Inverse Biomechanical Modeling of the Tongue via Machine Learning and Synthetic Training Data.
Proceedings of SPIE(2018)
摘要
The tongue's deformation during speech can be measured using tagged magnetic resonance imaging, but there is no current method to directly measure the pattern of muscles that activate to produce a given motion. In this paper, the activation pattern of the tongue's muscles is estimated by solving an inverse problem using a random forest. Examples describing different activation patterns and the resulting deformations are generated using a finite-element model of the tongue. These examples form training data for a random forest comprising 30 decision trees to estimate contractions in 262 contractile elements. The method was evaluated on data from tagged magnetic resonance data from actual speech and on simulated data mimicking flaps that might have resulted from glossectomy surgery. The estimation accuracy was modest (5.6% error), but it surpassed a semi-manual approach (8.1% error). The results suggest that a machine learning approach to contraction pattern estimation in the tongue is feasible, even in the presence of flaps.
更多查看译文
关键词
Machine-Learning,Model Inversion,Biomechanics,Random Forest
AI 理解论文
溯源树
样例
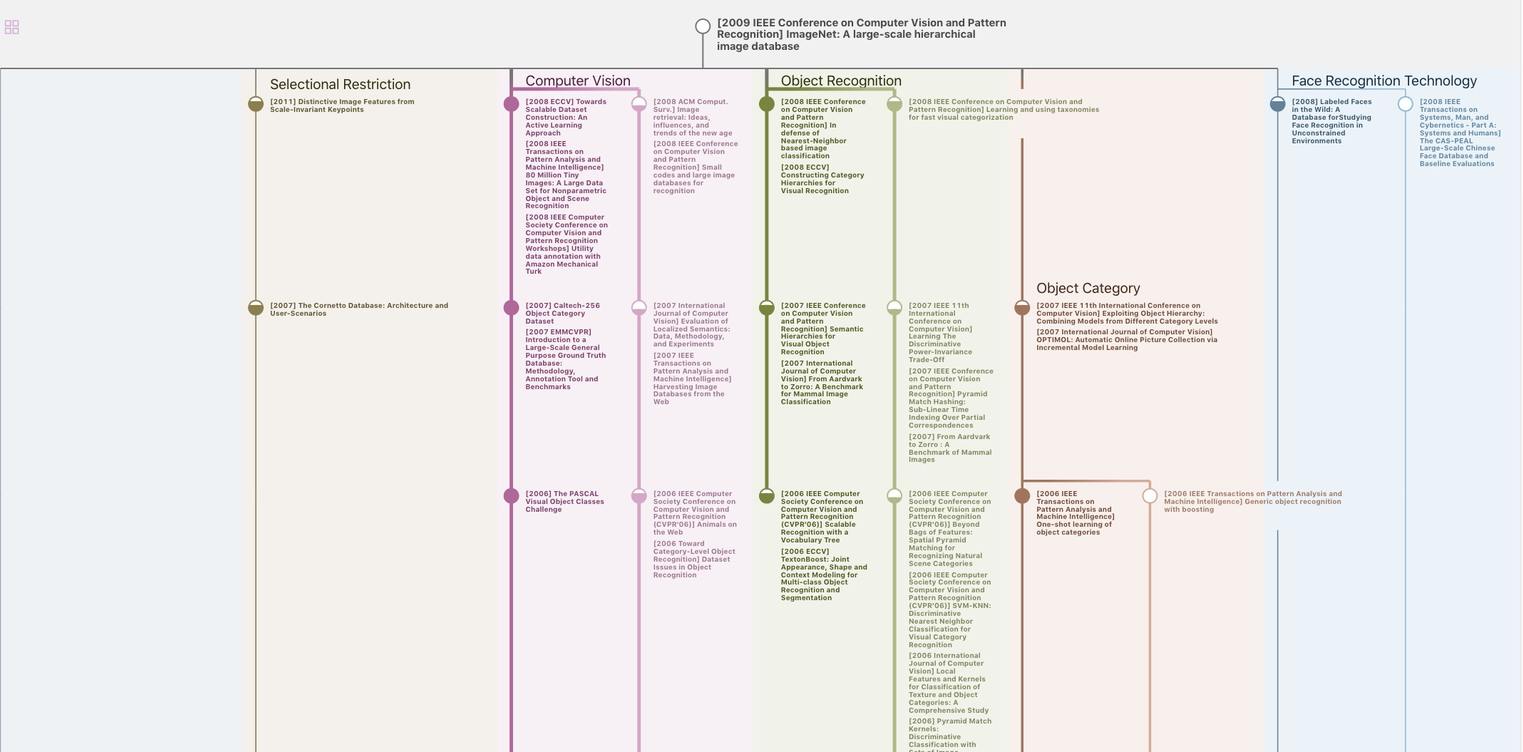
生成溯源树,研究论文发展脉络
Chat Paper
正在生成论文摘要