Research Hypothesis Generation Using Link Prediction in a Bipartite Graph
2018 IEEE International Conference on Big Data (Big Data)(2018)
摘要
The large volume of scientific publications is likely to have hidden knowledge that can be used for suggesting new research topics. We propose an automatic method that is helpful for generating research hypotheses in the field of physics using the massive number of physics journal publications. We convert the text data of titles and abstract sections in publications to a bipartite graph, extracting words of physical matter composed of chemical elements and extracting related keywords in the articles. The proposed method predicts the formation of new links between matter and keyword nodes based on collaborative filtering and matter popularity. The formation of links represents research hypotheses, as it suggests the new possible relationships between physical matter and keywords for physical properties or phenomena. The suggested method has better performance than existing methods for link prediction in the entire bipartite graph and the subgraph that contains only a specific keyword, such as 'antiferromagnetism' or 'superconductivity.' Our suggested concept for generating research hypotheses can be easily extended to various other research topics or industrial topics using patent literature.
更多查看译文
关键词
Hypothesis Generation,Text Mining,Link Prediction,Graph,Recommender Systems
AI 理解论文
溯源树
样例
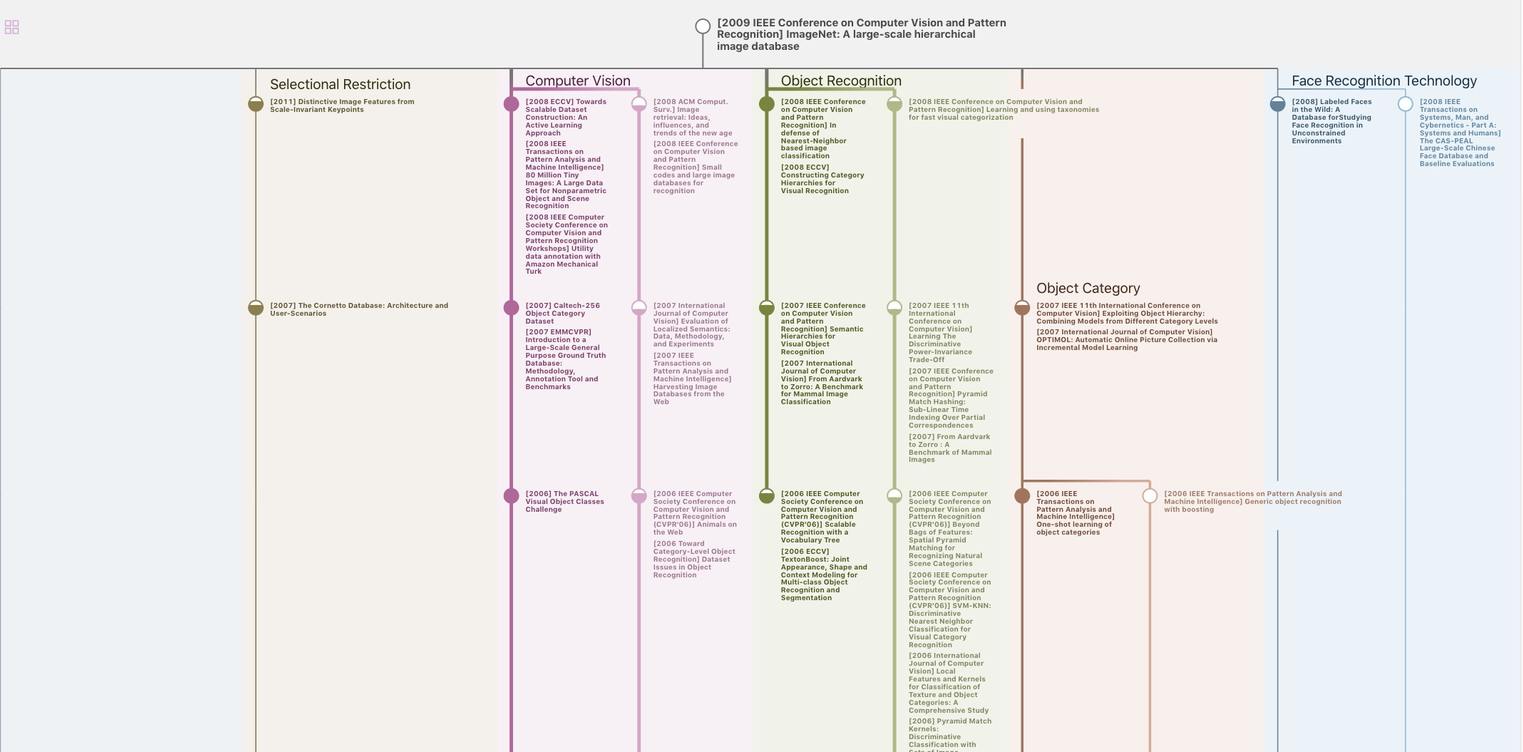
生成溯源树,研究论文发展脉络
Chat Paper
正在生成论文摘要