Logical shadow tomography: Efficient estimation of error-mitigated observables
arXiv (Cornell University)(2022)
摘要
We introduce a technique to estimate error-mitigated expectation values on noisy quantum computers. Our technique performs shadow tomography on a logical state to produce a memory-efficient classical reconstruction of the noisy density matrix. Using efficient classical post-processing, one can mitigate errors by projecting a general nonlinear function of the noisy density matrix into the codespace. The subspace expansion and virtual distillation can be viewed as special cases of the new framekwork. We show our method is favorable in the quantum and classical resources overhead. Relative to subspace expansion which requires $O\left(2^{N} \right)$ samples to estimate a logical Pauli observable with $[[N, k]]$ error correction code, our technique requires only $O\left(4^{k} \right)$ samples. Relative to virtual distillation, our technique can compute powers of the density matrix without additional copies of quantum states or quantum memory. We present numerical evidence using logical states encoded with up to sixty physical qubits and show fast convergence to error-free expectation values with only $10^5$ samples under 1% depolarizing noise.
更多查看译文
关键词
logical shadow tomography,efficient estimation,error-mitigated
AI 理解论文
溯源树
样例
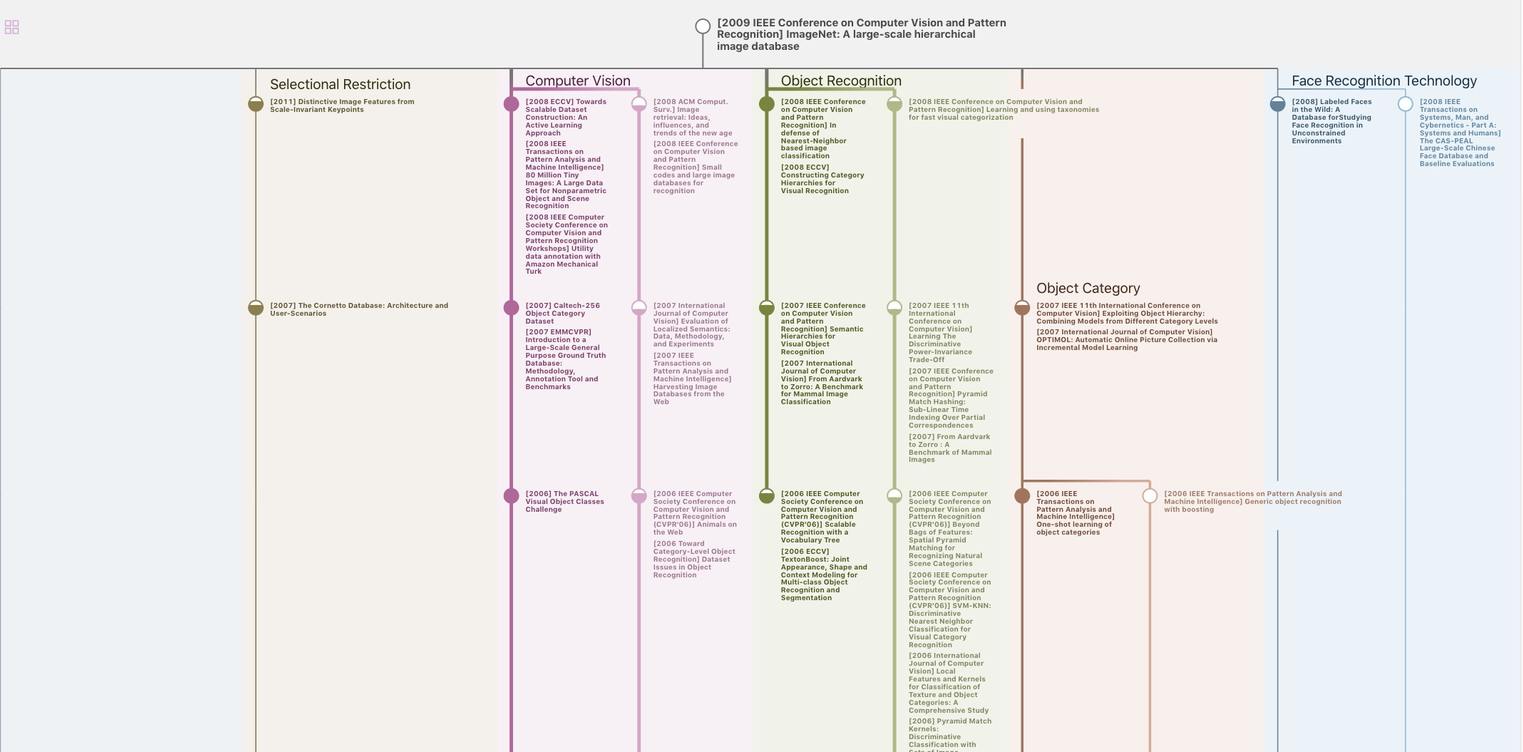
生成溯源树,研究论文发展脉络
Chat Paper
正在生成论文摘要