From Minimum Change to Maximum Density: On Determining Near-Optimal S-Repair
IEEE TRANSACTIONS ON KNOWLEDGE AND DATA ENGINEERING(2024)
Abstract
Dirty data are commonly observed in real applications, making cleaning them a key step in data preparation. The widely adopted idea of cleaning dirty data is based on detecting conflicts w.r.t. integrity constraints. Typical S-repair methods remove a minimal set of tuples (to avoid excessive removal and information loss) such that integrity constraints are no longer violated in remaining tuples. Unfortunately, multiple candidates of minimal removal sets may exist and are difficult to determine which one is indeed proper. We intuitively notice that a clean tuple often has more close neighbors (i.e., higher density) than dirty tuples. Hence, in this paper, we study the problem of finding the optimal S-repair under integrity constraints with the highest density, among various minimal removal sets. Our major contributions include (1) the np-hardness analysis on solving the problem, (2) a heuristic algorithm for efficiently tackling the problem and returning the optimal solution in certain cases, (3) an approximation performance bounded method with the same optimal solution guarantee. Experiments on real datasets collected from industry with real-world errors demonstrate the superiority of our work in cleaning dirty tuples.
MoreTranslated text
Key words
Dirty data,S-repair,data cleaning
AI Read Science
Must-Reading Tree
Example
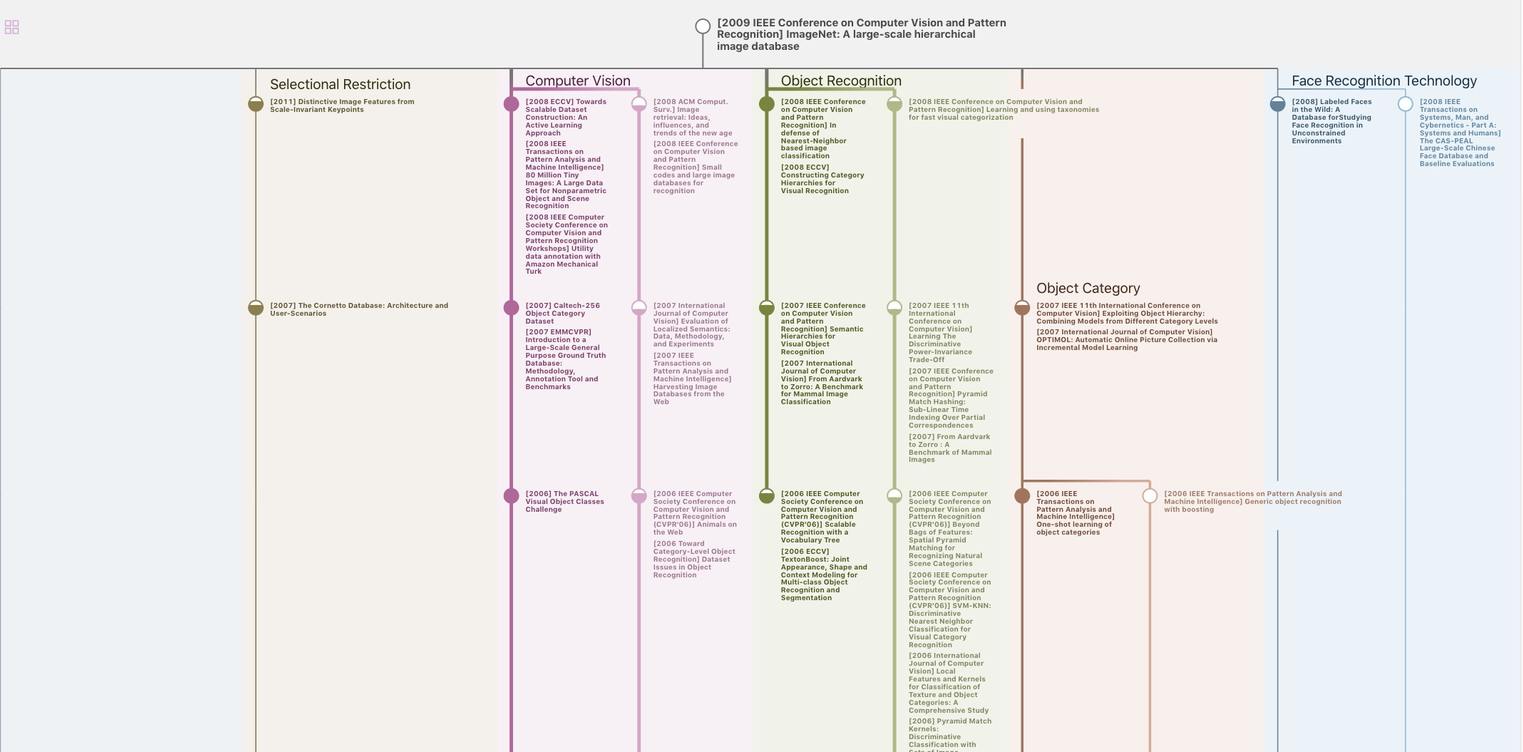
Generate MRT to find the research sequence of this paper
Chat Paper
Summary is being generated by the instructions you defined