Potent pairing: ensemble of long short-term memory networks and support vector machine for chemical-protein relation extraction.
DATABASE-THE JOURNAL OF BIOLOGICAL DATABASES AND CURATION(2018)
摘要
Biomedical researchers regularly discover new interactions between chemical compounds/ drugs and genes/ proteins, and report them in research literature. Having knowledge about these interactions is crucially important in many research areas such as precision medicine and drug discovery. The BioCreative VI Task 5 (CHEMPROT) challenge promotes the development and evaluation of computer systems that can automatically recognize and extract statements of such interactions from biomedical literature. We participated in this challenge with a Support VectorMachine (SVM) system and a deep learning-based system (ST-ANN), and achieved an F-score of 60.99 for the task. After the shared task, we have significantly improved the performance of the ST-ANN system. Additionally, we have developed a new deep learning-based system (I-ANN) that considerably outperforms the ST-ANN system. Both ST-ANN and I-ANN systems are centered around training an ensemble of artificial neural networks and utilizing different bidirectional Long Short-Term Memory (LSTM) chains for representing the shortest dependency path and/ or the full sentence. By combining the predictions of the SVM and the I-ANN systems, we achieved an F-score of 63.10 for the task, improving our previous F-score by 2.11 percentage points. Our systems are fully open-source and publicly available. We highlight that the systems we present in this study are not applicable only to the BioCreative VI Task 5, but can be effortlessly re-trained to extract any types of relations of interest, with no modifications of the source code required, if a manually annotated corpus is provided as training data in a specific file format.
更多查看译文
AI 理解论文
溯源树
样例
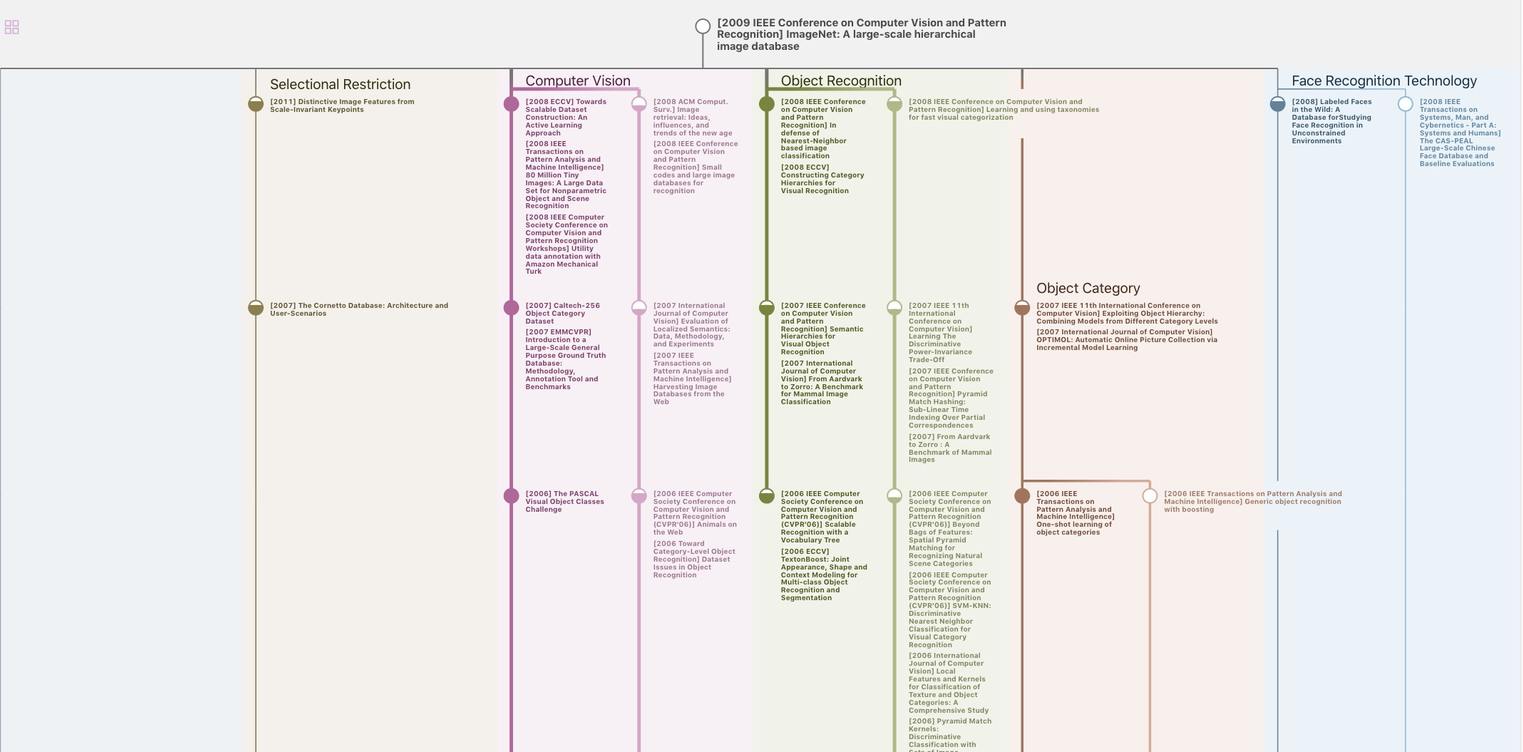
生成溯源树,研究论文发展脉络
Chat Paper
正在生成论文摘要