Machine Learning in Quantitative Protein-Peptide Affinity Prediction: Implications for Therapeutic Peptide Design.
CURRENT DRUG METABOLISM(2019)
摘要
Background: Protein-peptide recognition plays an essential role in the orchestration and regulation of cell signaling networks, which is estimated to be responsible for up to 40% of biological interaction events in the human interactome and has recently been recognized as a new and attractive druggable target for drug development and disease intervention. Methods: We present a systematic review on the application of machine learning techniques in the quantitative modeling and prediction of protein-peptide binding affinity, particularly focusing on its implications for therapeutic peptide design. We also briefly introduce the physical quantities used to characterize protein-peptide affinity and attempt to extend the content of generalized machine learning methods. Results: Existing issues and future perspective on the statistical modeling and regression prediction of protein-peptide binding affinity are discussed. Conclusion: There is still a long way to go before establishment of general, reliable and efficient machine leaning-based protein-peptide affinity predictors.
更多查看译文
关键词
Protein-peptide affinity,therapeutic peptide design,machine learning,statistical regression,druggable target,molecular recognition,computational peptidology
AI 理解论文
溯源树
样例
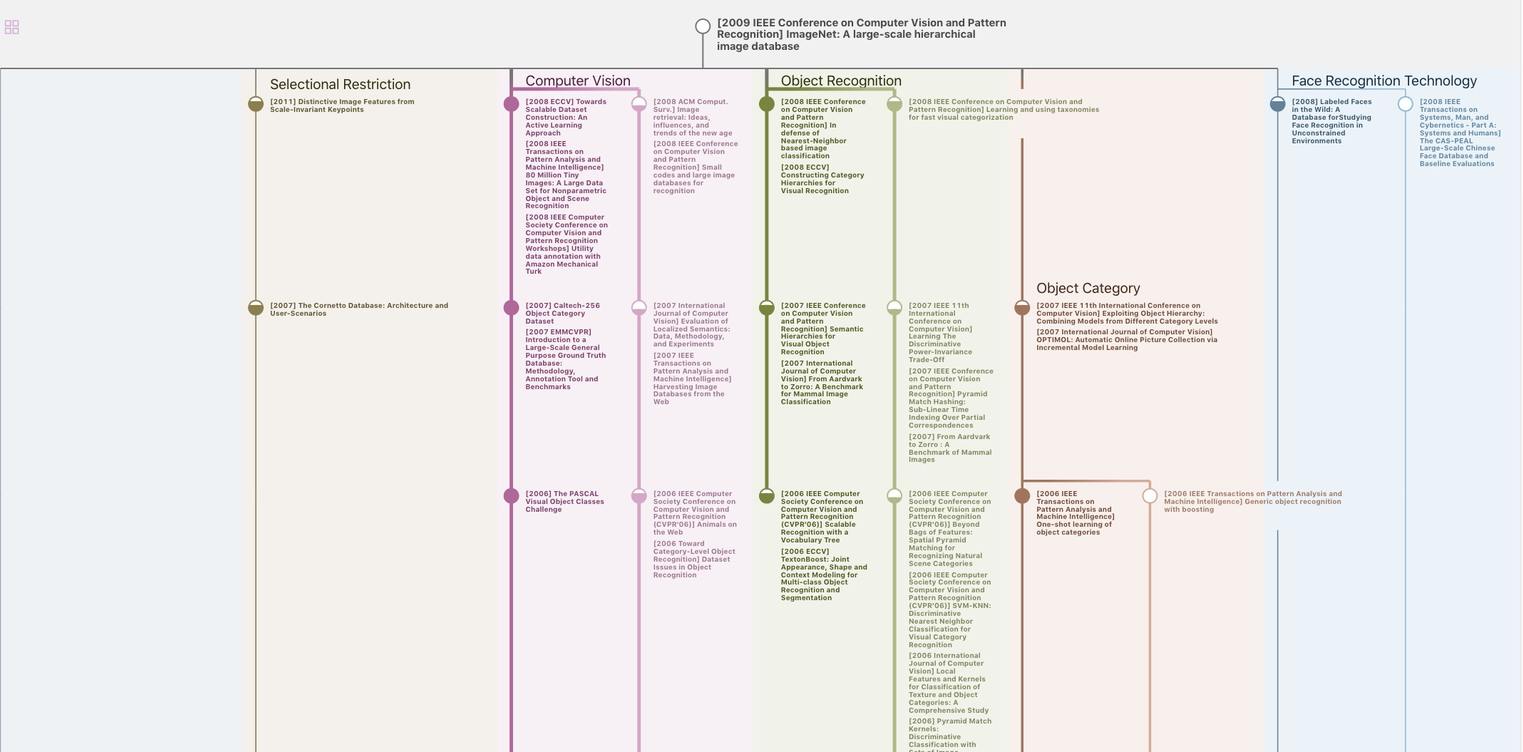
生成溯源树,研究论文发展脉络
Chat Paper
正在生成论文摘要