A Comparison Of Multiple Testing Adjustment Methods With Block-Correlation Positively-Dependent Tests
PLOS ONE(2017)
摘要
In high dimensional data analysis (such as gene expression, spatial epidemiology, or brain imaging studies), we often test thousands or more hypotheses simultaneously. As the number of tests increases, the chance of observing some statistically significant tests is very high even when all null hypotheses are true. Consequently, we could reach incorrect conclusions regarding the hypotheses. Researchers frequently use multiplicity adjustment methods to control type I error rates D primarily the family-wise error rate (FWER) or the false discovery rate (FDR) D while still desiring high statistical power. In practice, such studies may have dependent test statistics (or p-values) as tests can be dependent on each other. However, some commonly-used multiplicity adjustment methods assume independent tests. We perform a simulation study comparing several of the most common adjustment methods involved in multiple hypothesis testing, under varying degrees of block-correlation positive dependence among tests.
更多查看译文
关键词
multiple testing adjustment methods,tests,block-correlation,positively-dependent
AI 理解论文
溯源树
样例
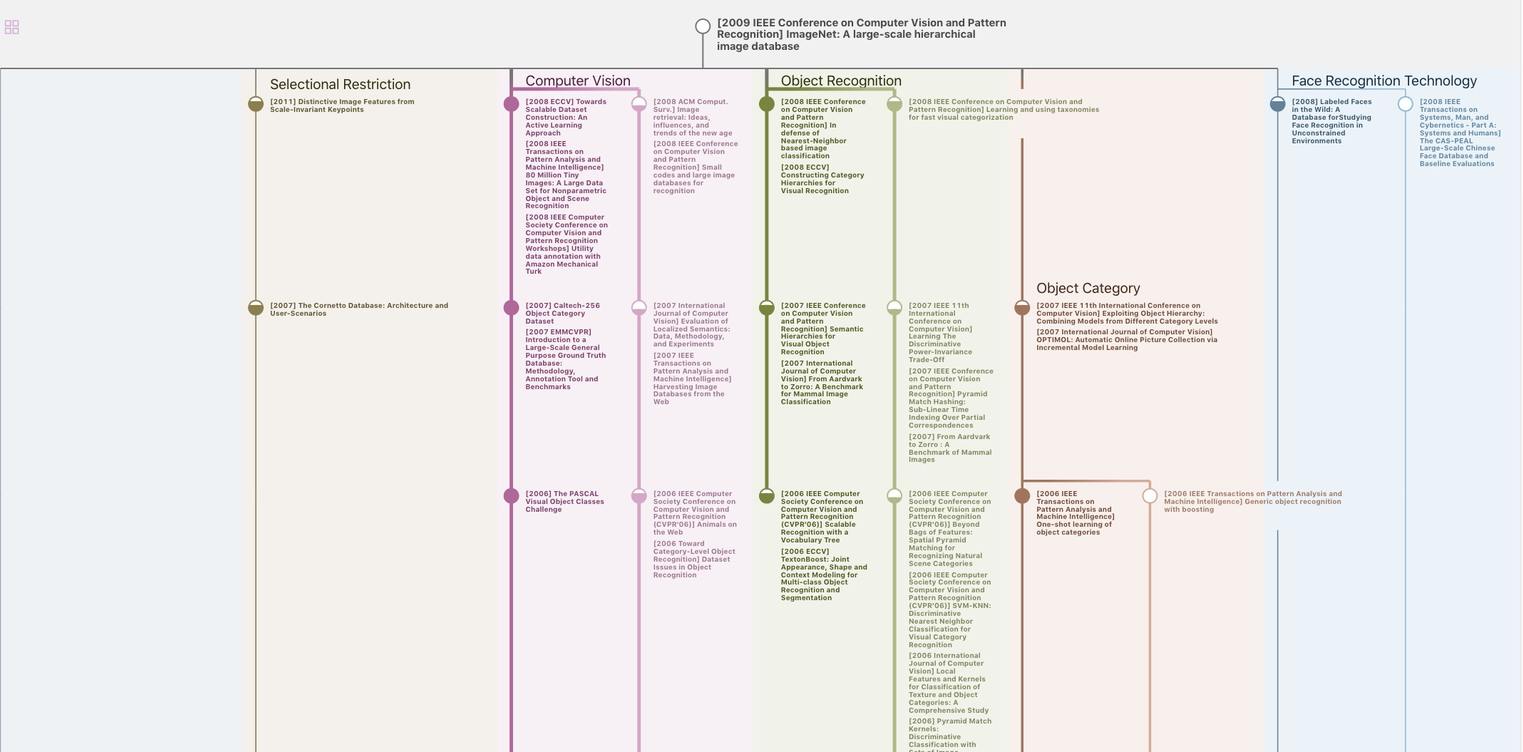
生成溯源树,研究论文发展脉络
Chat Paper
正在生成论文摘要