Re-Cnn: A Robust Convolutional Neural Networks For Image Recognition
NEURAL INFORMATION PROCESSING (ICONIP 2018), PT I(2018)
摘要
Recent years we have witnessed revolutionary changes, essentially caused by deep learning and Convolutional Neural Networks (CNN). The performance of image recognition by convolutional neural networks has been substantially boosted. Despite the greater success, the selection of the convolution kernel and the strategy of the pooling layer that only consider the local region and ignore the global region remain several major challenges. These problems may lead to a high correlation between the extracted features and the appearance of the over-fitting. To address the problem, in this paper, a novel and robust method to learn a removal correlation CNN (RE-CNN) model is proposed. This model is achieved by introducing and learning removal correlation layers on the basis of the existing high-capacity CNN architectures. Specifically, the removal correlation layer is trained by the reconstructed CNN features (in this paper, the CNN features are outputs of the layer before classifier layer) using canonical correlation analysis (CCA). The original CNN features are projected into a subspace where the reconstructed CNN features are not correlated. Our extensive experiments on MNIST and LFW datasets demonstrate that the proposed RE-CNN model can improve the recognition capabilities of many existing high-capacity CNN architectures.
更多查看译文
关键词
Image recognition, Convolutional neural networks, Removal correlation, Canonical correlation analysis
AI 理解论文
溯源树
样例
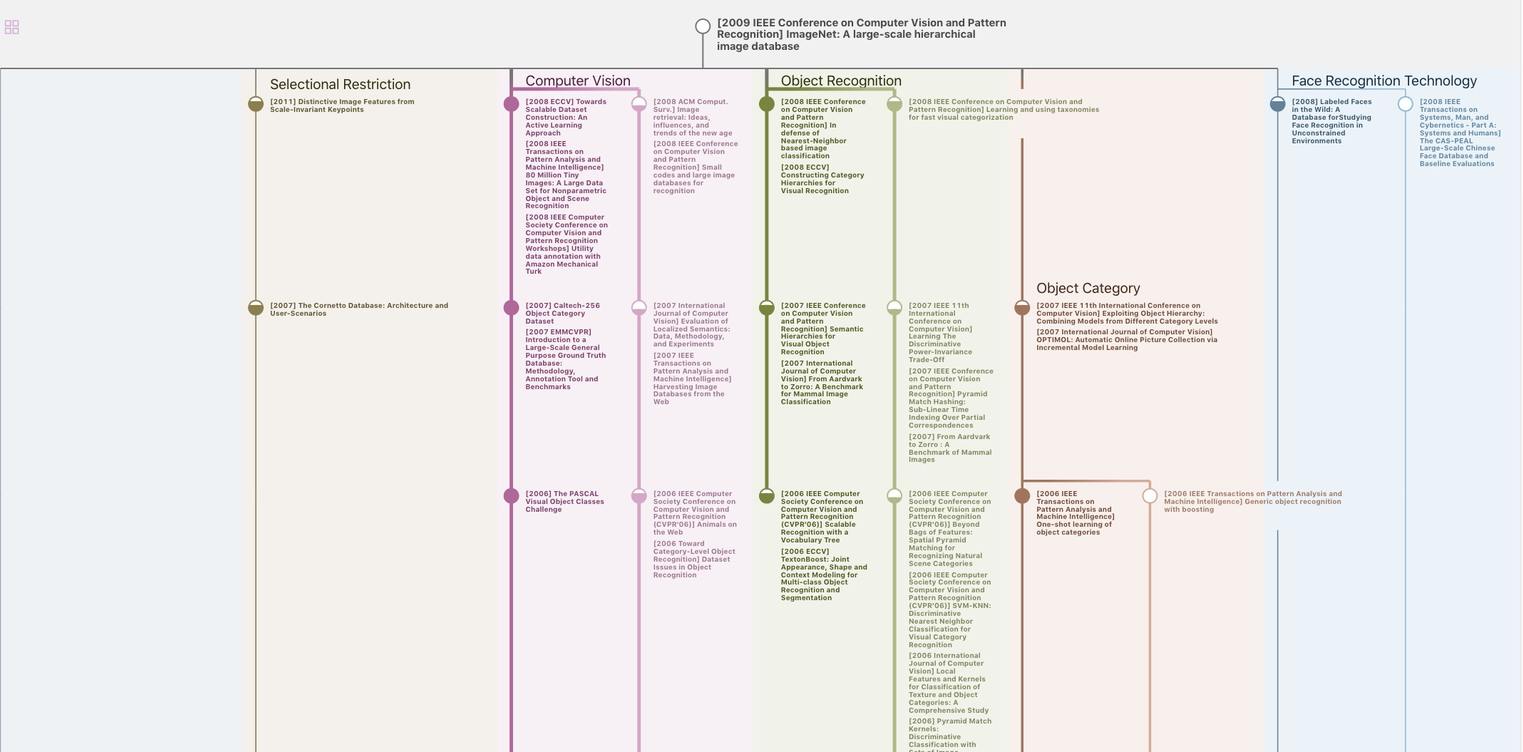
生成溯源树,研究论文发展脉络
Chat Paper
正在生成论文摘要