Machine-learning based lipid mediator serum concentration patterns allow identification of multiple sclerosis patients with high accuracy
SCIENTIFIC REPORTS(2018)
摘要
Based on increasing evidence suggesting that MS pathology involves alterations in bioactive lipid metabolism, the present analysis was aimed at generating a complex serum lipid-biomarker. Using unsupervised machine-learning, implemented as emergent self-organizing maps of neuronal networks, swarm intelligence and Minimum Curvilinear Embedding, a cluster structure was found in the input data space comprising serum concentrations of d = 43 different lipid-markers of various classes. The structure coincided largely with the clinical diagnosis, indicating that the data provide a basis for the creation of a biomarker (classifier). This was subsequently assessed using supervised machine-learning, implemented as random forests and computed ABC analysis-based feature selection. Bayesian statistics-based biomarker creation was used to map the diagnostic classes of either MS patients (n = 102) or healthy subjects (n = 301). Eight lipid-markers passed the feature selection and comprised GluCerC16, LPA20:4, HETE15S, LacCerC24:1, C16Sphinganine, biopterin and the endocannabinoids PEA and OEA. A complex classifier or biomarker was developed that predicted MS at a sensitivity, specificity and accuracy of approximately 95% in training and test data sets, respectively. The present successful application of serum lipid marker concentrations to MS data is encouraging for further efforts to establish an MS biomarker based on serum lipidomics.
更多查看译文
关键词
Lipid Markers,Random Forest,Endocannabinoid,Emergent Self-organizing Maps,Relapsing Remitting MS (RRMS)
AI 理解论文
溯源树
样例
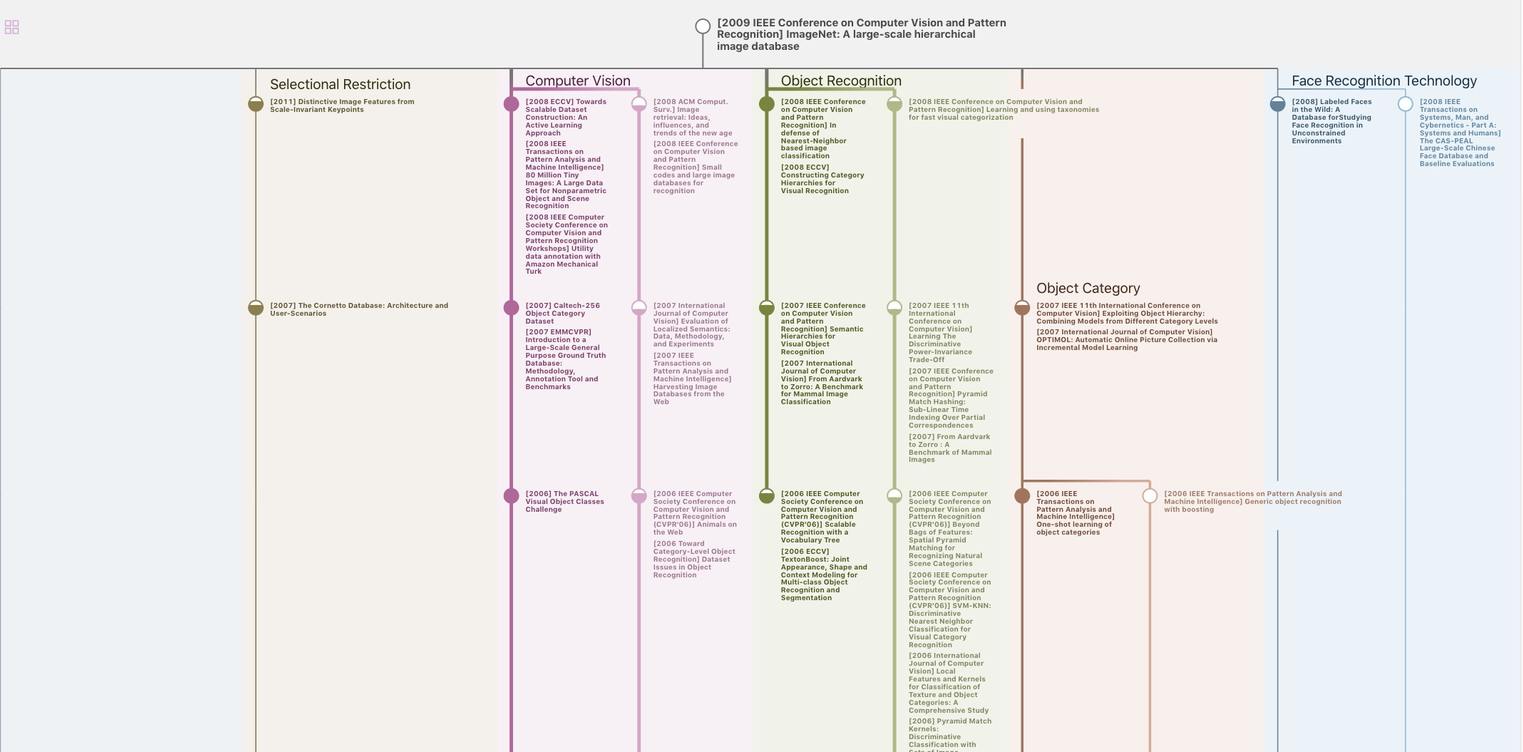
生成溯源树,研究论文发展脉络
Chat Paper
正在生成论文摘要