Adaptive Learning Control for Nonlinear Systems With Randomly Varying Iteration Lengths.
IEEE transactions on neural networks and learning systems(2019)
摘要
This paper proposes adaptive iterative learning control (ILC) schemes for continuous-time parametric nonlinear systems with iteration lengths that randomly vary. As opposed to the existing ILC works that feature nonuniform trial lengths, this paper is applicable to nonlinear systems that do not satisfy the globally Lipschitz continuous condition. In addition, this paper introduces a novel composite energy function based on newly defined virtual tracking error information for proving the asymptotical convergence. Both an original update algorithm and a projection-based update algorithm for estimating the unknown parameters are proposed. Extensions to cases with unknown input gains, iteration-varying tracking references, nonparametric uncertainty, high-order nonlinear systems, and multi-input-multi-output systems are all elaborated upon. Illustrative simulations are provided to verify the theoretical results.
更多查看译文
关键词
Convergence,Nonlinear systems,Control systems,Linear systems,Robots,Stochastic processes,Uncertainty
AI 理解论文
溯源树
样例
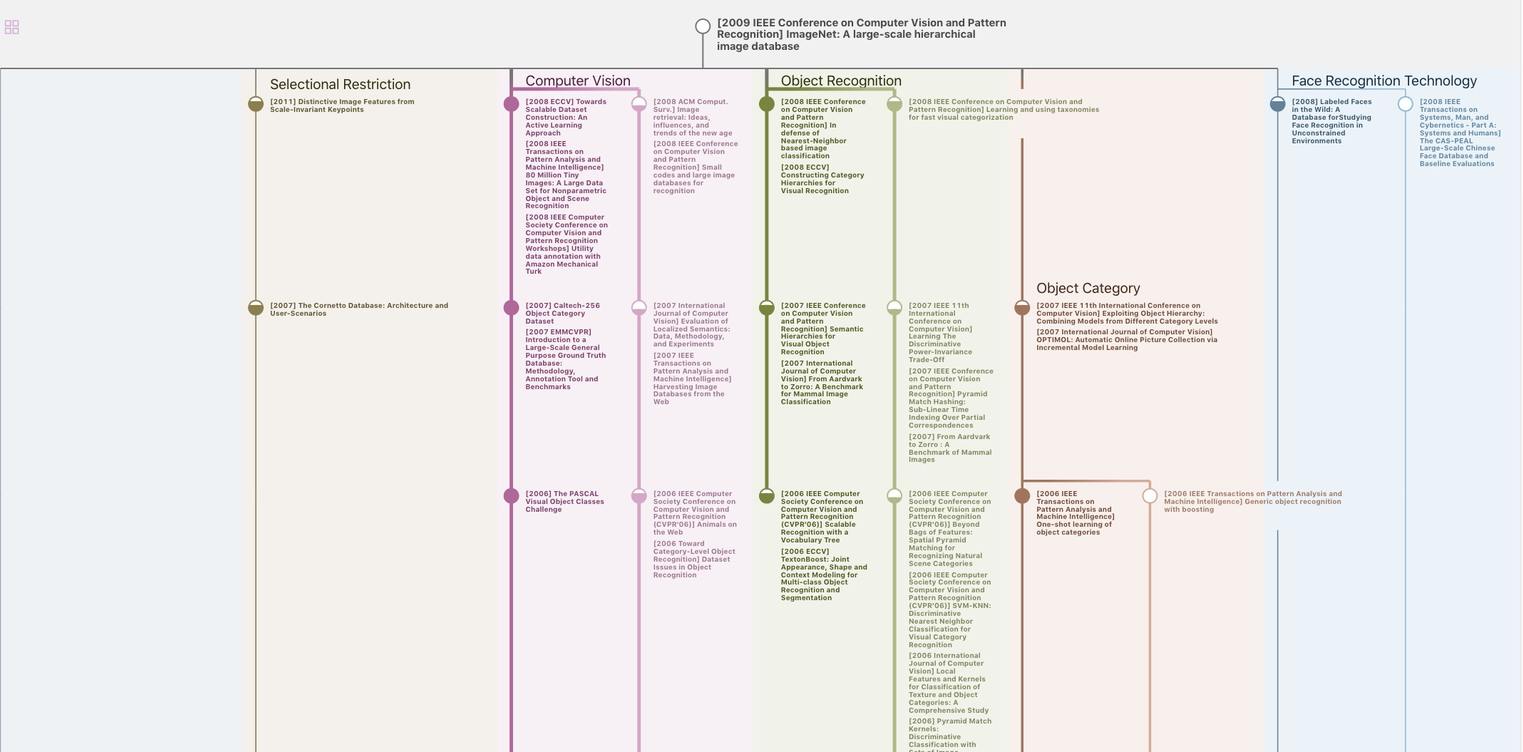
生成溯源树,研究论文发展脉络
Chat Paper
正在生成论文摘要