Pomdp And Hierarchical Options Mdp With Continuous Actions For Autonomous Driving At Intersections
2018 21ST INTERNATIONAL CONFERENCE ON INTELLIGENT TRANSPORTATION SYSTEMS (ITSC)(2018)
摘要
When applying autonomous driving technology to real-world scenarios, environmental uncertainties make the development of decision-making algorithms difficult. Modeling the problem as a Partially Observable Markov Decision Process (POMDP) [1] allows the algorithm to consider these uncertainties in the decision process, which makes it more robust to real sensor characteristics. However, solving the POMDP with reinforcement learning (RL) [2] often requires storing a large number of observations. Furthermore, for continuous action spaces, the system is computationally inefficient. This paper addresses these problems by proposing to model the problem as an MDP and learn a policy with RL using hierarchical options (HOMDP). The suggested algorithm can store the state-action pairs and only uses current observations to solve a POMDP problem. We compare the results of to the time-to-collision method [3] and the proposed POMDP-with-LSTM method. Our results show that the HOMDP approach is able to improve the performance of the agent for a four-way intersection task with two-way stop signs. The HOMDP method can generate both higher-level discrete options and lower-level continuous actions with only the observations of the current step.
更多查看译文
关键词
hierarchical options MDP,intersections,Partially Observable Markov Decision Process,reinforcement learning,HOMDP method,continuous actions,autonomous driving technology,decision-making algorithms,POMDP
AI 理解论文
溯源树
样例
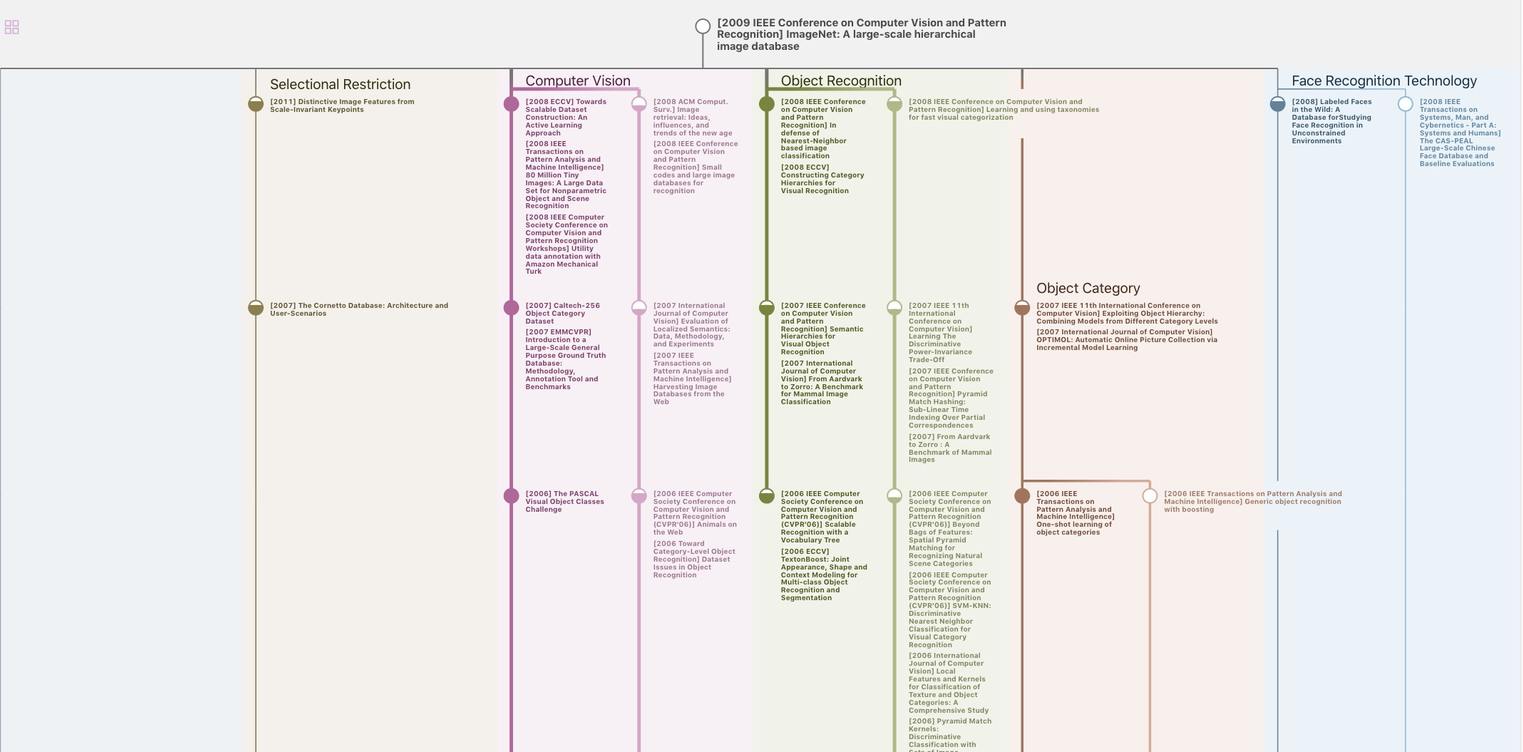
生成溯源树,研究论文发展脉络
Chat Paper
正在生成论文摘要