Multimodal Sentiment Analysis With Image-Text Interaction Network.
IEEE Trans. Multim.(2023)
Abstract
More and more users are getting used to posting images and text on social networks to share their emotions or opinions. Accordingly, multimodal sentiment analysis has become a research topic of increasing interest in recent years. Typically, there exist affective regions that evoke human sentiment in an image, which are usually manifested by corresponding words in people's comments. Similarly, people also tend to portray the affective regions of an image when composing image descriptions. As a result, the relationship between image affective regions and the associated text is of great significance for multimodal sentiment analysis. However, most of the existing multimodal sentiment analysis approaches simply concatenate features from image and text, which could not fully explore the interaction between them, leading to suboptimal results. Motivated by this observation, we propose a new image-text interaction network (ITIN) to investigate the relationship between affective image regions and text for multimodal sentiment analysis. Specifically, we introduce a cross-modal alignment module to capture region-word correspondence, based on which multimodal features are fused through an adaptive cross-modal gating module. Moreover, considering the complementary role of context information on sentiment analysis, we integrate the individual-modal contextual feature representations for achieving more reliable prediction. Extensive experimental results and comparisons on public datasets demonstrate that the proposed model is superior to the state-of-the-art methods.
MoreTranslated text
AI Read Science
Must-Reading Tree
Example
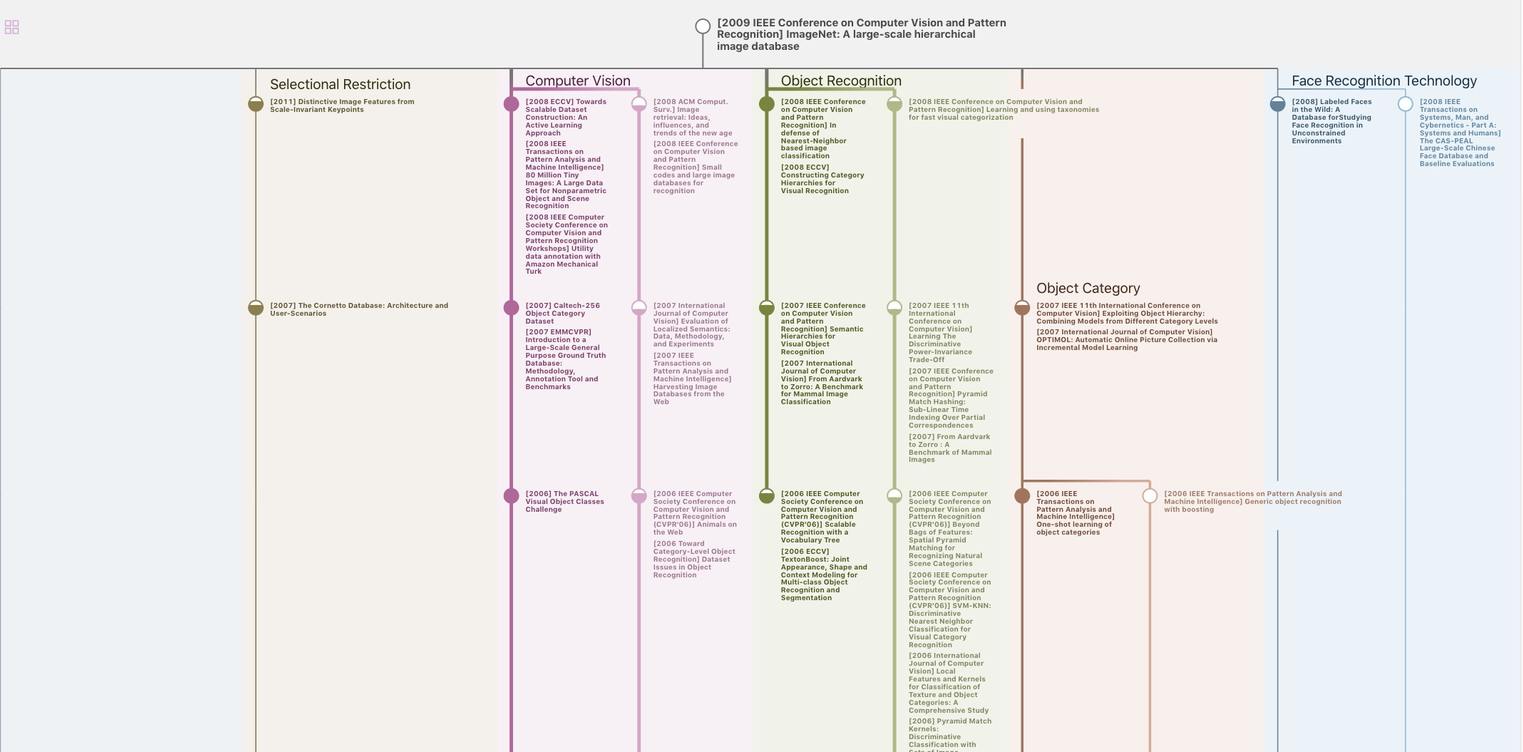
Generate MRT to find the research sequence of this paper
Chat Paper
Summary is being generated by the instructions you defined