Data Augmentation Via Latent Space Interpolation For Image Classification
2018 24TH INTERNATIONAL CONFERENCE ON PATTERN RECOGNITION (ICPR)(2018)
摘要
Effective training of the deep neural networks requires much data to avoid underdetermined and poor generalization. Data Augmentation alleviates this by using existing data more effectively. However standard data augmentation produces only limited plausible alternative data by for example, flipping, distorting, adding noise to, cropping a patch from the original samples. In this paper, we introduce the adversarial autoencoder (AAE) to impose the feature representations with uniform distribution and apply the linear interpolation on latent space, which is potential to generate a much broader set of augmentations for image classification. As a possible "recognition via generation" framework, it has potentials for several other classification tasks. Our experiments on the ILSVRC 2012, CIFAR-10 datasets show that the latent space interpolation (LSI) improves the generalization and performance of state-of-the-art deep neural networks.
更多查看译文
关键词
classification, data augmentation, vicinal risk minimization, inter-class sampling
AI 理解论文
溯源树
样例
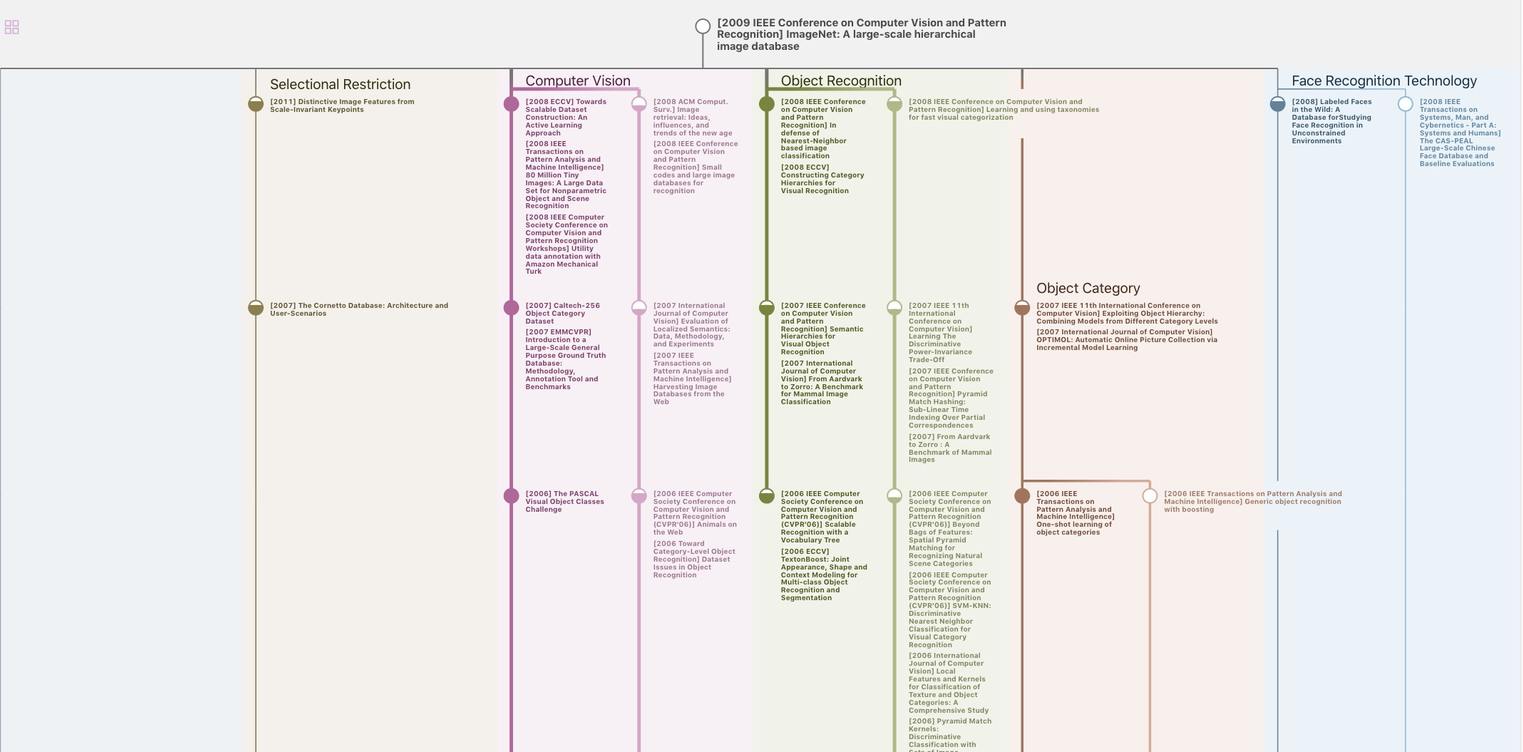
生成溯源树,研究论文发展脉络
Chat Paper
正在生成论文摘要