Robot Navigation on Slow Feature Gradients
NEURAL INFORMATION PROCESSING (ICONIP 2018), PT VII(2018)
摘要
Unsupervised learning with Slow Feature Analysis (SFA) enables an agent to learn spatial representations of its environment from images captured during an exploration phase. In a subsequent application phase, slow features encode the robot’s position. The representation is spatially smooth and implicitly encodes the average travel time during exploration. Following the SFA gradient allows the robot to navigate even around obstacles without any planning. Earlier work showed this basic principle in noise-free simulation, using two virtual cameras on a robot. We extend the approach to be more robust and more computationally efficient. We test it on a lawn mower robot with a single camera for navigation in free space and avoiding obstacles.
更多查看译文
AI 理解论文
溯源树
样例
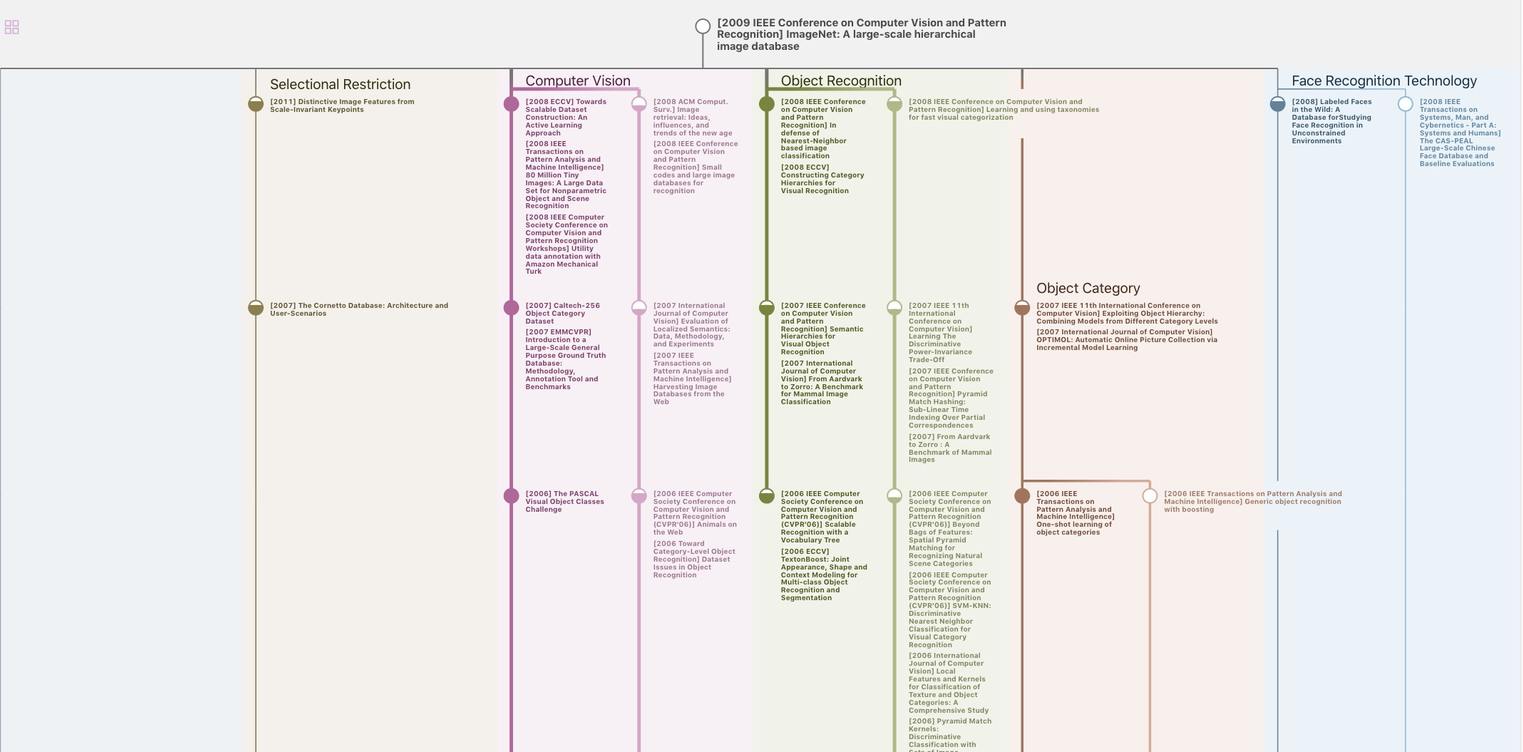
生成溯源树,研究论文发展脉络
Chat Paper
正在生成论文摘要