Snm: Stochastic Newton Methodfor Optimization Of Discrete Choice Models
2018 21ST INTERNATIONAL CONFERENCE ON INTELLIGENT TRANSPORTATION SYSTEMS (ITSC)(2018)
Abstract
Optimization algorithms are rarely mentioned in the discrete choice literature. One reason may be that classic Newton-Raphson methods have been rather successful in estimating discrete choice parameters on available data sets of limited size. However, thanks to recent advances in data collection, abundant data about choice situations become more and more available, and state-of-the-art algorithms can be computationally burdensome on these massive datasets. In this paper, inspired by the good practices from the machine learning field, we introduce a Stochastic Newton Method (SNM) for the estimation of discrete choice models parameters. Our preliminary results indicate that our method outperforms (stochastic) first-order and quasi-newton methods.
MoreTranslated text
Key words
Discrete Choice Models, Optimization
AI Read Science
Must-Reading Tree
Example
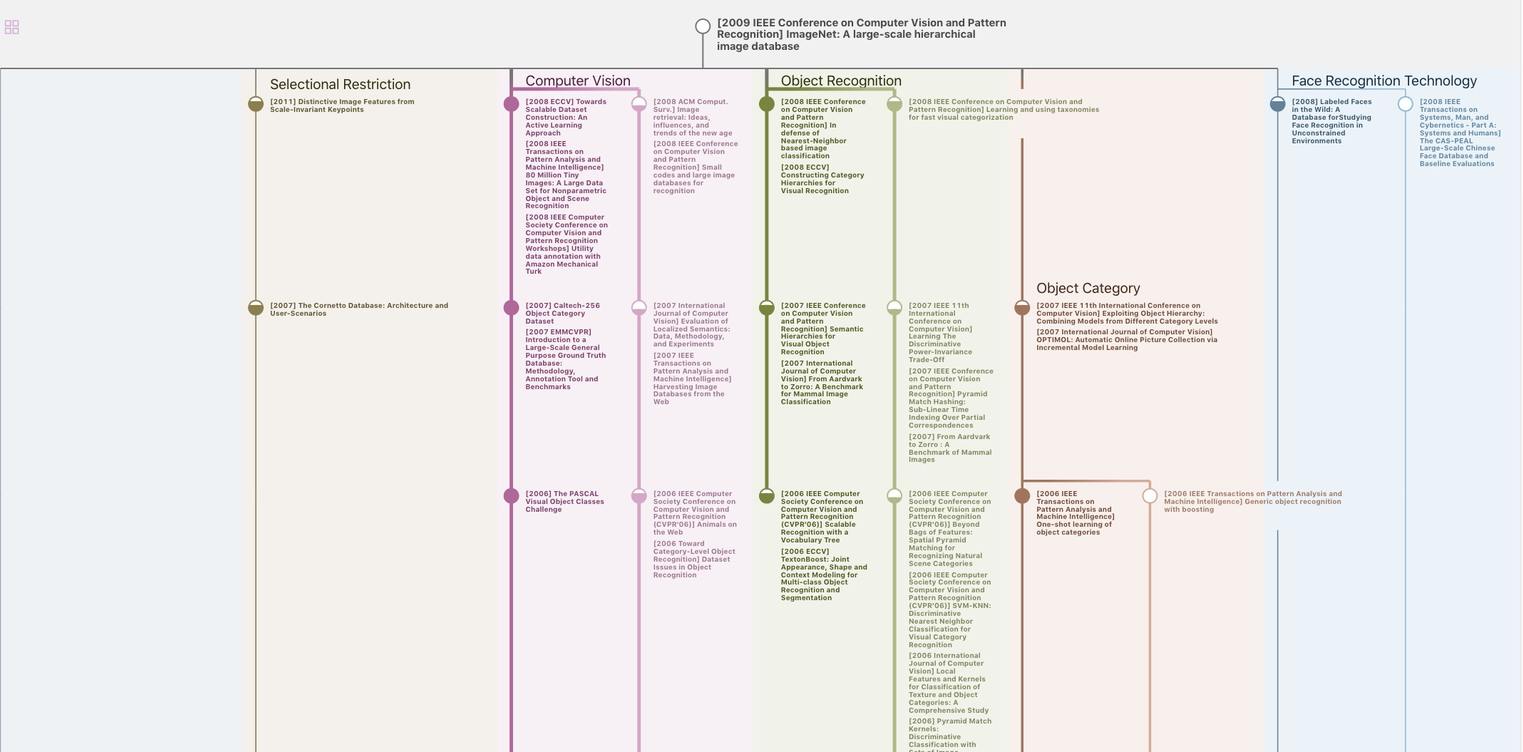
Generate MRT to find the research sequence of this paper
Chat Paper
Summary is being generated by the instructions you defined