ADVENT: Adversarial Entropy Minimization for Domain Adaptation in Semantic Segmentation
2019 IEEE/CVF Conference on Computer Vision and Pattern Recognition (CVPR)(2019)
Abstract
Semantic segmentation is a key problem for many computer vision tasks. While approaches based on convolutional neural networks constantly break new records on different benchmarks, generalizing well to diverse testing environments remains a major challenge. In numerous real world applications, there is indeed a large gap between data distributions in train and test domains, which results in severe performance loss at run-time. In this work, we address the task of unsupervised domain adaptation in semantic segmentation with losses based on the entropy of the pixel-wise predictions. To this end, we propose two novel, complementary methods using (i) entropy loss and (ii) adversarial loss respectively. We demonstrate state-of-the-art performance in semantic segmentation on two challenging "synthetic-2-real" set-ups and show that the approach can also be used for detection.
MoreTranslated text
Key words
Scene Analysis and Understanding,Deep Learning,Recognition: Detection,Categorization,Retrieval,Segmentation,Grouping and Shape
AI Read Science
Must-Reading Tree
Example
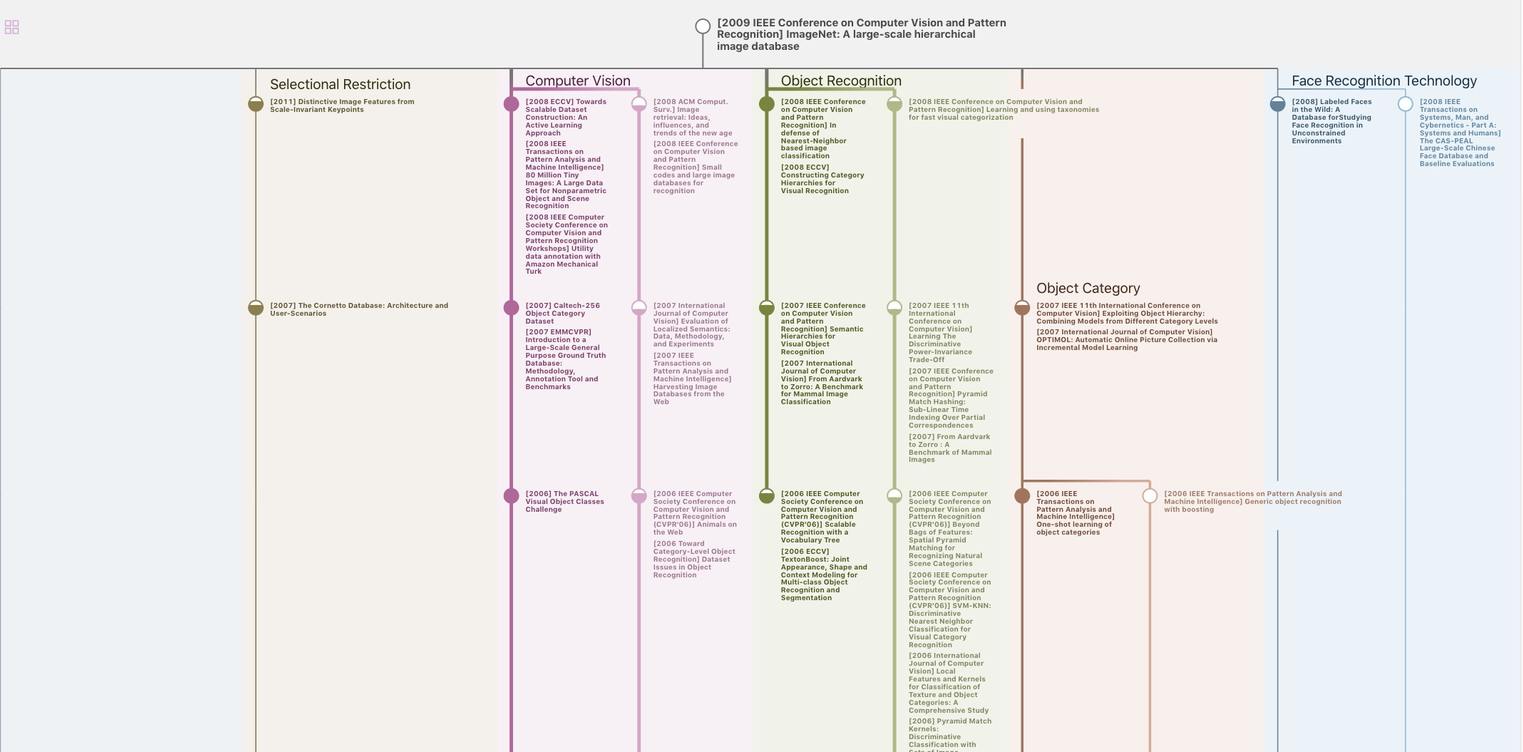
Generate MRT to find the research sequence of this paper
Chat Paper
Summary is being generated by the instructions you defined