Learning Controllable Fair Representations
22ND INTERNATIONAL CONFERENCE ON ARTIFICIAL INTELLIGENCE AND STATISTICS, VOL 89(2019)
Abstract
Learning data representations that are transferable and are fair with respect to certain protected attributes is crucial to reducing unfair decisions while preserving the utility of the data. We propose an information-theoretically motivated objective for learning maximally expressive representations subject to fairness constraints. We demonstrate that a range of existing approaches optimize approximations to the Lagrangian dual of our objective. In contrast to these existing approaches, our objective allows the user to control the fairness of the representations by specifying limits on unfairness. Exploiting duality, we introduce a method that optimizes the model parameters as well as the expressiveness-fairness trade-off. Empirical evidence suggests that our proposed method can balance the trade-off between multiple notions of fairness and achieves higher expressiveness at a lower computational cost.
MoreTranslated text
Key words
representations,learning
AI Read Science
Must-Reading Tree
Example
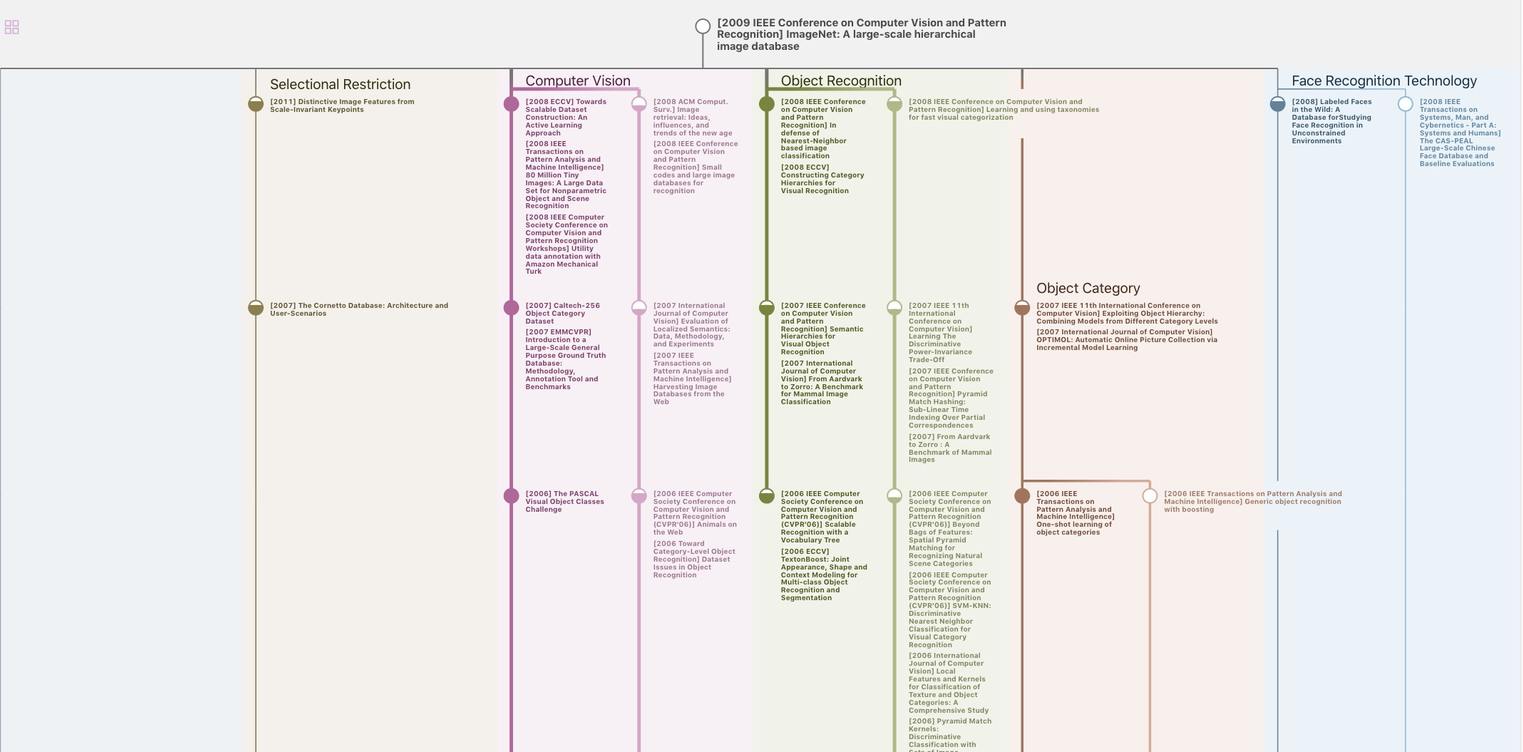
Generate MRT to find the research sequence of this paper
Chat Paper
Summary is being generated by the instructions you defined