Vehicle Detection and Localization using 3D LIDAR Point Cloud and Image Semantic Segmentation
2018 21ST INTERNATIONAL CONFERENCE ON INTELLIGENT TRANSPORTATION SYSTEMS (ITSC)(2018)
摘要
This paper presents a real-time approach to detect and localize surrounding vehicles in urban driving scenes. We propose a multimodal fusion framework that processes both 3D LIDAR point cloud and RGB image to obtain robust vehicle position and size in a Bird's Eye View (BEV). Semantic segmentation from RGB images is obtained using our efficient Convolutional Neural Network (CNN) architecture called ERFNet. Our proposal takes advantage of accurate depth information provided by LIDAR and detailed semantic information processed from a camera. The method has been tested using the KITTI object detection benchmark. Experiments show that our approach outperforms or is on par with other state-of-the-art proposals but our CNN was trained in another dataset, showing a good generalization capability to any domain, a key point for autonomous driving.
更多查看译文
关键词
KITTI object detection benchmark,CNN,autonomous driving,vehicle detection,image semantic segmentation,urban driving scenes,multimodal fusion framework,RGB image,robust vehicle position,Bird's Eye View,3D LIDAR point cloud,convolutional neural network,ERFNet,BEV
AI 理解论文
溯源树
样例
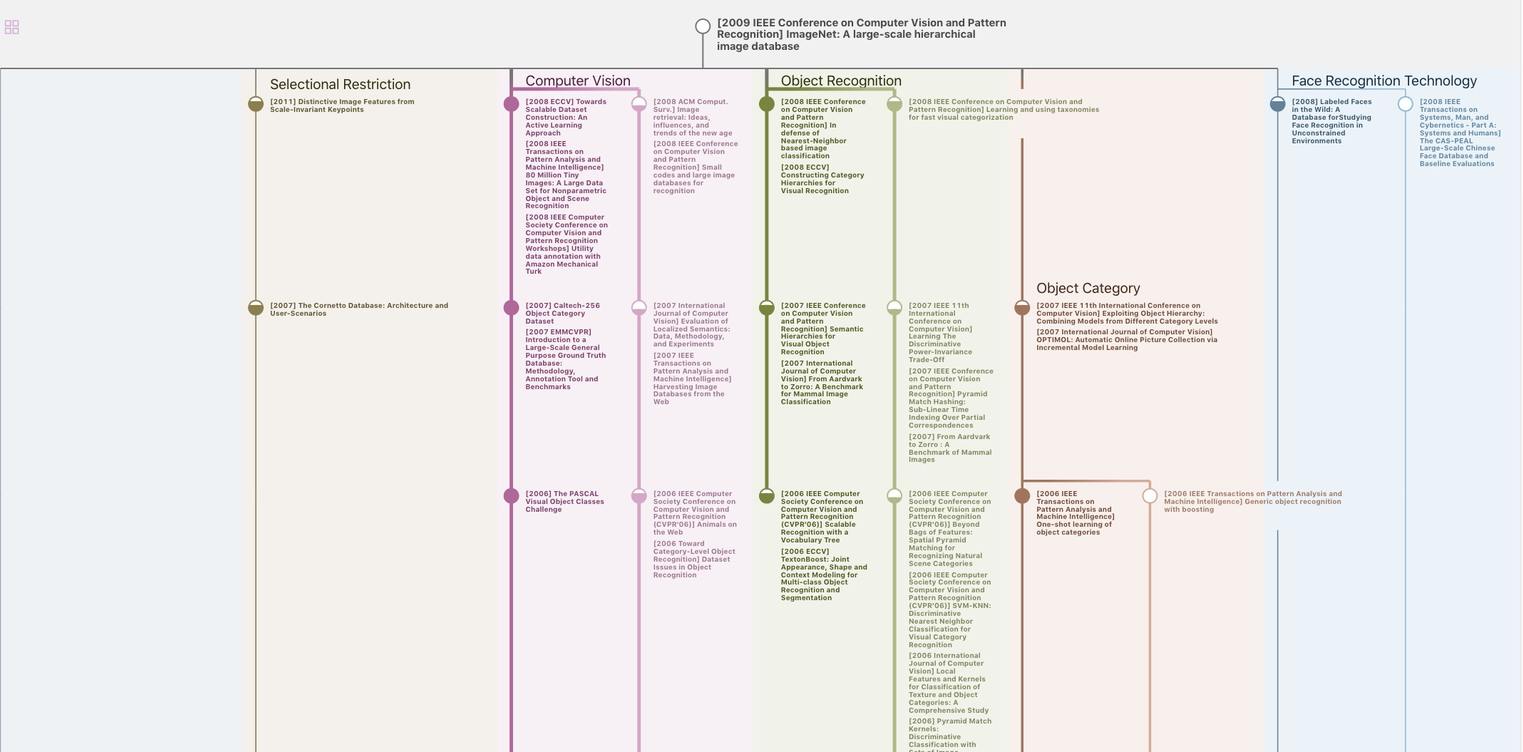
生成溯源树,研究论文发展脉络
Chat Paper
正在生成论文摘要