Unsupervised Learning for Detection of Defects in Pulsed Infrared Thermography of Metals
2022 IEEE International Conference on Electro Information Technology (eIT)(2022)
摘要
Metal Additive Manufacturing (AM) is a promising method for cost-efficient fabrication of complex shape structures for applications in harsh environment, such as in a nuclear reactor. However, internal defects (pores) occur in high-strength AM alloys, which are manufactured with Laser Powder Bed Fusion (LPBF) AM method. Pulsed Infrared Thermography (PIT) is an efficient nondestructive evaluation (NDE) method to examine actual structures, because this method offers one-sided non-contact measurements, and fast processing of large sample areas. However, imaging of material defects, particularly defects with sizes at microscopic level, is challenging. In this paper, we benchmark the performance of several Unsupervised Learning (UL) algorithms designed to enhance imaging of microscopic defects in metals with PIT. UL aims to learn the latent principal patterns (dictionaries) in PIT data to detect defects with minimal human supervision. Performance of Independent Component Analysis (ICA), Sparse Coding (SC), Principal Component Analysis (PCA) and Exploratory Factor Analysis (EFA) was compared using F-score, UL model training time and defects reconstruction time. We obtained the average F-score of 0.75, and a highest F-score of 0.89 for the EFA algorithm. Overall, EFA outperforms other UL algorithms considered in this study.
更多查看译文
关键词
Unsupervised Learning,Metallic Microscopic Defects Detection,Pulsed Infrared Thermography,Metal Additively Manufacturing
AI 理解论文
溯源树
样例
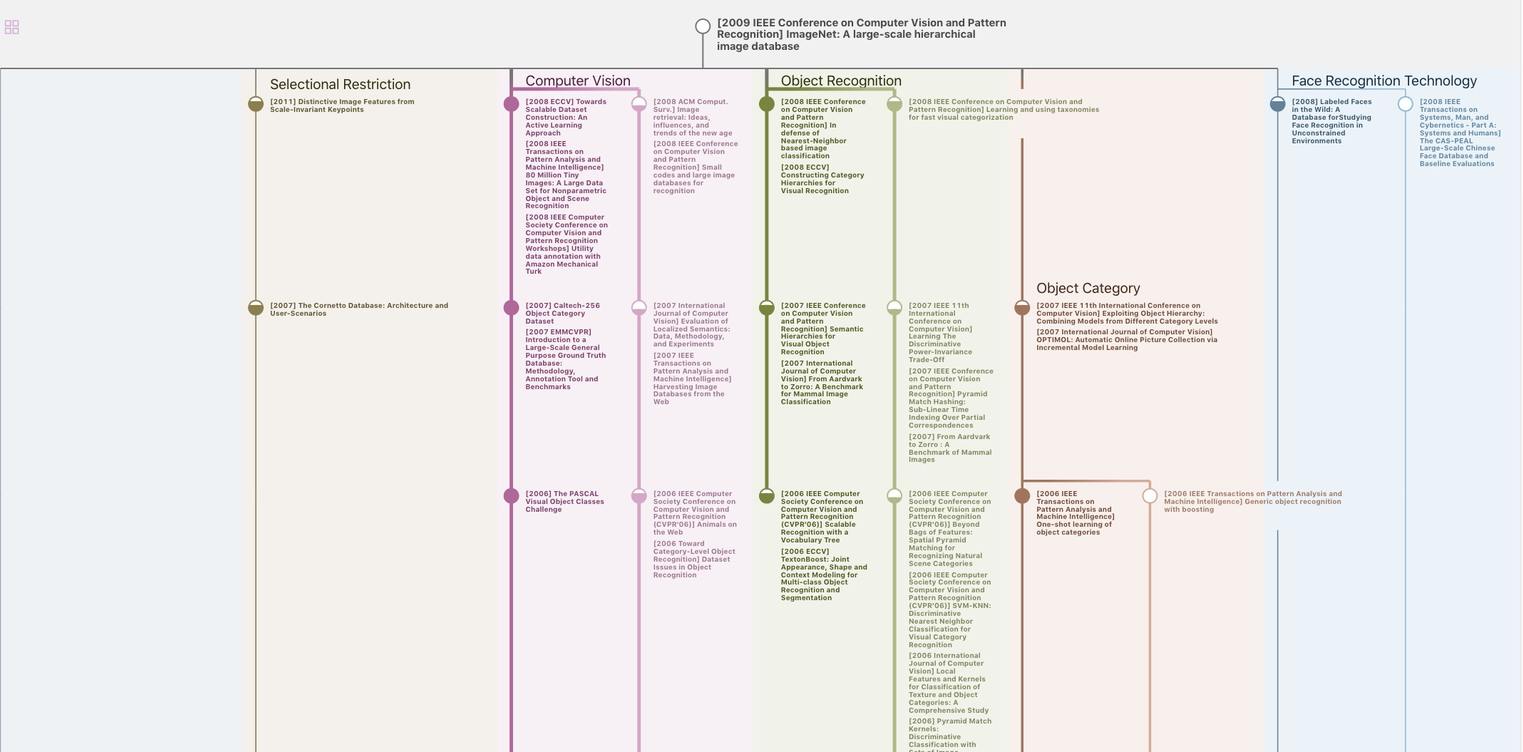
生成溯源树,研究论文发展脉络
Chat Paper
正在生成论文摘要