Recent advances in machine learning methods for predicting heat shock proteins.
CURRENT DRUG METABOLISM(2019)
摘要
Background: As molecular chaperones, Heat Shock Proteins (HSPs) not only play key roles in protein folding and maintaining protein stabilities, but are also linked with multiple kinds of diseases. Therefore, HSPs have been regarded as the focus of drug design. Since HSPs from different families play distinct functions, accurately classifying the families of HSPs is the key step to clearly understand their biological functions. In contrast to labor-intensive and cost-ineffective experimental methods, computational classification of HSP families has emerged to be an alternative approach. Methods: We reviewed the paper that described the existing datasets of HSPs and the representative computational approaches developed for the identification and classification of HSPs. Results: The two benchmark datasets of HSPs, namely HSPIR and sHSPdb were introduced, which provided invaluable resources for computationally identifying HSPs. The gold standard dataset and sequence encoding schemes for building computational methods of classifying HSPs were also introduced. The three representative web-servers for identifying HSPs and their families were described. Conclusion: The existing machine learning methods for identifying the different families of HSPs indeed yielded quite encouraging results and did play a role in promoting the research on HSPs. However, the number of HSPs with known structures is very limited. Therefore, determining the structure of the HSPs is also urgent, which will be helpful in revealing their functions.
更多查看译文
关键词
Heat shock protein,n-peptide composition,reduced amino acid composition,machine learning,drug target,web server
AI 理解论文
溯源树
样例
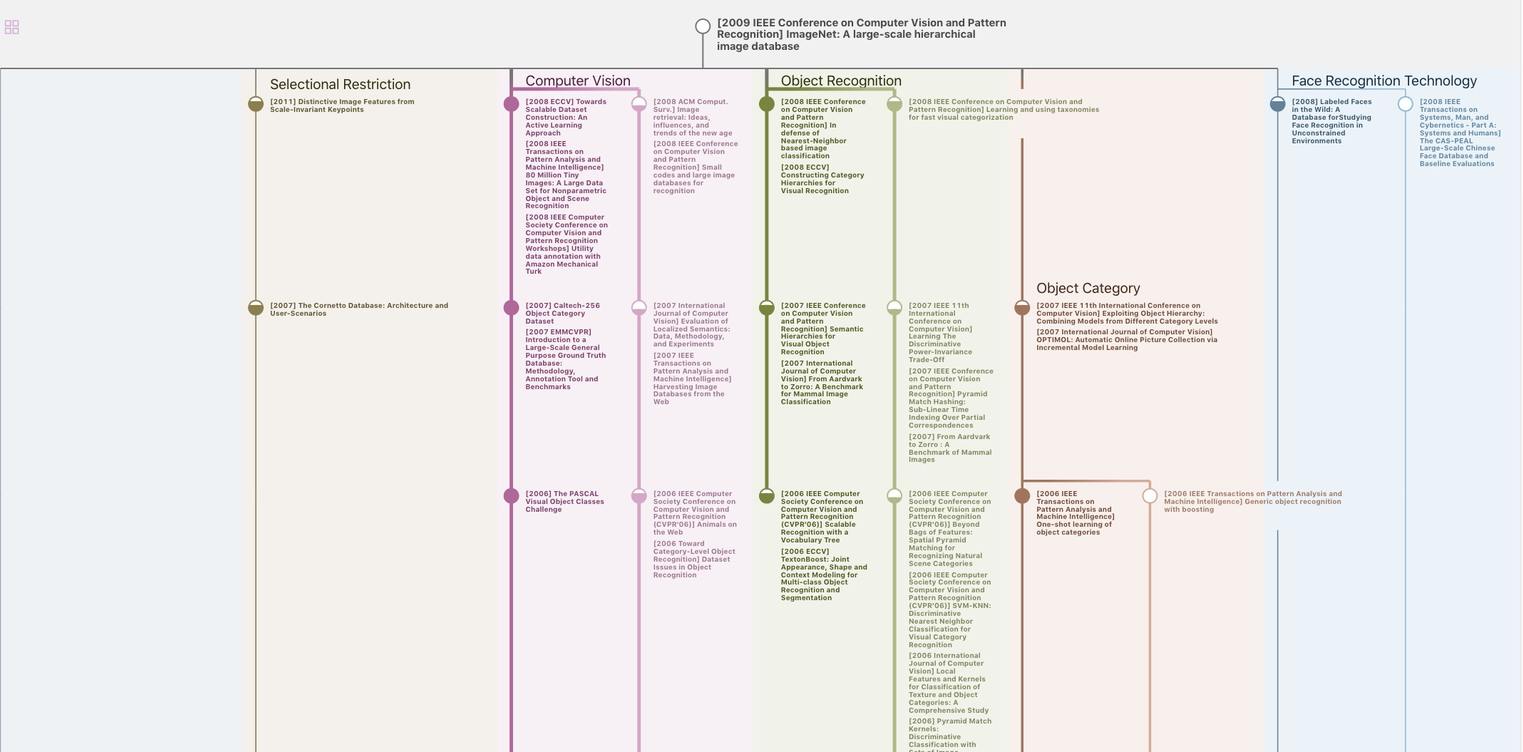
生成溯源树,研究论文发展脉络
Chat Paper
正在生成论文摘要