Improving Social Media Popularity Prediction with Content-aware Post Dependencies
crossref(2024)
Abstract
Abstract Numerous social media posts exhibit inherent qualities that determine their popularity even before being published. Consequently, comprehending the patterns of post popularity and forecasting them before publication holds significant practical value. Existing work mainly utilizes information from individual posts to make predictions, ignoring the popularity correlation between different posts. By analyzing the dataset, we find that there is an important relationship between the content and popularity of different posts published in a short period. To address this challenge, we introduce Content-aware Post Dependency Networks (CPDN) that utilize information from content-related posts to improve the popularity prediction of target posts. CPDN employs multimodal feature adapters to create task-specific representations for target posts. Utilizing hierarchical category information, it captures nuanced correlations between posts. The model incorporates recurrent networks with gating layers and attention mechanisms to aggregate posts with correlated content for better prediction of target posts. The experimental results on Social Media Popularity Dataset under two different settings demonstrate the superiority of our method over the state-of-the-art methods. We release our code in \href{https://github.com/Daisy-zzz/CPDN.git}{Daisy-zzz/CPDN} for reproducibility.
MoreTranslated text
AI Read Science
Must-Reading Tree
Example
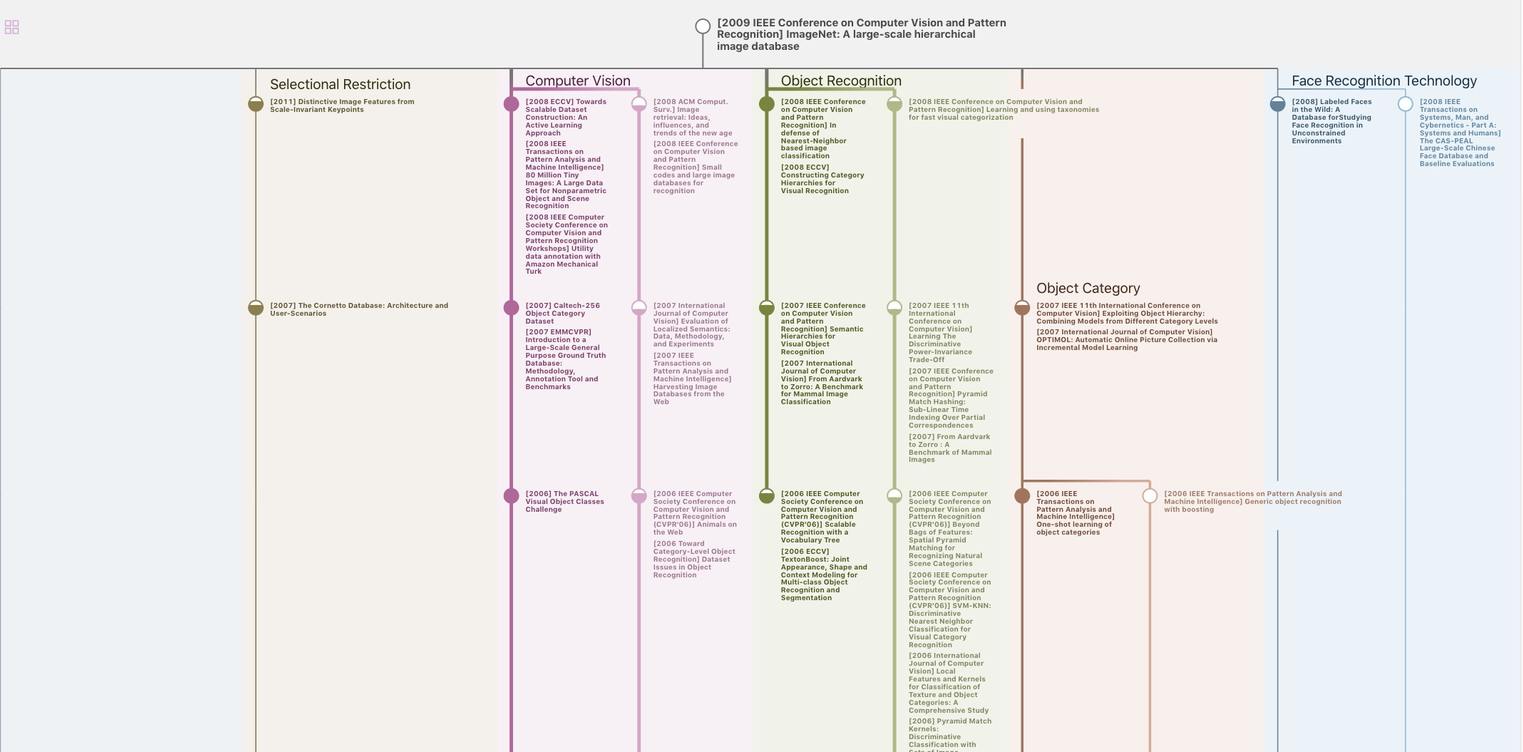
Generate MRT to find the research sequence of this paper
Chat Paper
Summary is being generated by the instructions you defined