Covariate selection strategies for causal inference: Classification and comparison.
BIOMETRICAL JOURNAL(2018)
摘要
When causal effects are to be estimated from observational data, we have to adjust for confounding. A central aim of covariate selection for causal inference is therefore to determine a set that is sufficient for confounding adjustment, but other aims such as efficiency or robustness can be important as well. In this paper, we review six general approaches to covariate selection that differ in the targeted type of adjustment set. We discuss and illustrate their advantages and disadvantages using causal diagrams. Moreover, the approaches and different ways of implementing them are compared empirically in an extensive simulation study. We conclude that there are considerable differences between the approaches but none of them is uniformly best, with performance depending on the chosen adjustment method as well as the true confounding structure. Any prior structural knowledge on the causal relations is helpful to choose the most appropriate method.
更多查看译文
关键词
average causal effect,causal diagram,confounder selection,propensity score matching,variable selection
AI 理解论文
溯源树
样例
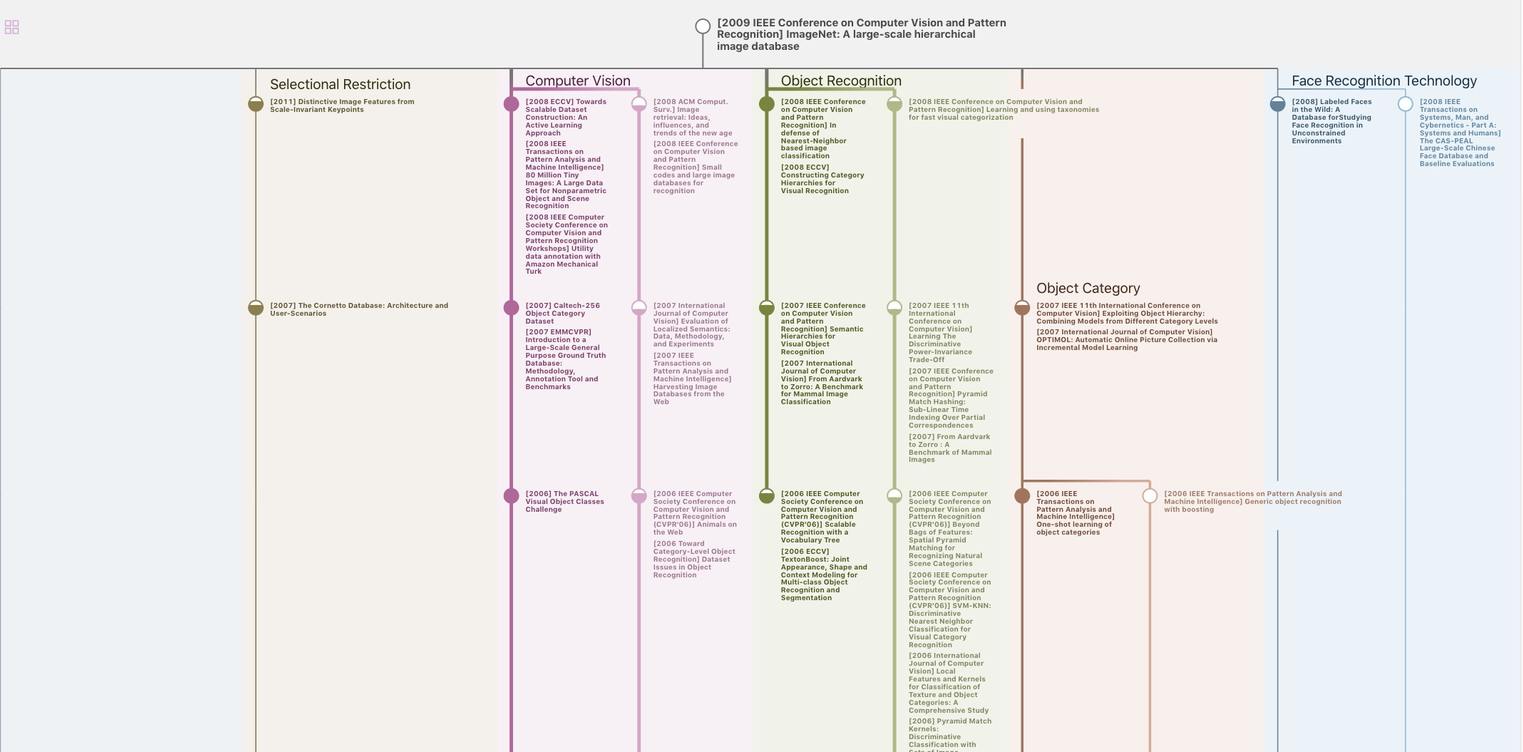
生成溯源树,研究论文发展脉络
Chat Paper
正在生成论文摘要