A machine learning ensemble to predict treatment outcomes following an Internet intervention for depression.
PSYCHOLOGICAL MEDICINE(2019)
摘要
Background. Some Internet interventions are regarded as effective treatments for adult depression, but less is known about who responds to this form of treatment. Method. An elastic net and random forest were trained to predict depression symptoms and related disability after an 8-week course of an Internet intervention, Deprexis, involving adults (N = 283) from across the USA. Candidate predictors included psychopathology, demographics, treatment expectancies, treatment usage, and environmental context obtained from population databases. Model performance was evaluated using predictive R-2 (R-pred(2)), the expected variance explained in a new sample, estimated by 10 repetitions of 10-fold cross-validation. Results. An ensemble model was created by averaging the predictions of the elastic net and random forest. Model performance was compared with a benchmark linear autoregressive model that predicted each outcome using only its baseline. The ensemble predicted more variance in post-treatment depression (8.0% gain, 95% CI 0.8-15; total R-pred(2) = 0.25), disability (5.0% gain, 95% CI -0.3 to 10; total R-pred(2)= 0.25), and well-being (11.6% gain, 95% CI 4.9-19; total R-pred(2)= 0.29) than the benchmark model. Important predictors included comorbid psychopathology, particularly total psychopathology and dysthymia, low symptom-related disability, treatment credibility, lower access to therapists, and time spent using certain Deprexis modules. Conclusion. A number of variables predict symptom improvement following an Internet intervention, but each of these variables makes relatively small contributions. Machine learning ensembles may be a promising statistical approach for identifying the cumulative contribution of many weak predictors to psychosocial depression treatment response.
更多查看译文
关键词
Depression treatment,Internet interventions,machine learning
AI 理解论文
溯源树
样例
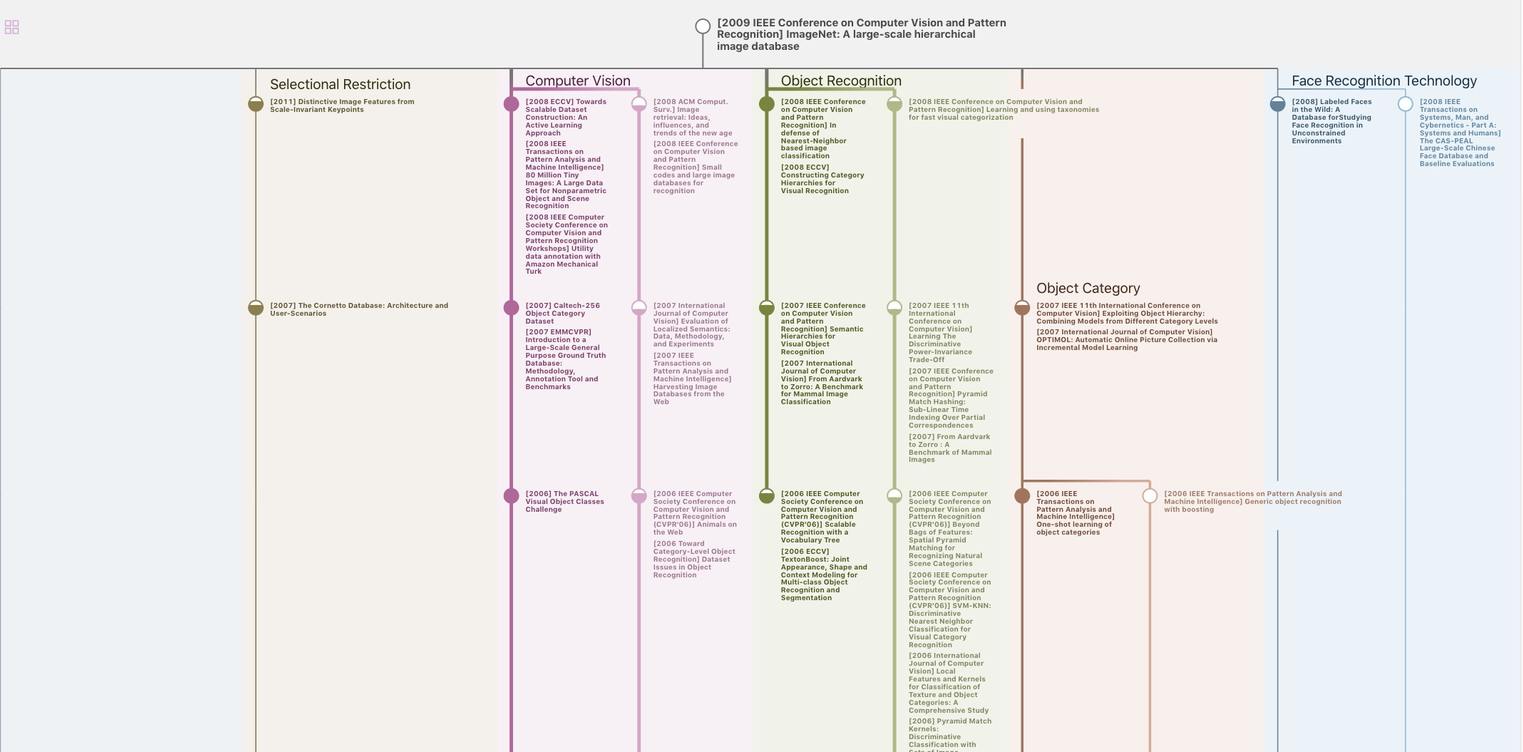
生成溯源树,研究论文发展脉络
Chat Paper
正在生成论文摘要