Learning from vertically distributed data across multiple sites: An efficient privacy-preserving algorithm for Cox proportional hazards model with variable selection
JOURNAL OF BIOMEDICAL INFORMATICS(2024)
摘要
Objective: To develop a lossless distributed algorithm for regularized Cox proportional hazards model with variable selection to support federated learning for vertically distributed data.Methods: We propose a novel distributed algorithm for fitting regularized Cox proportional hazards model when data sharing among different data providers is restricted. Based on cyclical coordinate descent, the proposed algorithm computes intermediary statistics by each site and then exchanges them to update the model parameters in other sites without accessing individual patient-level data. We evaluate the performance of the proposed algorithm with (1) a simulation study and (2) a real-world data analysis predicting the risk of Alzheimer's dementia from the Religious Orders Study and Rush Memory and Aging Project (ROSMAP). Moreover, we compared the performance of our method with existing privacy-preserving models.Results: Our algorithm achieves privacy-preserving variable selection for time-to-event data in the vertically distributed setting, without degradation of accuracy compared with a centralized approach. Simulation dem-onstrates that our algorithm is highly efficient in analyzing high-dimensional datasets. Real-world data analysis reveals that our distributed Cox model yields higher accuracy in predicting the risk of Alzheimer's dementia than the conventional Cox model built by each data provider without data sharing. Moreover, our algorithm is computationally more efficient compared with existing privacy-preserving Cox models with or without regularization term.Conclusion: The proposed algorithm is lossless, privacy-preserving and highly efficient to fit regularized Cox model for vertically distributed data. It provides a suitable and convenient approach for modeling time-to-event data in a distributed manner.
更多查看译文
关键词
Distributed algorithm,Cox proportional hazards model,Privacy preserving,Variable selection,Vertical partitioning
AI 理解论文
溯源树
样例
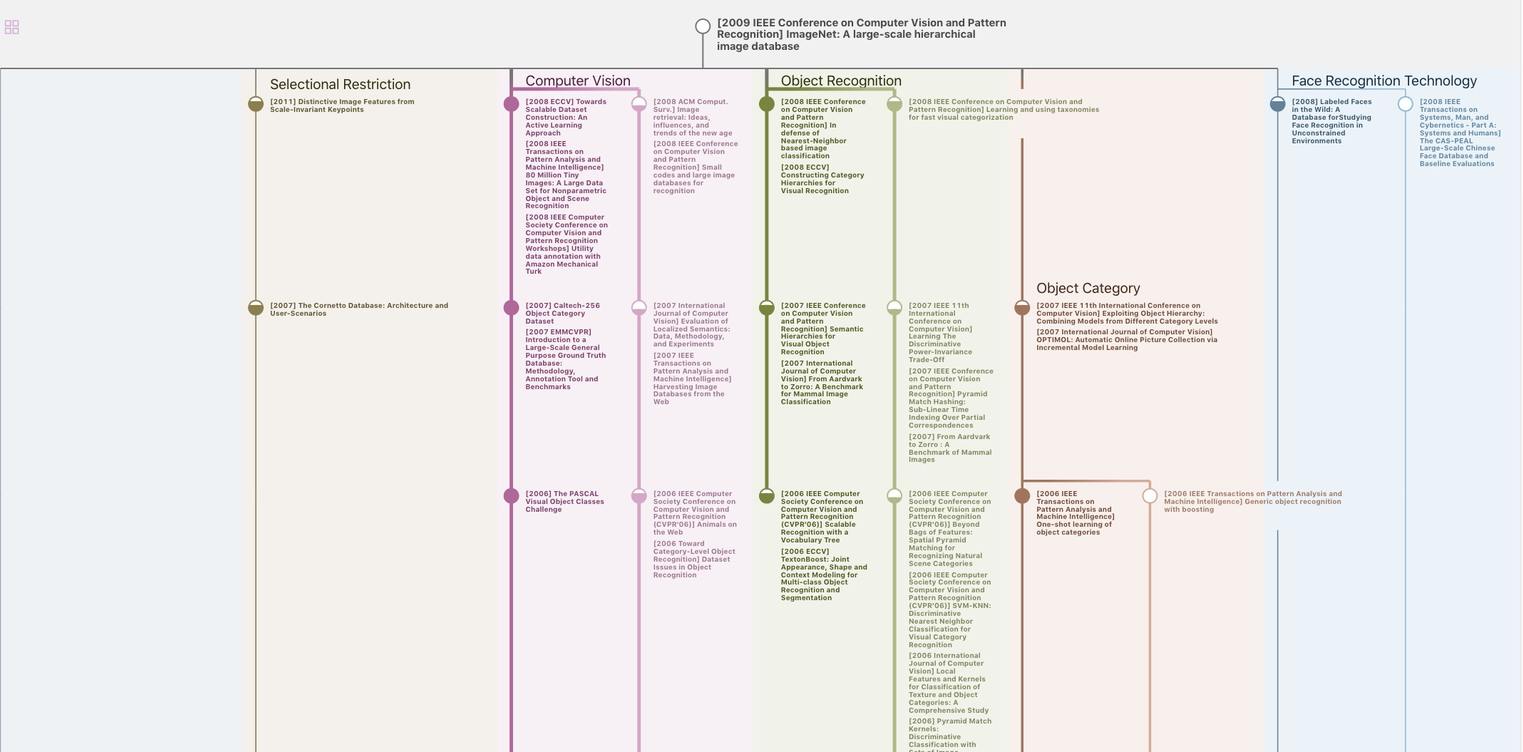
生成溯源树,研究论文发展脉络
Chat Paper
正在生成论文摘要