Machine Learning Classification Models To Improve The Docking-Based Screening: A Case Of Pi3k-Tankyrase Inhibitors
MOLECULAR INFORMATICS(2018)
Abstract
One of the major challenges in the current drug discovery is the improvement of the docking-based virtual screening performance. It is especially important in the rational design of compounds with desired polypharmacology or selectivity profiles. To address this problem, we present a methodology for the development of target-specific scoring functions possessing high screening power. These scoring functions were built using the machine learning methods for the dual target inhibitors of PI3K alpha and tankyrase, promising targets for colorectal cancer therapy. The Deep Neural Network models achieve the external test AUC ROC values of 0.96 and 0.93 for the random split and 0.90 and 0.84 for the time-based split of the PI3K alpha and tankyrase inhibitors, respectively. In addition, the impact of the training set size and the actives/decoys ratio on the model quality was assessed. The study demonstrates that the optimized scoring functions could significantly improve the docking screening power for each individual target. This is very useful in the design of multitarget or selective drugs wherein the screening filters are applied in sequence.
MoreTranslated text
Key words
Docking,drug discovery,machine learning,virtual screening
AI Read Science
Must-Reading Tree
Example
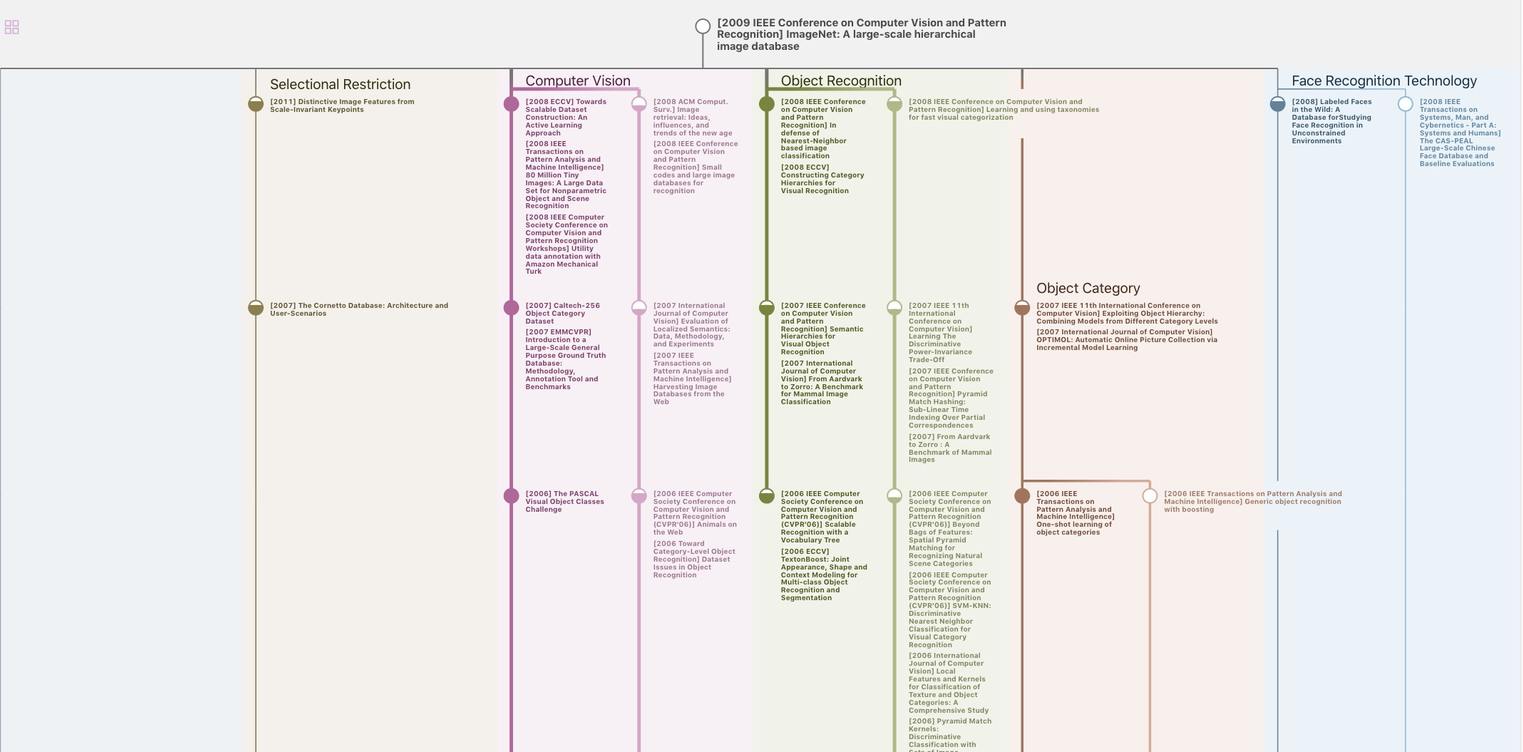
Generate MRT to find the research sequence of this paper
Chat Paper
Summary is being generated by the instructions you defined