Footprints of antigen processing boost MHC class II natural ligand predictions
Genome medicine(2018)
摘要
Background Major histocompatibility complex class II (MHC-II) molecules present peptide fragments to T cells for immune recognition. Current predictors for peptide to MHC-II binding are trained on binding affinity data, generated in vitro and therefore lacking information about antigen processing. Methods We generate prediction models of peptide to MHC-II binding trained with naturally eluted ligands derived from mass spectrometry in addition to peptide binding affinity data sets. Results We show that integrated prediction models incorporate identifiable rules of antigen processing. In fact, we observed detectable signals of protease cleavage at defined positions of the ligands. We also hypothesize a role of the length of the terminal ligand protrusions for trimming the peptide to the MHC presented ligand. Conclusions The results of integrating binding affinity and eluted ligand data in a combined model demonstrate improved performance for the prediction of MHC-II ligands and T cell epitopes and foreshadow a new generation of improved peptide to MHC-II prediction tools accounting for the plurality of factors that determine natural presentation of antigens.
更多查看译文
关键词
Antigen processing,Binding predictions,Eluted ligands,MHC-II,Machine learning,Mass spectrometry,Neural networks,T cell epitope
AI 理解论文
溯源树
样例
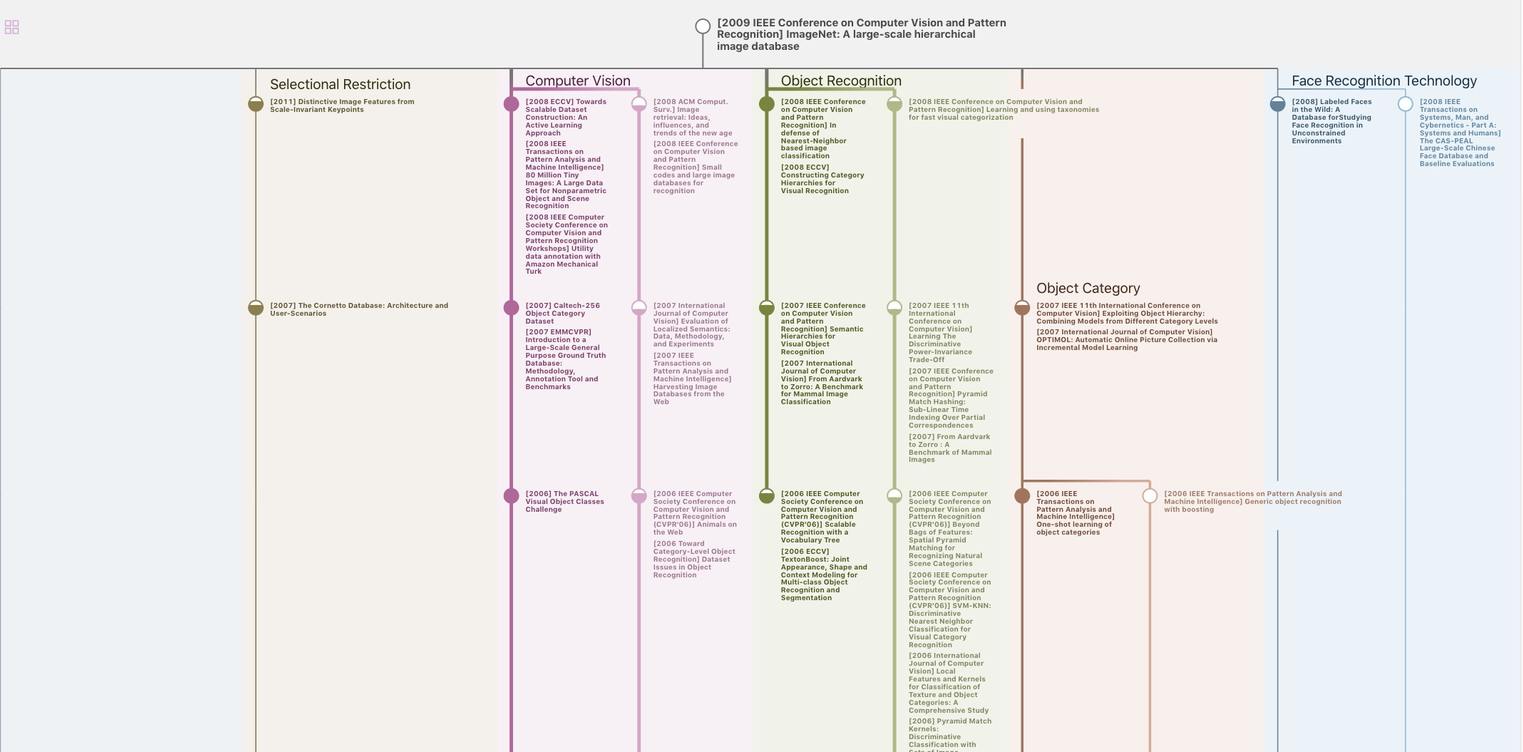
生成溯源树,研究论文发展脉络
Chat Paper
正在生成论文摘要