Mix'n'Squeeze: Thwarting Adaptive Adversarial Samples Using Randomized Squeezing.
arXiv: Cryptography and Security(2018)
摘要
Deep Learning (DL) has been shown to be particularly vulnerable to adversarial samples. To combat adversarial strategies, numerous defenses have been proposed in the literature. Among these, feature squeezing emerges as an effective defense by reducing unnecessary features without changing the DL model. However, feature squeezing is a static defense and does not resist adaptive attacks. Namely, feature squeezing is a deterministic process: as soon as an adversarial sample is found, this sample will always succeed against the classifier. In this work, we address this problem and introduce Mixu0027nu0027Squeeze, the first randomized feature squeezing defense that leverages key-based randomness and is secure against adaptive whitebox adversaries. Our defense consists of pre-processing the classifier inputs by embedding carefully selected randomness within each feature, before applying feature squeezing, so that an adaptive whitebox attacker can no longer predict the effect of their own perturbations on the resulting sample. We thoroughly implement and evaluate Mixu0027nu0027Squeeze in the context of image classification in light of the various reported strategies to generate adversarial samples. We also analyze the resilience of Mixu0027nu0027Squeeze with respect to state of the art adaptive strategies and we show that---in contrast to common belief---Mixu0027nu0027Squeeze does not hamper the classifieru0027s accuracy while significantly decreasing the success probability of an adaptive whitebox adversary.
更多查看译文
AI 理解论文
溯源树
样例
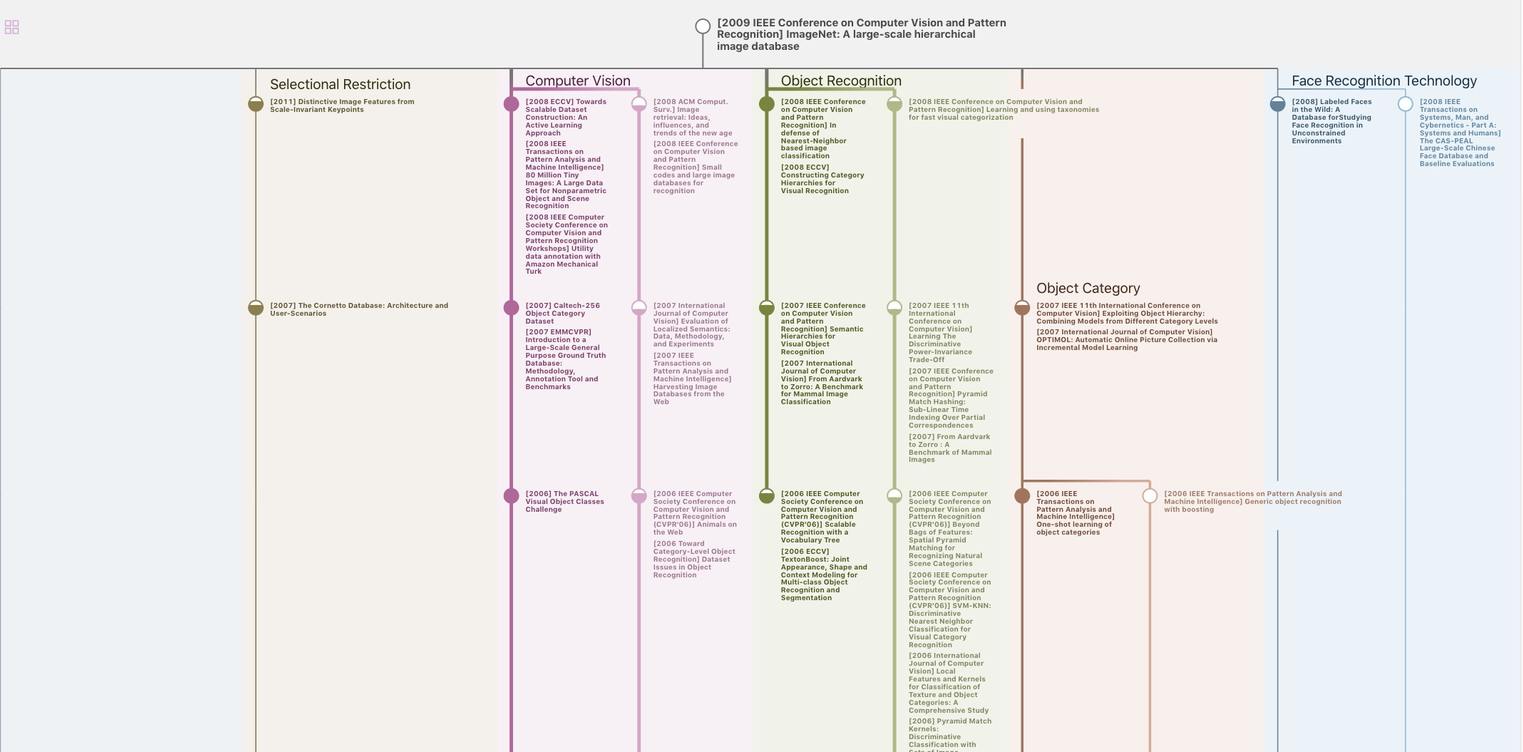
生成溯源树,研究论文发展脉络
Chat Paper
正在生成论文摘要