Cayley- Klein Metric Learning with Shrinkage-Expansion Constraints
2018 24th International Conference on Pattern Recognition (ICPR)(2018)
摘要
Cayley-Klein metric is a specific kind of non-Euclidean metric in projective space. Recently, it has been introduced into metric learning with encouraging performance when dealing with computer vision tasks. However, the original Cayley-Klein metric learning methods with conventional pairwise and triplet-wise constraints, which are fixed bound based constraints, may not perform well when the intra-and inter-class variations of data distribution become complex. Pairwise constraints restrict the distance between samples of a similar pair to be lower than a fixed upper bound, and the distance between samples of a dissimilar pair higher than a fixed lower bound. Triplet-wise constraints restrict the distance between samples of a similar pair to be smaller than that between a pair of samples from different classes. In this paper, we propose a novel Cayley-Klein metric learning method (CKseML) with adaptive shrinkage-expansion pairwise constraints. CKseML is very effective in learning metric from data with complex distributions. Our experimental results demonstrate that CKseML achieves better performance than the original Cayley-Klein metric learning methods.
更多查看译文
关键词
triplet-wise constraints,adaptive shrinkage-expansion pairwise constraints,original Cayley-Klein metric learning methods,shrinkage-expansion constraints,CKseML
AI 理解论文
溯源树
样例
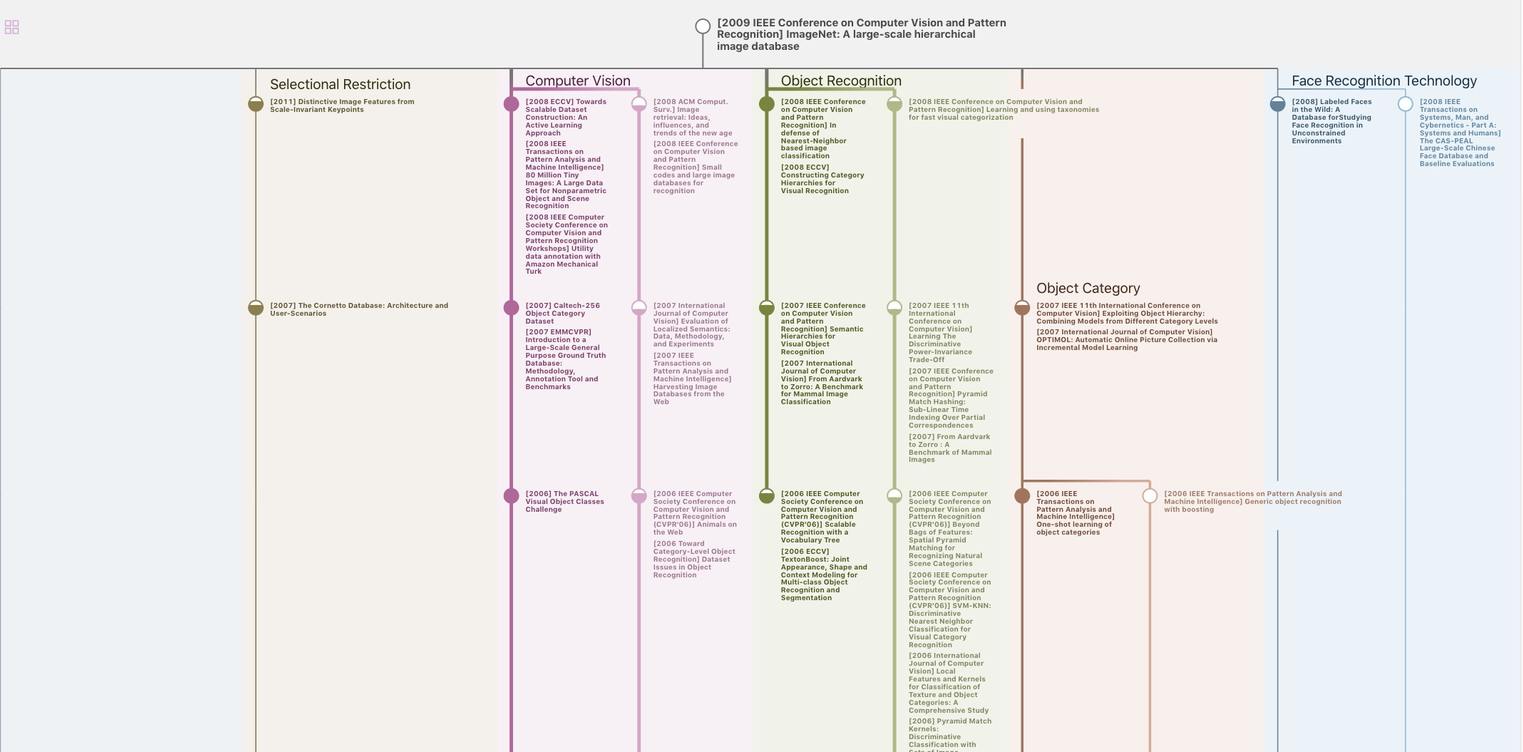
生成溯源树,研究论文发展脉络
Chat Paper
正在生成论文摘要