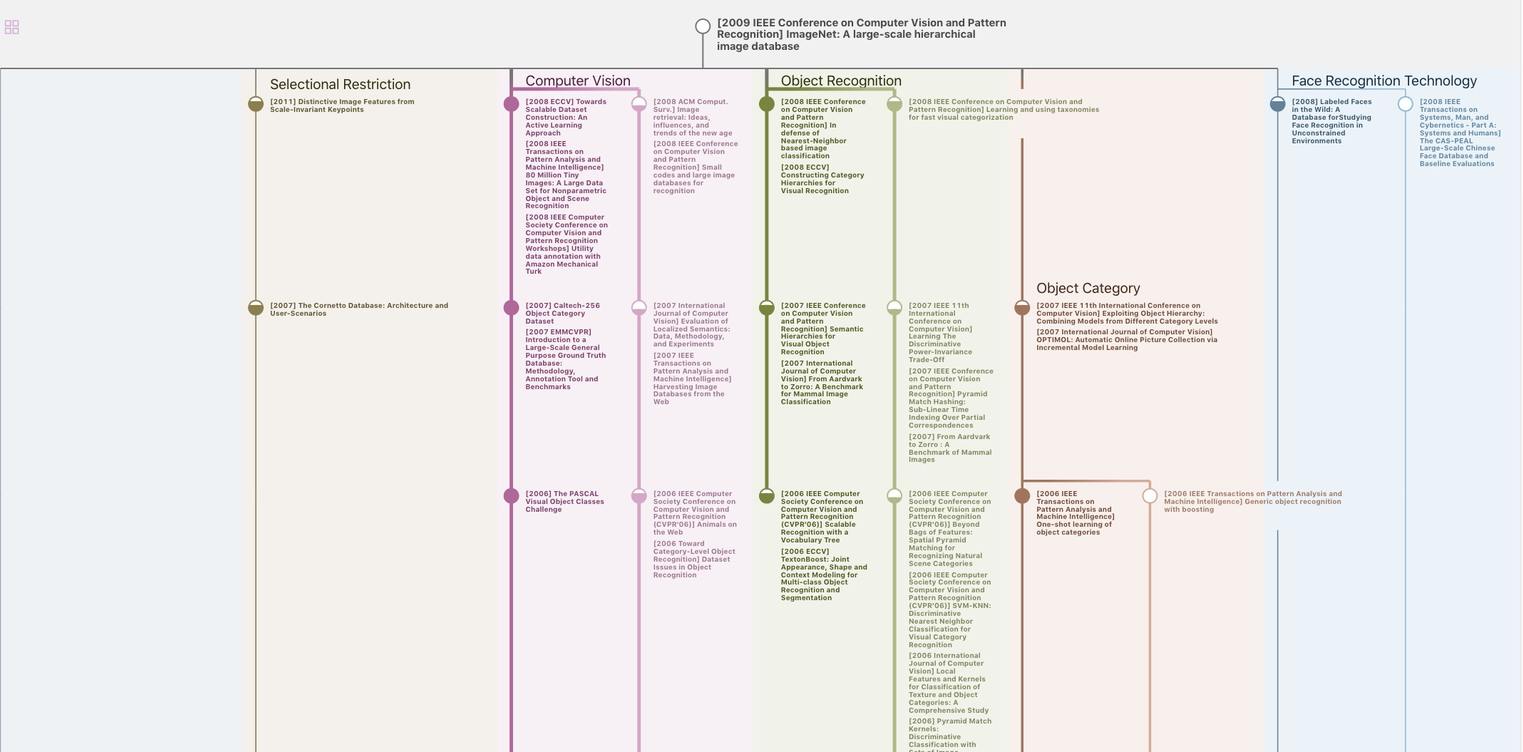
生成溯源树,研究论文发展脉络
Chat Paper
正在生成论文摘要