Semantic Map Annotation Through UAV Video Analysis Using Deep Learning Models in ROS.
MMM(2019)
摘要
Enriching the map of the flight environment with semantic knowledge is a common need for several UAV applications. Safety legislations require no-fly zones near crowded areas that can be indicated by semantic annotations on a geometric map. This work proposes an automatic annotation of 3D maps with crowded areas, by projecting 2D annotations that are derived through visual analysis of UAV video frames. To this aim, a fully convolutional neural network is proposed, in order to comply with the computational restrictions of the application, that can effectively distinguish between crowded and non-crowded scenes based on a regularized multiple-loss training method, and provide semantic heatmaps that are projected on the 3D occupancy grid of Octomap. The projection is based on raycasting and leads to polygonal areas that are geo-localized on the map and could be exported in KML format. Initial qualitative evaluation using both synthetic and real world drone scenes, proves the applicability of the method.
更多查看译文
关键词
Drone imaging, Crowd detection, Deep learning, FCNN, Semantic mapping, Octomap, ROS
AI 理解论文
溯源树
样例
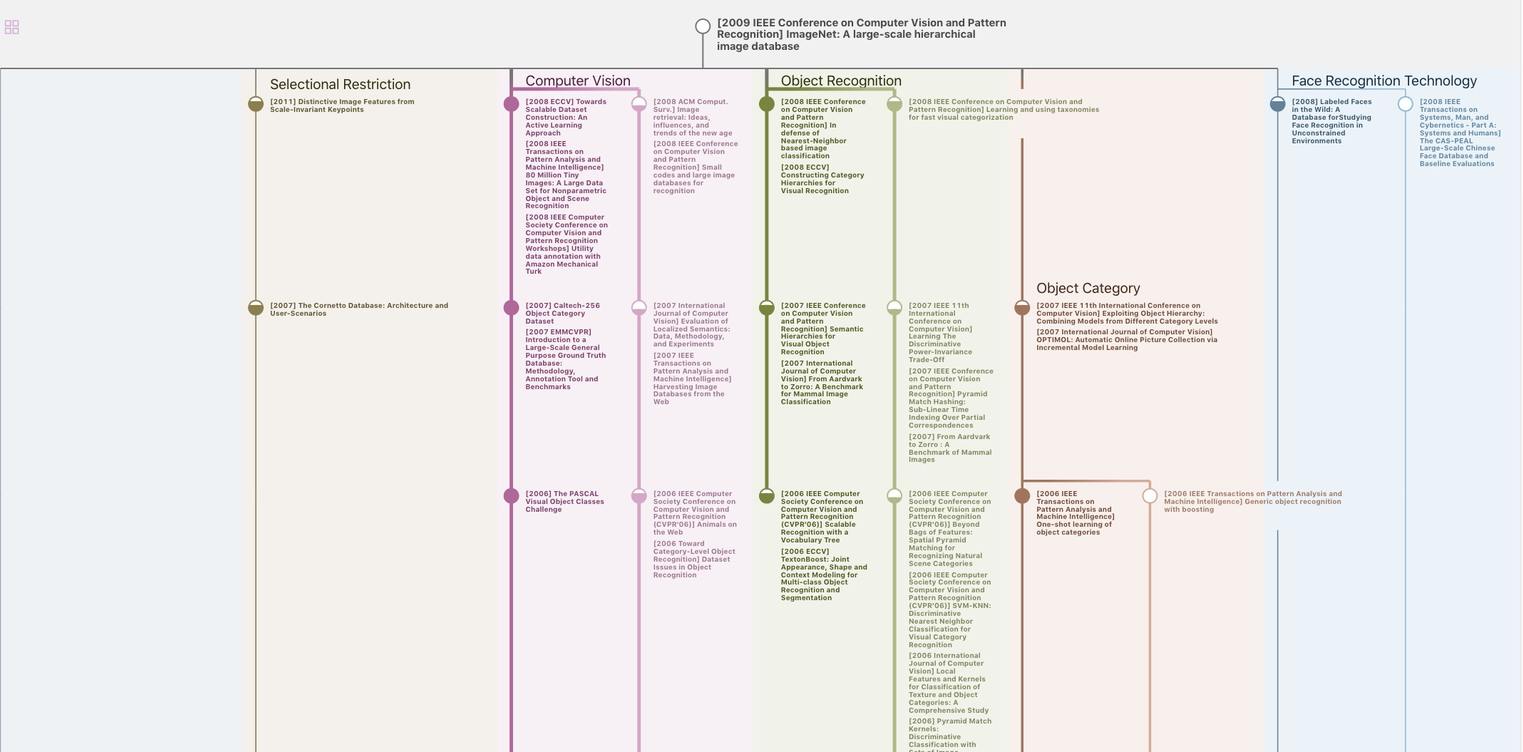
生成溯源树,研究论文发展脉络
Chat Paper
正在生成论文摘要