Semisupervised Regression With Optimized Rank for Matrix Data Classification.
IEEE transactions on cybernetics(2019)
摘要
There has been growing interest in developing more effective algorithms for matrix data classification. At present, most of the existing vector-based classifications involve vectorization process, which results in two main problems. First, the underlying structural information is disregarded. Second, the vectorization of a matrix incurs the creation of a vector with potentially very high dimensionality, which may lead to over-fitting when the number of training data is small. To avoid such problems, we propose a new matrix-based regression algorithm for classification, in which the input matrices to be classified are directly used to learn two regression matrices for each order of the input matrix. To further explore the discrimination information, we add a joint ℓ2,1-norm on two regression matrices, which endows the algorithm optimized regression rank by uncovering common sparse columns in the two regression matrices. To further boost the classification performance, we incorporate a semisupervised learning process, which leverages both labeled and unlabeled data to enhance the training process. Experiments on public benchmark datasets show that our method outperforms a number of the existing state-of-the-art classification methods even when only few labeled training samples are provided.
更多查看译文
关键词
Tensile stress,Sparse matrices,Matrix decomposition,Covariance matrices,Support vector machines,Training,Data visualization
AI 理解论文
溯源树
样例
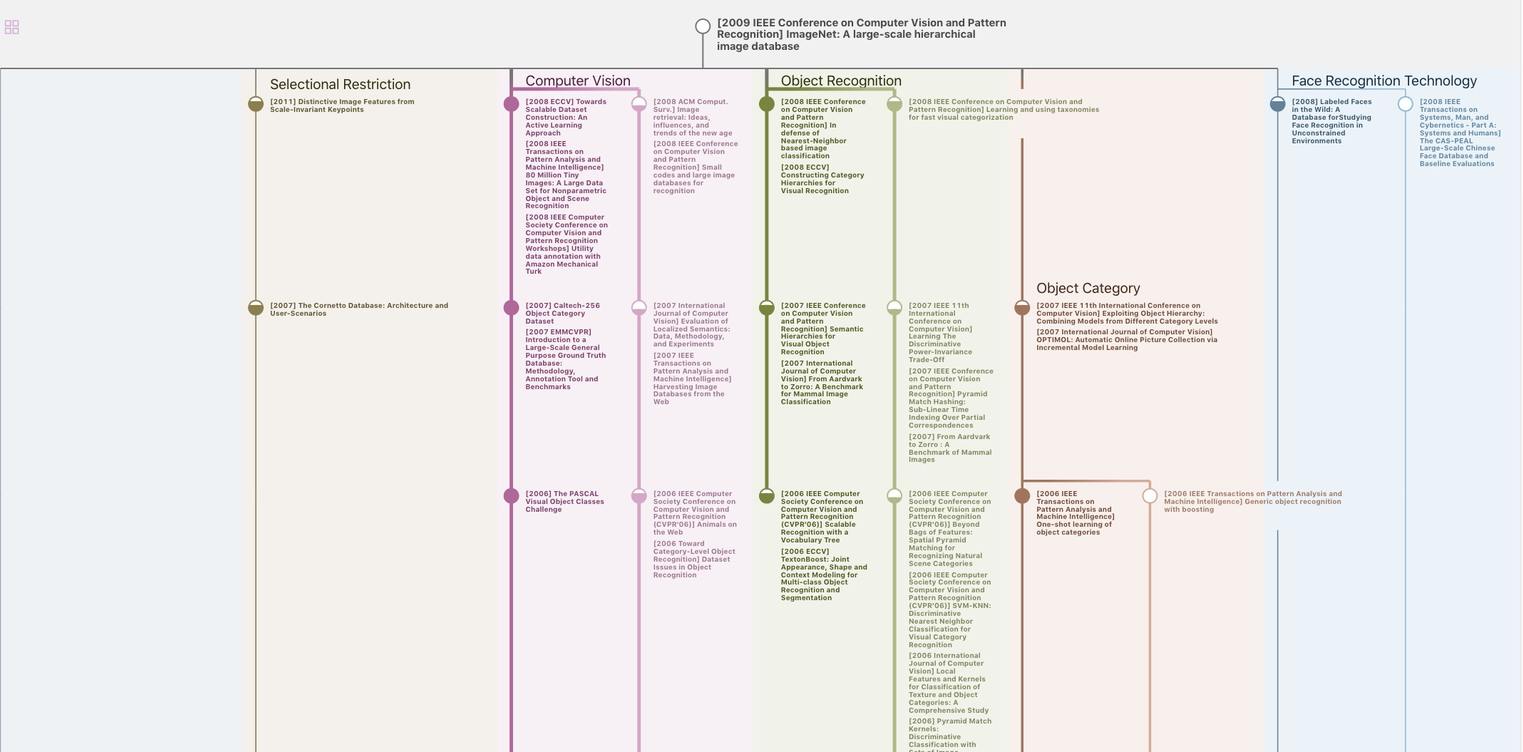
生成溯源树,研究论文发展脉络
Chat Paper
正在生成论文摘要