Comparison of Risk Prediction With the CKD-EPI and MDRD Equations in Non-ST-Segment Elevation Acute Coronary Syndrome.
CLINICAL CARDIOLOGY(2016)
Abstract
Background: Chronic Kidney Disease Epidemiology Collaboration (CKD-EPI) equations estimate glomerular filtration rate (GFR) more accurately than the Modification of Diet in Renal Disease (MDRD) equation. Hypothesis: New CKD-EPI equations improve risk stratification in patients with non-ST-segment elevation acute coronary syndrome (NSTE-ACS) and provide complementary information to the Global Registry of Acute Coronary Events (GRACE) risk score. Methods: We studied 350 subjects (mean age, 68 +/- 12 years; 70% male) with NSTE-ACS. Estimated GFR was calculated using the MDRD and new CKD-EPI equations based on serum creatinine (SCr) and/or cystatin C (CysC) concentrations obtained within 48 hours of hospital admission. The primary endpoint was all-cause death during follow-up. Results: Over the study period (median, 648 days [interquartile range, 236-1042 days]), 31 patients died (0.05% events per person-year). Decedents had poorer renal-function parameters (P < 0.001). Both CysC-based CKD-EPI equations had the highest areas under the receiver operating characteristic curve for the prediction of all-cause mortality. After multivariate adjustment, only CysC-based CKD-EPI equations were independent predictors of all-cause mortality (CKD-EPISCr-CysC, per mL/min/1.73 m(2): hazard ratio: 0.975, 95% confidence interval: 0.956-0.994, P = 0.009; CKD-EPICysC, per mL/min/1.73 m(2:) hazard ratio: 0.976, 95% confidence interval: 0.959-0.993, P = 0.005). Reclassification analyses showed that only CysC-based CKD-EPI equations improved predictive accuracy of the GRACE risk score. Conclusions: In patients with NSTE-ACS, CysC-based CKD-EPI equations improved clinical risk stratification for mortality and added complementary prognostic information to the GRACE risk score.
MoreTranslated text
Key words
risk prediction,ckd-epi,non-st-segment
AI Read Science
Must-Reading Tree
Example
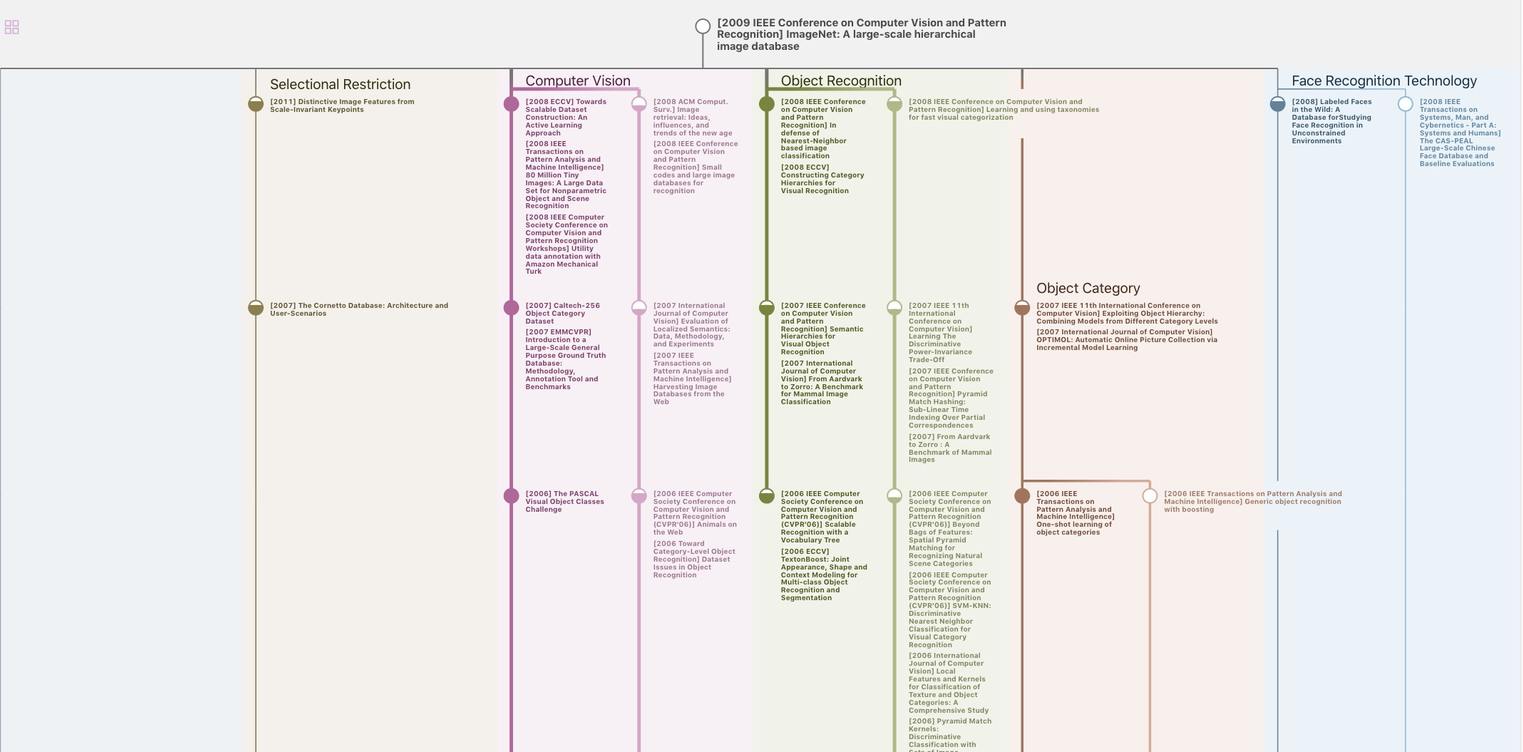
Generate MRT to find the research sequence of this paper
Chat Paper
Summary is being generated by the instructions you defined