Robust Covariance Estimation for Approximate Factor Models.
Journal of Econometrics(2019)
摘要
In this paper, we study robust covariance estimation under the approximate factor model with observed factors. We propose a novel framework to first estimate the initial joint covariance matrix of the observed data and the factors, and then use it to recover the covariance matrix of the observed data. We prove that once the initial matrix estimator is good enough to maintain the element-wise optimal rate, the whole procedure will generate an estimated covariance with desired properties. For data with bounded fourth moments, we propose to use adaptive Huber loss minimization to give the initial joint covariance estimation. This approach is applicable to a much wider class of distributions, beyond sub-Gaussian and elliptical distributions. We also present an asymptotic result for adaptive Huber’s M-estimator with a diverging parameter. The conclusions are demonstrated by extensive simulations and real data analysis.
更多查看译文
关键词
C13,C38
AI 理解论文
溯源树
样例
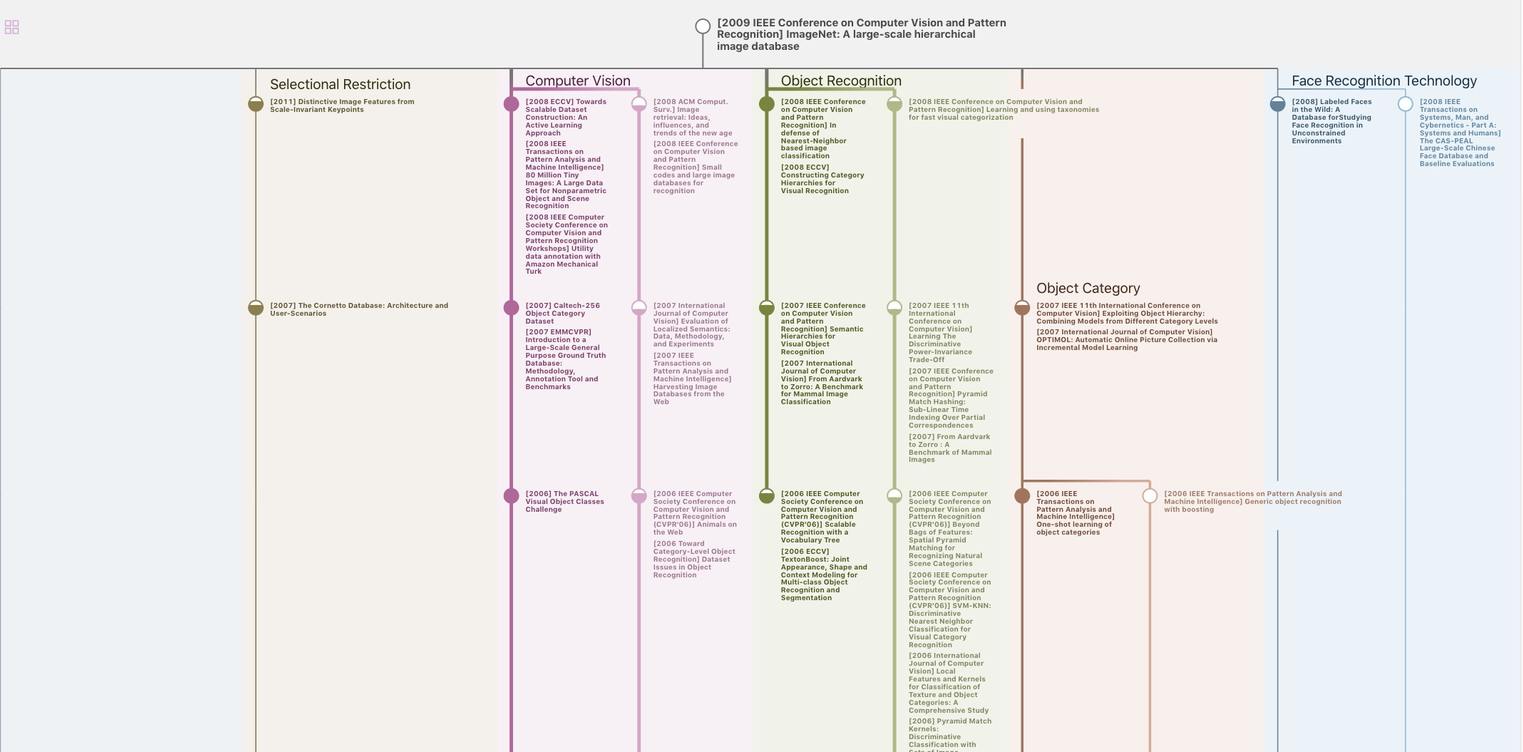
生成溯源树,研究论文发展脉络
Chat Paper
正在生成论文摘要