Design and analysis considerations for combining data from multiple biomarker studies.
STATISTICS IN MEDICINE(2019)
摘要
Pooling data from multiple studies improves estimation of exposure-disease associations through increased sample size. However, biomarker exposure measurements can vary substantially across laboratories and often require calibration to a reference assay prior to pooling. We develop two statistical methods for aggregating biomarker data from multiple studies: the full calibration method and the internalized method. The full calibration method calibrates all biomarker measurements regardless of the availability of reference laboratory measurements while the internalized method calibrates only non-reference laboratory measurements. We compare the performance of these two aggregation methods to two-stage methods. Furthermore, we compare the aggregated and two-stage methods when estimating the calibration curve from controls only or from a random sample of individuals from the study cohort. Our findings include the following: (1) Under random sampling for calibration, exposure effect estimates from the internalized method have a smaller mean squared error than those from the full calibration method. (2) Under the controls-only calibration design, the full calibration method yields effect estimates with the least bias. (3) The two-stage approaches produce average effect estimates that are similar to the full calibration method under a controls only calibration design and the internalized method under a random sample calibration design. We illustrate the methods in an application evaluating the relationship between circulating vitamin D levels and stroke risk in a pooling project of cohort studies.
更多查看译文
关键词
aggregation,between-study variability,calibration,pooling project,two-stage method
AI 理解论文
溯源树
样例
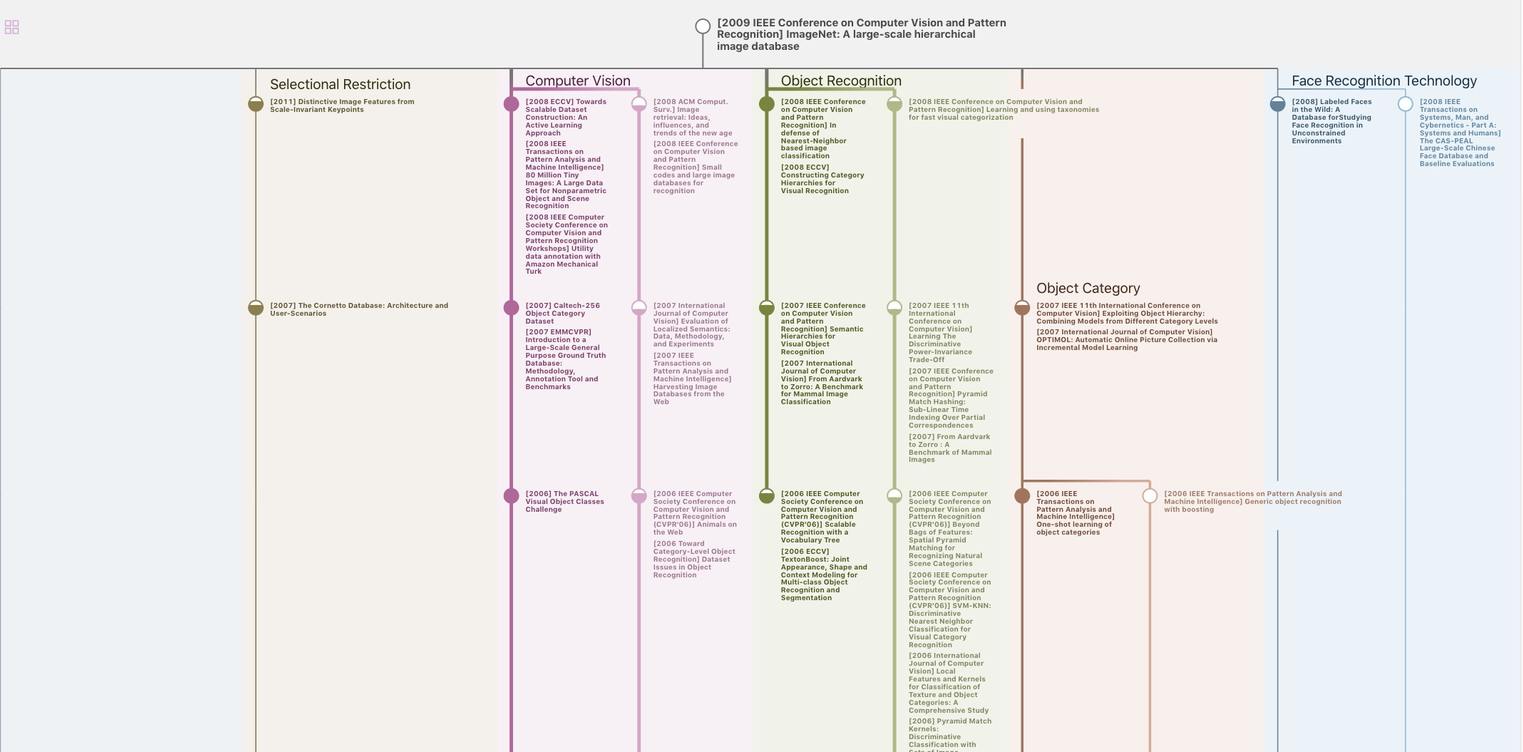
生成溯源树,研究论文发展脉络
Chat Paper
正在生成论文摘要