Deep-learning based surface region selection for deep inspiration breath hold (DIBH) monitoring in left breast cancer radiotherapy.
PHYSICS IN MEDICINE AND BIOLOGY(2018)
摘要
Deep inspiration breath hold (DIBH) with surface supervising is a common technique for cardiac dose reduction in left breast cancer radiotherapy. Surface supervision accuracy relies on the characteristics of surface region. In this study, a convolutional neural network (CNN) based automatic region-of-interest (ROI) selection method was proposed to select an optimal surface ROI for DIBH surface monitoring. The curvature entropy and the normal of each vertex on the breast cancer patient surface were calculated and formed as representative maps for ROI selection learning. 900 ROIs were randomly extracted from each patient's surface representative map, and the corresponding rigid ROI registration errors (REs) were calculated. The VGG-16 (a 16-layer network structure developed by Visual Geometry Group(VGG) from University of Oxford) pre-trained on a large natural image database ImageNet were fine-tuned using 27 thousand extracted ROIs and the corresponding REs from thirty patients. The RE prediction accuracy of the trained model was validated on additional ten patients. Satisfactory RE predictive accuracies were achieved with the root mean square error (RMSE)/mean absolute error (MAE) smaller than 1 mm/0.7 mm in translations and 0.45 degrees/0.35 degrees in rotations, respectively. The REs of the model selected ROls on ten testing cases is close to the minimal predicted RE with mean RE differences <1 mm and <0.5 degrees for translation and rotation, respectively. The proposed RE predictive model can be utilized for selecting a quasi-optimal ROI in left breast cancer DIBH radiotherapy (DIBH-RT).
更多查看译文
关键词
transfer learning,DIBH,ROI selection,motion monitoring
AI 理解论文
溯源树
样例
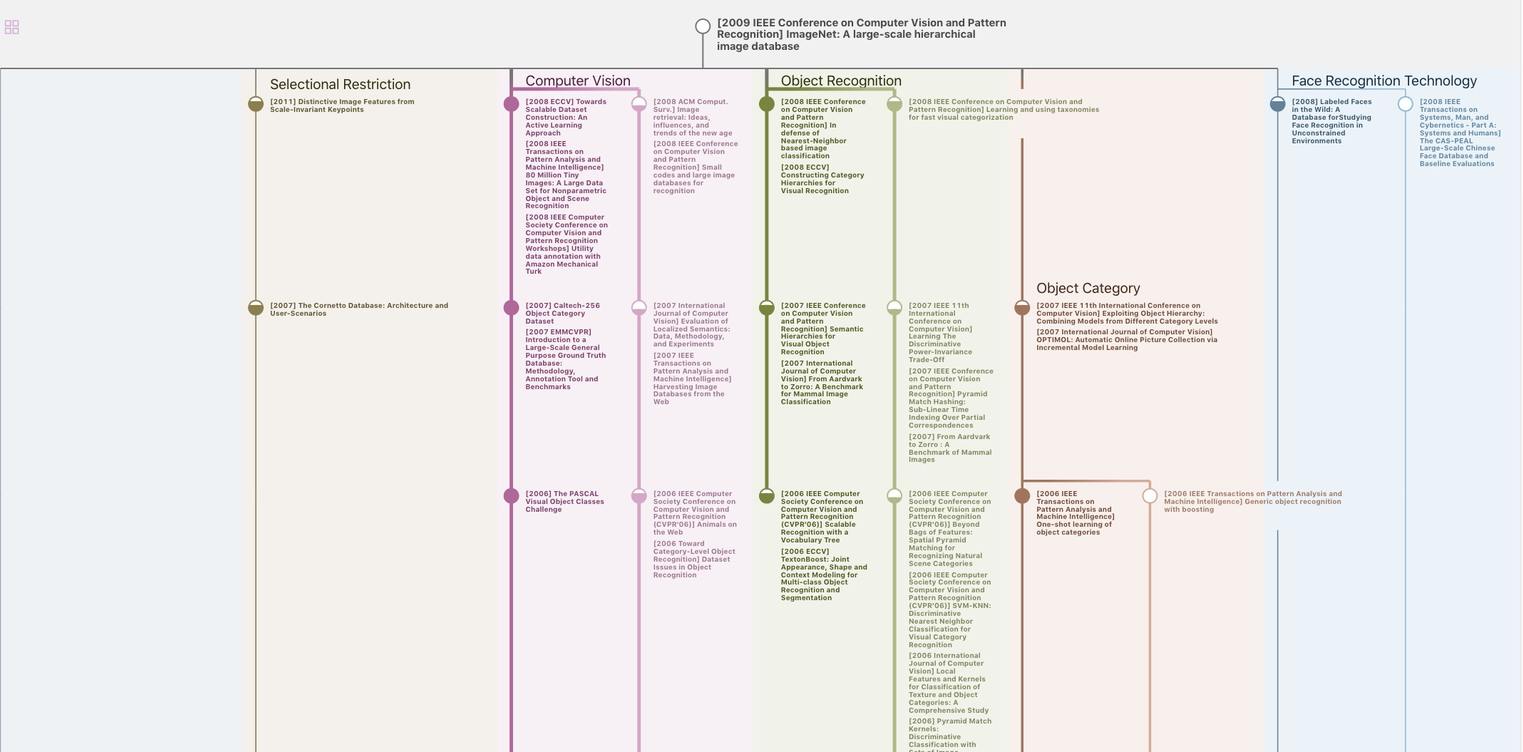
生成溯源树,研究论文发展脉络
Chat Paper
正在生成论文摘要