Self-Consistent Models and Values.
Annual Conference on Neural Information Processing Systems(2021)
摘要
Learned models of the environment provide reinforcement learning (RL) agents with flexible ways of making predictions about the environment.Models enable planning, i.e. using more computation to improve value functions or policies, without requiring additional environment interactions.In this work, we investigate a way of augmenting model-based RL, by additionally encouraging a learned model and value function to be jointly \emph{self-consistent}.This lies in contrast to classic planning methods like Dyna, which only update the value function to be consistent with the model.We propose a number of possible self-consistency updates, study them empirically in both the tabular and function approximation settings, and find that with appropriate choices self-consistency can be useful both for policy evaluation and control.
更多查看译文
关键词
models,values,self-consistent
AI 理解论文
溯源树
样例
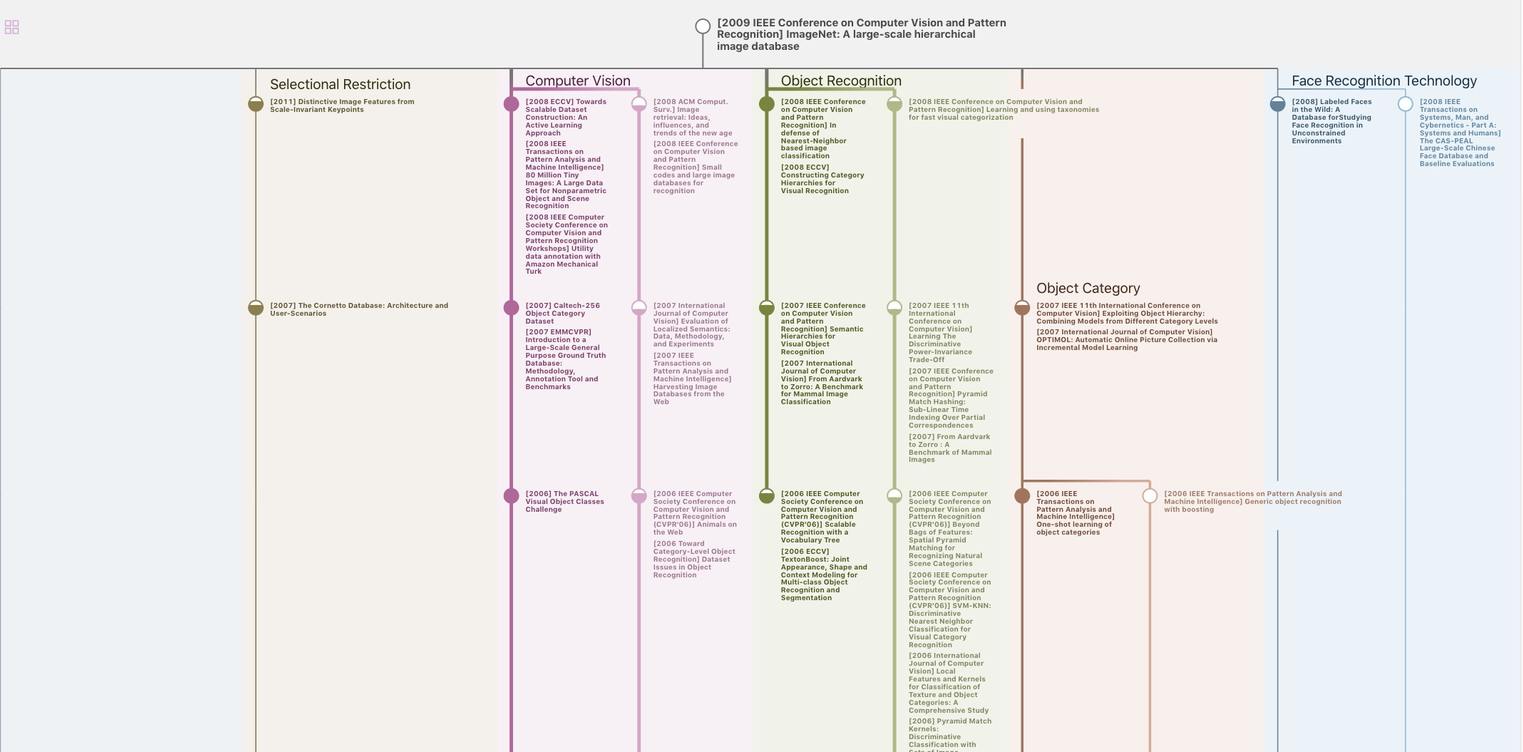
生成溯源树,研究论文发展脉络
Chat Paper
正在生成论文摘要