Region And Temporal Dependency Fusion For Multi-Label Action Unit Detection
2018 24TH INTERNATIONAL CONFERENCE ON PATTERN RECOGNITION (ICPR)(2018)
摘要
Automatic Facial Action Unit (AU) detection from videos increases numerous interests over the past years due to its importance for analyzing facial expressions. Many proposed methods face challenges in detecting sparse face regions for different AUs, in the fusion of temporal dependency, and in learning multiple AUs simultaneously. In this paper, we propose a novel deep neural network architecture for AU detection to model above-mentioned challenges jointly. Firstly, to capture the region sparsity, we design a region pooling layer after a fully convolutional network to extract per-region features for each AU. Secondly, in order to integrate temporal dependency, Long Short Term Memory (LSTM) is stacked on the top of regional features. Finally, the regional features and outputs of LSTMs are utilized together to produce per-frame multi-label predictions. Experimental results on three large spontaneous AU datasets, BP4D, GFT and DISFA, have demonstrated our work outperforms state-of-the-art methods. On three datasets, our work has highest average F1 and AUC scores with an average F1 score improvement of 4.8% on BP4D, 12.7% on GFT and 14.3% on DISFA, and an average AUC score improvement of 27.4% on BP4D and 33.5% on DISFA.
更多查看译文
关键词
temporal dependency,multilabel action unit detection,videos increases numerous interests,facial expressions,sparse face regions,deep neural network architecture,AU detection,region sparsity,region pooling layer,fully convolutional network,per-region features,regional features,per-frame multilabel predictions,spontaneous AU datasets,state-of-the-art methods,BP4D,long short term memory,multiple AU,automatic facial action unit detection
AI 理解论文
溯源树
样例
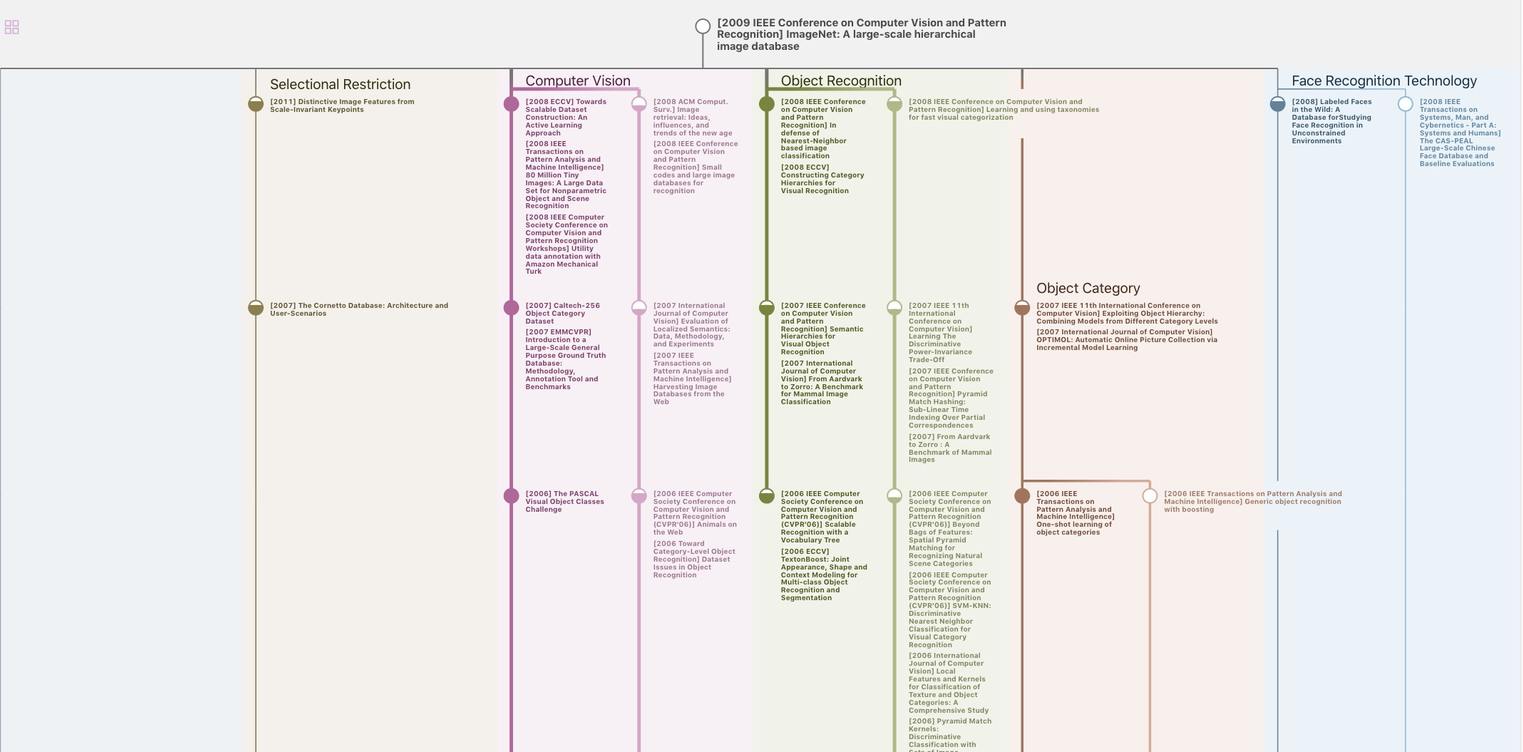
生成溯源树,研究论文发展脉络
Chat Paper
正在生成论文摘要