Deep Positron: A Deep Neural Network Using the Posit Number System
2019 DESIGN, AUTOMATION & TEST IN EUROPE CONFERENCE & EXHIBITION (DATE)(2019)
Abstract
The recent surge of interest in Deep Neural Networks (DNNs) has led to increasingly complex networks that tax computational and memory resources. Many DNNs presently use 16-bit or 32-bit floating point operations. Significant performance and power gains can be obtained when DNN accelerators support low-precision numerical formats. Despite considerable research, there is still a knowledge gap on how low-precision operations can be realized for both DNN training and inference. In this work, we propose a DNN architecture, Deep Positron, with posit numerical format operating successfully at ≤8 bits for inference. We propose a precision-adaptable FPGA soft core for exact multiply-and-accumulate for uniform comparison across three numerical formats, fixed, floating-point and posit. Preliminary results demonstrate that 8-bit posit has better accuracy than 8-bit fixed or floating-point for three different low-dimensional datasets. Moreover, the accuracy is comparable to 32-bit floating-point on a Xilinx Virtex-7 FPGA device. The trade-offs between DNN performance and hardware resources, i.e. latency, power, and resource utilization, show that posit outperforms in accuracy and latency at 8-bit and below.
MoreTranslated text
Key words
deep neural networks, machine learning, DNN accelerators, posits, floating point, tapered precision, low-precision
AI Read Science
Must-Reading Tree
Example
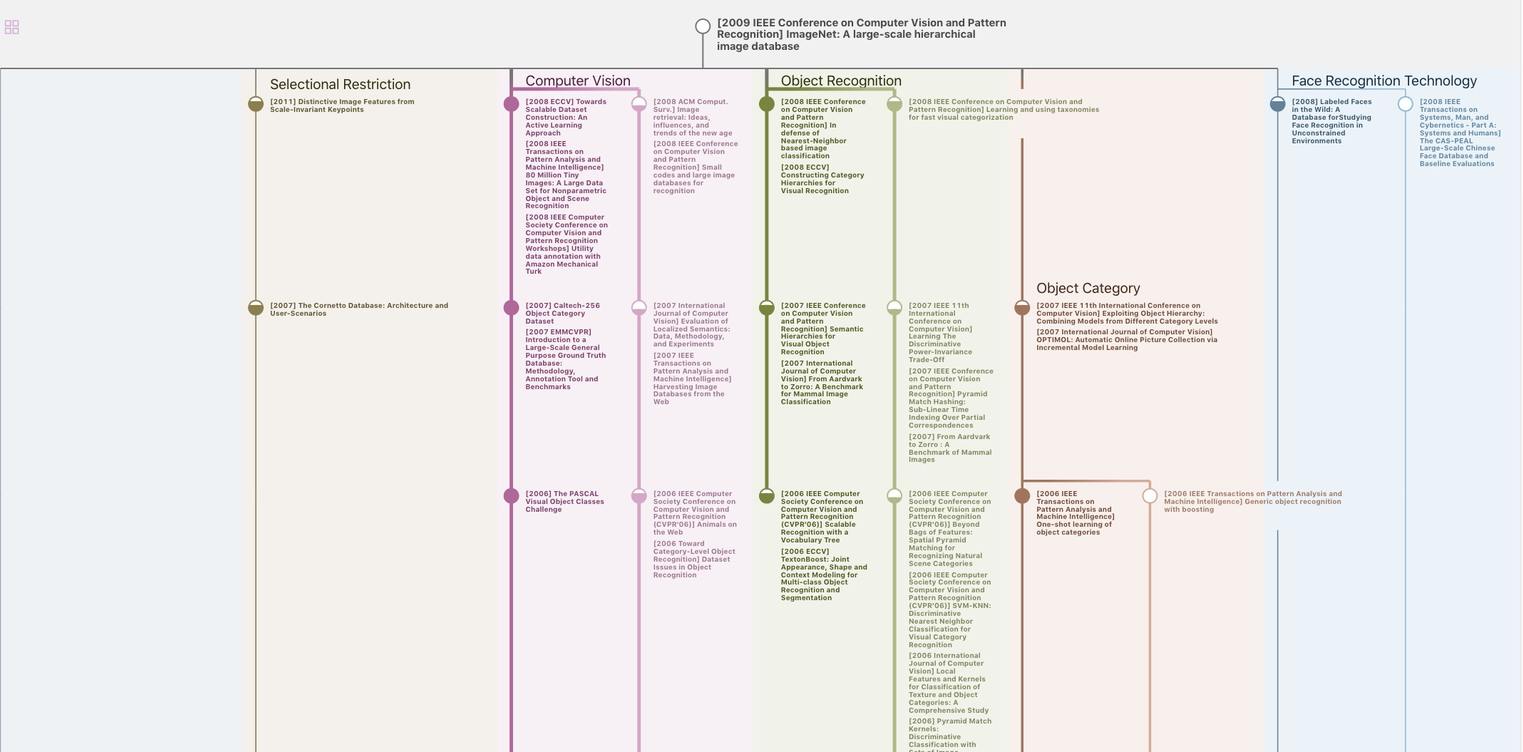
Generate MRT to find the research sequence of this paper
Chat Paper
Summary is being generated by the instructions you defined