The convergence of artificial intelligence and chemistry for improved drug discovery.
FUTURE MEDICINAL CHEMISTRY(2018)
摘要
Future Medicinal ChemistryVol. 10, No. 22 CommentaryThe convergence of artificial intelligence and chemistry for improved drug discoveryClive P Green, Ola Engkvist & Garry PairaudeauClive P Green*Author for correspondence: E-mail Address: clive.green@astrazeneca.com Sample Management, Discovery Sciences, IMED Biotech Unit, AstraZeneca, Cambridge, UK, Ola Engkvist Hit Discovery, Discovery Sciences, IMED Biotech Unit, AstraZeneca, Gothenburg, Sweden & Garry Pairaudeau Hit Discovery, Discovery Sciences, IMED Biotech Unit, AstraZeneca, Cambridge, UKPublished Online:30 Nov 2018https://doi.org/10.4155/fmc-2018-0161AboutSectionsPDF/EPUB ToolsAdd to favoritesDownload CitationsTrack Citations ShareShare onFacebookTwitterLinkedInReddit Keywords: artificial intelligencecompound designlead discoverysynthesis planningRecent evidence from AstraZeneca suggests that a major revision to research strategy can result in greater success rates for preclinical projects reaching lead optimization [1]. In the period 2005–2010 only 23% of new projects delivered leads of sufficient quality to support lead optimization. The earlier use of toxicity assays and a long-term focus on core disease areas more than doubled this to 48%. However, if the bold ambitions of pharmaceutical companies to develop novel innovative drugs are to be achieved, particularly for challenging but highly validated targets, continued improvement in lead discovery research is required. The pharmaceutical sector, like many others, is considering the potential of artificial intelligence (AI) to address key problems impacting drug discovery productivity. With notable recent successes in natural language processing and voice and image recognition, a growth in computational power and accessibility to larger and more complex datasets through the application of high-throughput assay technologies, AI is now frequently badged as the answer to delivering new medicines to patients faster. Here, we share our perspective on the current state of AI within medicinal chemistry, the key steps to deliver experimental validation and the critical interdependencies with other technologies that must be developed in parallel if AI is to improve preclinical research productivity.Computational drug designSince AI was first crystallized in Alan Turing's famous work of the 1950's, there have been several developments that have gathered huge interest and inflated expectations. The focus of the current decade is deep learning [2], a subfield of machine learning, which uses artificial neural networks loosely inspired by the structure of the human brain. While AI is not new, neither is the desire of chemists to predict structure–activity relationships. Since the precomputer era, when Hammett related reaction rates and equilibrium constants for reactions of benzoic acid derivatives, or Hansch's pioneering computer-assisted identification and quantification of physicochemical properties of drugs on biological activity, chemists have strived to make accurate predictions with confidence and focus laboratory testing on the highest value scientific activities.It is toward this end that chemists have begun to investigate the application of AI to the two fundamental questions in medicinal chemistry, 'what compound should I make next?' and 'how can I make it?' In the last 40 years, the scientific discipline of chemoinformatics has created a sophisticated suite of computational drug design tools, ranging from classical structure-based QSAR techniques [3] to more recent advances in matched molecular pairs [4] and free-energy perturbation [5]; with most recent medicines having utilized these methods in some way. In addition, chemists can readily search the entire published chemical literature to aid synthesis planning. While these are powerful tools it is important to make an early distinction that coupling existing drug design methods in an automated process does not, in our view, constitute AI.Designing new molecules & planning synthesis with AIAny true AI system for de novo molecular design must have the fundamental intelligence to generate independently novel molecular representations that are drug-like and chemically sensible. It is no surprise therefore that considerable efforts in the research community have been focused on this challenge, with notable achievements exemplified by molecular graph convolution methods [6], variational autoencoders [7] and recurrent neural networks (RNN) [8]. In recent research from Olivecrona and co-workers at AstraZeneca [9], an RNN was trained on simplified molecular-input line-entry system (SMILES) representations from the ChEMBL database. Policy-based reinforcement learning was used to tune the pretrained RNNs and generate novel sequences that followed the conditional probability distributions learned from the training set. Of the sequences generated by the network, 94% corresponded to valid molecular structures, of which 90% were novel.Once molecular structure inputs are available, machine learning and neural network algorithms can be deployed routinely in compound property and activity prediction. A recent increase in the scale and complexity of neural networks has seen a renewed interest in their application, with multitask deep neural networks (DNN) outperforming other machine-learning methods in some cases. The work of Olivecrona et al. optimized a model toward generating novel structures with predicted biological activity against DRD2 [9]. The model generated structures of which >95% were predicted to be active, including experimentally confirmed actives that were not included in either the generative model or the activity prediction model. While the prediction of dopamine activity is a relatively straightforward problem, this work demonstrated the ability to autonomously generate molecules and then learn from their scoring against a predictive model.Perhaps the greatest scepticism for AI in medicinal chemistry that we encounter is reserved for chemical synthesis planning. This is unsurprising when one considers that: retrosynthesis is a revered, creative process that requires extensive chemical knowledge; and the standard methodology of automated rule-based methods for forward reaction prediction lacks the necessary chemical intelligence to provide broad coverage and confidence in the output. The advent of DNN methods for synthesis prediction could offer an improvement [10]. In one approach, Segler et al. used a training set of 3.5 million reactions to create a DNN capable of performing reaction prediction and retrosynthetic analysis with an accuracy of 97 and 95%, respectively [11]. In a separate study, they used a training set of 12 million reactions and a system that combined policy networks and Monte Carlo tree search for retrosynthetic prediction [12]. Their system solved almost twice as many molecules and was 30-times faster in comparison to traditional rules-based methods. More impressively, chemists could not distinguish between synthetic routes generated by a computer system and routes reported by expert chemists in the literature. The same authors also reported the application of graph theory for reaction prediction that outperformed rule-based methods for 180,000 randomly selected binary reactions and offers the exciting potential to propose novel chemical reactions [13]. While we are still some distance from AI replicating some of the great achievements in total synthesis of complex natural products, a tool to rapidly triage synthetic routes to routine molecules seems a tangible goal.The challenges ahead & charting progressWith the component parts of an AI medicinal chemistry solution seemingly within our grasp, it becomes plausible to consider the steps required to leverage this technology in pursuit of improved preclinical drug discovery productivity. First, it is important to recognize that AI in drug discovery faces many significant challenges, such as the dependency on using experimental data for training and validation, and the scoring of compounds binding to proteins. This differs to recent AI successes in games like Go and chess where as much training data as needed can be automatically generated and perfect scoring functions exist to train models. Therefore, continuous and specific innovation in AI must be undertaken to address the unique challenges faced in medicinal chemistry and the complexity of drug discovery data. Significant progress is being made in areas such as one-shot learning [14], transfer learning [15] and conformal prediction [16], with recent advances in free-energy perturbation [5] offering potential to address the scoring challenge. Second, we should recognize that our current human-led processes are far from infallible and we lack data to quantify medicinal chemistry success. As such we should be mindful not to set the benchmark for AI approaches unrealistically high. Third, to realize the full impact of AI techniques in the future we will require considerable resources to be invested in data curation, integration and stewardship.Experimental validation of AI on real-world drug discovery projects, both in competition with, and augmenting, conventional human-led processes, is a critical next step in learning how AI can contribute to medicinal chemistry and to focus AI innovation. The commitment of resources and budget, in particular, to rapid synthesis of compounds identified by AI design, is essential during this phase. While one can envisage a strong rationale for applying AI across all stages of drug discovery, our current focus is in lead generation. In this phase the optimization of molecules is primarily driven by in vitro properties, which enables the data to be derived in rapid learning cycles utilizing our vast historic collection of relevant physicochemical, absorption, distribution, metabolism, and excretion (ADME) and in vitro toxicological data to develop predictive models.Our hypothesis, that AI has the potential to find the best series of molecules for in vivo optimization, is predicated on one other major technology breakthrough – a significant development in automated chemical synthesis. When coupled with the aforementioned improvement in automated chemical synthesis planning there exists the possibility to transform the time to synthesize clusters of compounds of interest from anywhere in chemical space. Through this paradigm AI exploration can be propelled by enough data to make further design iterations with high confidence. Notable recent advancements have been made in automating chemistry [17], although we suggest that given the immense size of drug-like and chemical synthesis reaction space a far greater focus is needed on addressing challenges of automating three-to-five-step synthesis incorporating major medicinal chemistry reaction classes [18]. In so doing, we could look to AI for a more objective design approach that offers a powerful complement to the traditional medicinal chemistry practice of multicomponent optimization of drug properties. After consideration of the potential impact of AI and automated chemistry, we propose five levels of AI-driven drug discovery (Table 1), analogous to the five levels of autonomous driving [19], to chart the progress of these rapidly advancing technologies. We expect that the significant practical challenges associated with automating chemistry will cause it to lag behind AI-driven drug design. As compound synthesis remains the rate-determining step for in vitro property optimization it is realistic to assume that humans will want to retain control over the compounds that enter synthesis. By contrast, research by Delaney [20] suggests that medicinal and agrochemical projects are currently operating at the level of a self-avoiding walk, with the intriguing possibility that it might actually be more efficient to have algorithms in charge today. While we doubt that many projects would currently be willing to cede this level of control, the research provides evidence that system control of medicinal chemistry could be achievable and may be advantageous if automated synthesis can be routinely adopted.Table 1. Five levels of artificial intelligence-driven drug discovery.LevelNameDefinitionDecision on what compounds to make0No assistanceManual design, synthesis and analysisHuman1Analytical assistanceInput from computational analysisHuman2Partial design assistanceHuman design and synthesis with occasional input from AI design methodsHuman3Augmented design and partial synthesis assistanceAI design and partial automated synthesis with significant input from humans in both design and synthesisHuman4Conditional automationAI design and automated synthesis with occasional input from humansHuman and system5High automationAI design and automated synthesis with minimal or no input from humansSystemAI: Artificial intelligence.ConclusionIn summary, we believe the recent focus on AI has yielded promising results that now warrant investment in experimental validation of AI design for medicinal chemistry. It is prudent, given the lack of real-world project evidence, to target initially incremental productivity improvements through the application of AI; with the true impact only likely to emerge from a significant concomitant development of automated chemistry. Time will tell how valuable these techniques will be in drug discovery; however, if the impacts in other areas of society are any benchmark we can expect to see significant changes over the next decade.AcknowledgementsThe authors thank T Kogej, H Chen, C Tyrchan, W Czechtizky, L Carlsson and C Bendtsen for valuable discussion on AI and machine learning.Financial & competing interests disclosureThe authors have no relevant affiliations or financial involvement with any organization or entity with a financial interest in or financial conflict with the subject matter or materials discussed in the manuscript. This includes employment, consultancies, honoraria, stock ownership or options, expert testimony, grants or patents received or pending, or royalties.No writing assistance was utilized in the production of this manuscript.Open accessThis work is licensed under the Attribution-NonCommercial-NoDerivatives 4.0 Unported License. To view a copy of this license, visit http://creativecommons.org/licenses/by-nc-nd/4.0/Papers of special note have been highlighted as: • of interestReferences1 Morgan P, Brown DG, Lennard S et al. Impact of a five-dimensional framework on R&D productivity at AstraZeneca. Nat. Rev. Drug Discov. 17, 167–181 (2018).Crossref, Medline, CAS, Google Scholar2 Chen H, Engkvist O, Wang Y, Olivecrona M, Blaschke T. The rise of deep learning in drug discovery. Drug Discov. Today 23(6), 1241–1250 (2018). • A comprehensive recent review of deep learning in drug discovery.Crossref, Medline, Google Scholar3 Cherkasov A, Muratov EN, Fourches D et al. QSAR modeling: where have you been? where are you going to? J. Med. Chem. 57(12), 4977–5010 (2014).Crossref, Medline, CAS, Google Scholar4 Tyrchan C, Evertsson E. Matched molecular pair analysis in short: algorithms, applications and limitations. Comput. Struct. Biotechnol. J. 15, 86–90 (2016).Crossref, Medline, Google Scholar5 Shivakumar D, Williams J, Wu Y, Damm W, Shelley J, Sherman W. Prediction of absolute solvation free energies using molecular dynamics free energy perturbation and the OPLS force field. J. Chem. Theory Comput. 6(5), 1509–1519 (2010).Crossref, Medline, CAS, Google Scholar6 Duvenaud D, Maclaurin D, Aguilera-Iparraguirre J et al. Convolutional networks on graphs for learning molecular fingerprints. Proceedings of the 29th Annual Conference on Neural Information Processing Systems. Montreal, Canada, 2224–2232, 7–12 December 2015.Google Scholar7 Gómez-Bombarelli R, Wei JN, Duvenaud D et al. Automatic chemical design using a data-driven continuous representation of molecules. ACS Cent. Sci. 4(2), 268–276 (2018).Crossref, Medline, CAS, Google Scholar8 Segler MHS, Kogej T, Tyrchan C, Waller MP. Generating focused molecule libraries for drug discovery with recurrent neural networks. ACS Cent. Sci. 4(1), 120–131 (2018).Crossref, Medline, CAS, Google Scholar9 Olivecrona M, Blaschke T, Engkvist O, Chen H. Molecular de-novo design through deep reinforcement learning. J. Cheminform. 9(1), 1–14 (2017). • An example of deep learning techniques performing de novo design to identify compounds that have been experimentally confirmed as active at a biological receptor.Crossref, Medline, Google Scholar10 Engkvist O, Norrby P-O, Selmi N et al. Computational prediction of chemical reactions: current status and outlook. Drug Discov. Today 23(6), 1203–1218 (2018).Crossref, Medline, CAS, Google Scholar11 Segler MHS, Waller MP. Neural-symbolic machine learning for retrosynthesis and reaction prediction. Chem. Eur. J. 23(25), 5966–5971 (2017).Crossref, Medline, CAS, Google Scholar12 Segler MHS, Preuss M, Waller MP. Planning chemical syntheses with deep neural networks and symbolic AI. Nature 555, 604–610 (2018). • The application of deep learning to plan chemical synthesis routes that are indistinguishable from those generated by humans.Crossref, Medline, CAS, Google Scholar13 Segler MHS, Waller MP. Modeling chemical reasoning to predict and invent reactions. Chem. Eur. J. 23(25), 6118–6128 (2017).Crossref, Medline, CAS, Google Scholar14 Altae-Tran H, Ramsundar B, Pappu AS, Pande V. Low data drug discovery with one-shot learning. ACS Cent. Sci. 3(4), 283–293 (2017).Crossref, Medline, CAS, Google Scholar15 Simões RS, Maltarollo VG, Oliveira PR, Honorio KM. Transfer and multi-task learning in QSAR modeling: advances and challenges. Front. Pharmacol. 9(74), PMC5807924 (2018).Google Scholar16 Ahlberg E, Winiwarter S, Boström H et al. Using conformal prediction to prioritize compound synthesis in drug discovery. Proc. Machine Learn. Res. 60, 1–11 (2017).Google Scholar17 Schneider G. Automating drug discovery. Nat. Rev. Drug Discov. 17, 97–113 (2018).Crossref, Medline, CAS, Google Scholar18 Schneider N, Lowe DM, Sayle RA, Tarselli MA, Landrum GA. Big data from pharmaceutical patents: a computational analysis of medicinal chemists' bread and butter. J. Med. Chem. 59, 4385–4402 (2016).Crossref, Medline, CAS, Google Scholar19 SAE International. Taxonomy and definitions for terms related to on-road motor vehicle automated driving systems J3016_201401. www.sae.org/standards/content/j3016_201401/.Google Scholar20 Delaney J. Modelling iterative compound optimisation using a self-avoiding walk. Drug Discov. Today 14(3–4), 198–207 (2009). • A proposal to simulate the essential behavior of optimization projects using a simple self-avoiding walk model.Crossref, Medline, CAS, Google ScholarFiguresReferencesRelatedDetailsCited ByMolecular targets of insecticides and herbicides – Are there useful overlaps?Pesticide Biochemistry and Physiology, Vol. 191Role of Artificial Intelligence and Machine Learning in Drug Discovery and Drug RepurposingThe Impact of Artificial Intelligence on Waste Management for Climate Change12 March 2023Coverage Score: A Model Agnostic Method to Efficiently Explore Chemical Space22 July 2022 | Journal of Chemical Information and Modeling, Vol. 62, No. 18Defining Levels of Automated Chemical Design5 May 2022 | Journal of Medicinal Chemistry, Vol. 65, No. 10Prediction of Compound Synthesis Accessibility Based on Reaction Knowledge Graph3 February 2022 | Molecules, Vol. 27, No. 3Artificial Intelligence in Compound Design4 November 2021Magic bullets: Drug repositioning and drug combinationsProbabilistic Random Forest improves bioactivity predictions close to the classification threshold by taking into account experimental uncertainty19 August 2021 | Journal of Cheminformatics, Vol. 13, No. 1Emerging role of artificial intelligence in waste management practicesIOP Conference Series: Earth and Environmental Science, Vol. 889, No. 1Machine learning techniques applied to the drug design and discovery of new antivirals: a brief look over the past decade7 May 2021 | Expert Opinion on Drug Discovery, Vol. 16, No. 9A comprehensive review on the application of artificial intelligence in drug discovery.16 June 2021 | The Applied Biology & Chemistry JournalUncertainty quantification in drug designDrug Discovery Today, Vol. 26, No. 2Artificial Intelligence in Medicine in the United States, China and India4 December 2020"Ring Breaker": Neural Network Driven Synthesis Prediction of the Ring System Chemical Space30 April 2020 | Journal of Medicinal Chemistry, Vol. 63, No. 16Datasets and their influence on the development of computer assisted synthesis planning tools in the pharmaceutical domain1 January 2020 | Chemical Science, Vol. 11, No. 1Deep Learning for Deep Chemistry: Optimizing the Prediction of Chemical Patterns26 November 2019 | Frontiers in Chemistry, Vol. 7Concepts of Artificial Intelligence for Computer-Assisted Drug Discovery11 July 2019 | Chemical Reviews, Vol. 119, No. 18 Vol. 10, No. 22 Follow us on social media for the latest updates Metrics History Received 4 May 2018 Accepted 10 October 2018 Published online 30 November 2018 Published in print November 2018 Information© 2018 Clive GreenKeywordsartificial intelligencecompound designlead discoverysynthesis planningAcknowledgementsThe authors thank T Kogej, H Chen, C Tyrchan, W Czechtizky, L Carlsson and C Bendtsen for valuable discussion on AI and machine learning.Financial & competing interests disclosureThe authors have no relevant affiliations or financial involvement with any organization or entity with a financial interest in or financial conflict with the subject matter or materials discussed in the manuscript. This includes employment, consultancies, honoraria, stock ownership or options, expert testimony, grants or patents received or pending, or royalties.No writing assistance was utilized in the production of this manuscript.Open accessThis work is licensed under the Attribution-NonCommercial-NoDerivatives 4.0 Unported License. To view a copy of this license, visit http://creativecommons.org/licenses/by-nc-nd/4.0/PDF download
更多查看译文
关键词
artificial intelligence,compound design,lead discovery,synthesis planning
AI 理解论文
溯源树
样例
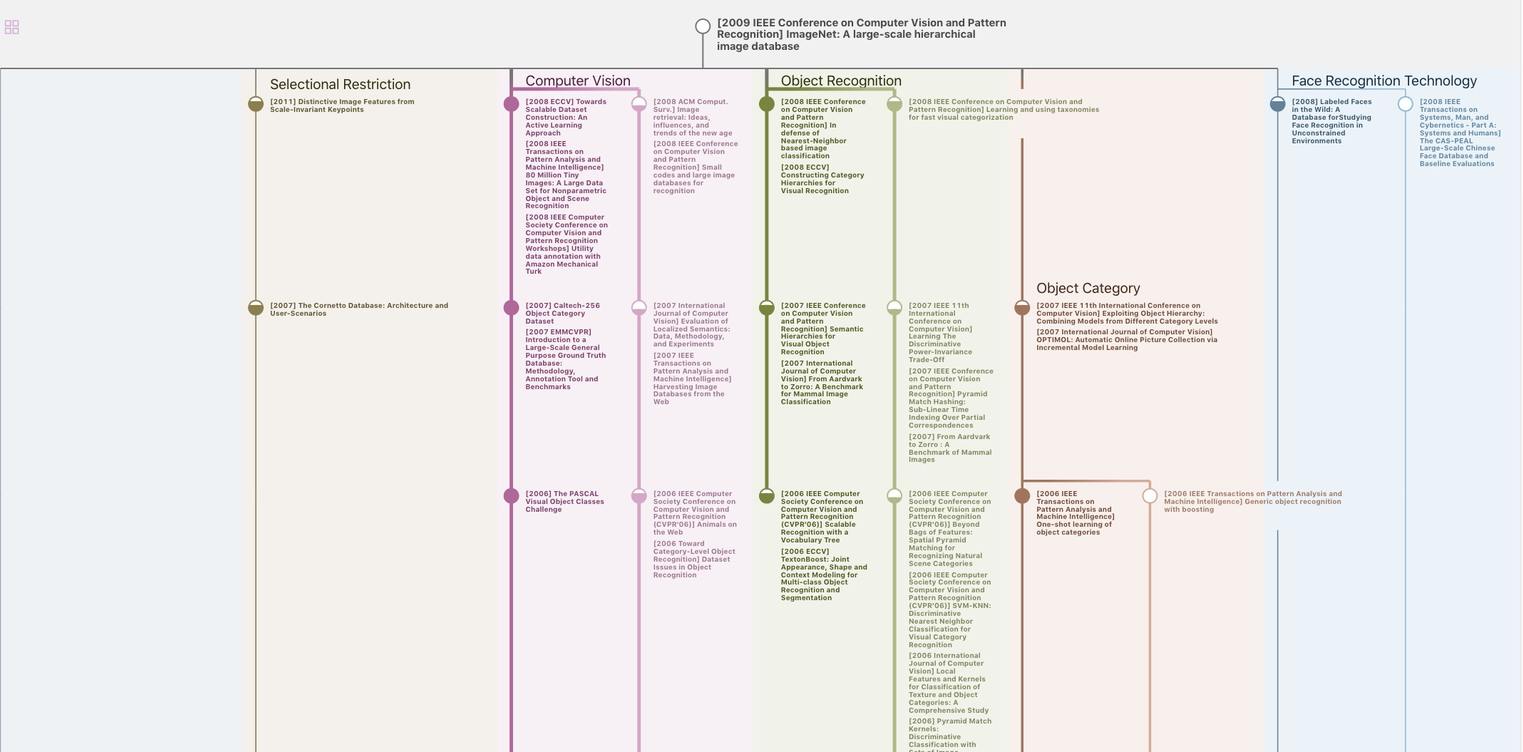
生成溯源树,研究论文发展脉络
Chat Paper
正在生成论文摘要