Brain dynamics and temporal trajectories during task and naturalistic processing
NeuroImage(2019)
摘要
Human functional Magnetic Resonance Imaging (fMRI) data are acquired while participants engage in diverse perceptual, motor, cognitive, and emotional tasks. Although data are acquired temporally, they are most often treated in a quasi-static manner. Yet, a fuller understanding of the mechanisms that support mental functions necessitates the characterization of dynamic properties. Here, we describe an approach employing a class of recurrent neural networks called reservoir computing, and show the feasibility and potential of using it for the analysis of temporal properties of brain data. We show that reservoirs can be used effectively both for condition classification and for characterizing lower-dimensional “trajectories” of temporal data. Classification accuracy was approximately 90% for short clips of “social interactions” and around 70% for clips extracted from movie segments. Data representations with 12 or fewer dimensions (from an original space with over 300) attained classification accuracy within 5% of the full data. We hypothesize that such low-dimensional trajectories may provide “signatures” that can be associated with tasks and/or mental states. The approach was applied across participants (that is, training in one set of participants, and testing in a separate group), showing that representations generalized well to unseen participants. Taken together, we believe the present approach provides a promising framework to characterize dynamic fMRI information during both tasks and naturalistic conditions.
更多查看译文
关键词
Working Memory,Brain-inspired Computing,Neuromorphic Computing,Neural Synchrony,Neural Network Training
AI 理解论文
溯源树
样例
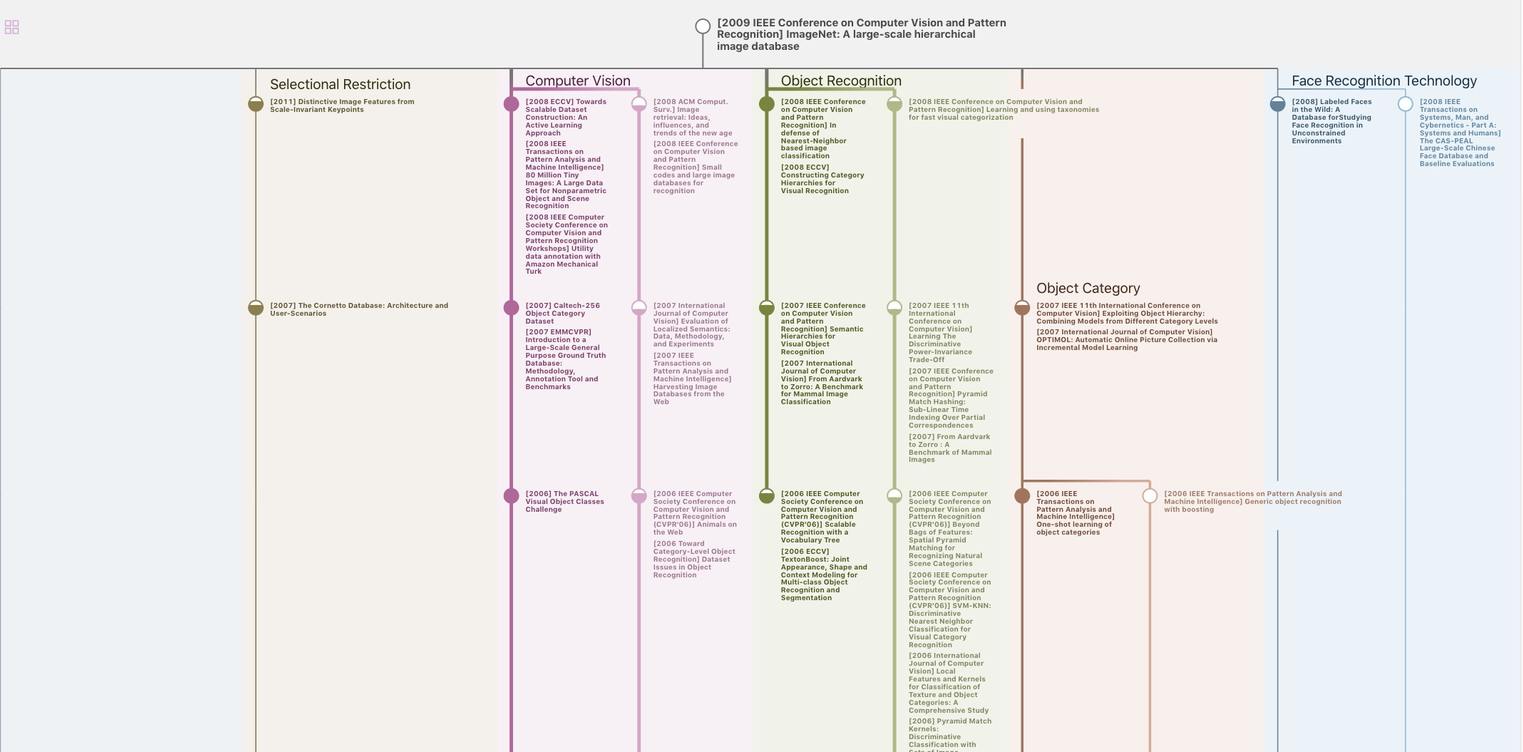
生成溯源树,研究论文发展脉络
Chat Paper
正在生成论文摘要