High-Performance Deep Neural Network-Based Tomato Plant Diseases and Pests Diagnosis System With Refinement Filter Bank.
FRONTIERS IN PLANT SCIENCE(2018)
摘要
A fundamental problem that confronts deep neural networks is the requirement of a large amount of data for a system to be efficient in complex applications. Promising results of this problemaremade possible through the use of techniques such as data augmentation or transfer learning of pre-trained models in large datasets. But the problem still persists when the application provides limited or unbalanced data. In addition, the number of false positives resulting from training a deep model significantly cause a negative impact on the performance of the system. This study aims to address the problem of false positives and class unbalance by implementing a Refinement Filter Bank framework for Tomato Plant Diseases and Pests Recognition. The system consists of three main units: First, a Primary Diagnosis Unit (Bounding Box Generator) generates the bounding boxes that contain the location of the infected area and class. The promising boxes belonging to each class are then used as input to a Secondary Diagnosis Unit (CNN Filter Bank) for verification. In this second unit, misclassified samples are filtered through the training of independent CNN classifiers for each class. The result of the CNN Filter Bank is a decision of whether a target belongs to the category as it was detected (True) or not (False) otherwise. Finally, an integration unit combines the information from the primary and secondary units while keeping the True Positive samples and eliminating the False Positives that were misclassified in the first unit. By this implementation, the proposed approach is able to obtain a recognition rate of approximately 96%, which represents an improvement of 13% compared to our previous work in the complex task of tomato diseases and pest recognition. Furthermore, our system is able to deal with the false positives generated by the bounding box generator, and class unbalances that appear especially on datasets with limited data.
更多查看译文
关键词
plant diseases,detection,deep neural networks,filter banks,false positives
AI 理解论文
溯源树
样例
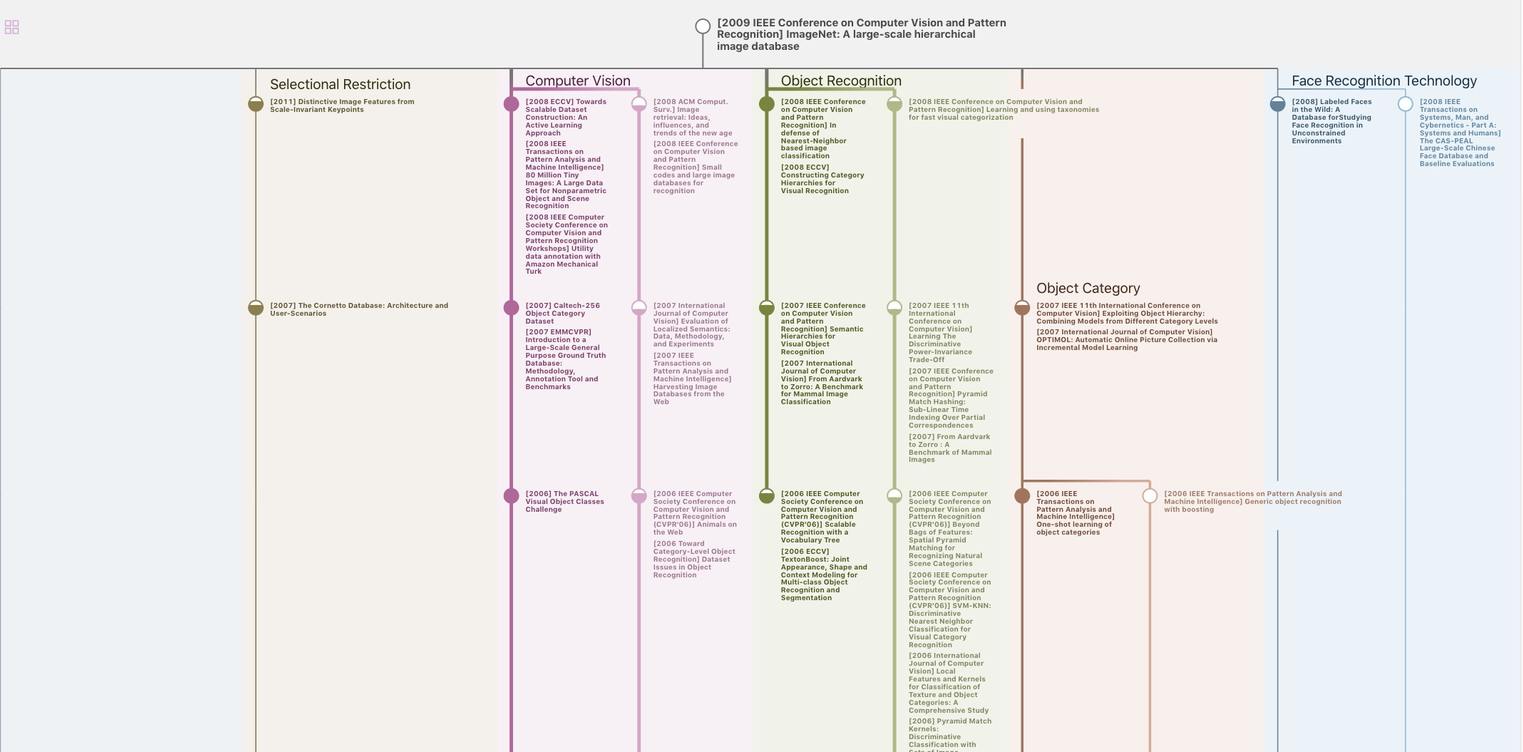
生成溯源树,研究论文发展脉络
Chat Paper
正在生成论文摘要