Automatic quantification framework to detect cracks in teeth.
Proceedings of SPIE(2018)
摘要
Studies show that cracked teeth are the third most common cause for tooth loss in industrialized countries. If detected early and accurately, patients can retain their teeth for a longer time. Most cracks are not detected early because of the discontinuous symptoms and lack of good diagnostic tools. Currently used imaging modalities like Cone Beam Computed Tomography (CBCT) and intraoral radiography often have low sensitivity and do not show cracks clearly. This paper introduces a novel method that can detect, quantify, and localize cracks automatically in high resolution CBCT (hr-CBCT) scans of teeth using steerable wavelets and learning methods. These initial results were created using hr-CBCT scans of a set of healthy teeth and of teeth with simulated longitudinal cracks. The cracks were simulated using multiple orientations. The crack detection was trained on the most significant wavelet coefficients at each scale using a bagged classifier of Support Vector Machines. Our results show high discriminative specificity and sensitivity of this method. The framework aims to be automatic, reproducible, and open-source. Future work will focus on the clinical validation of the proposed techniques on different types of cracks ex-vivo. We believe that this work will ultimately lead to improved tracking and detection of cracks allowing for longer lasting healthy teeth.
更多查看译文
关键词
Wavelet analysis,Tooth fracture detection,Machine learning,High-resolution Cone Beam Computed Tomography
AI 理解论文
溯源树
样例
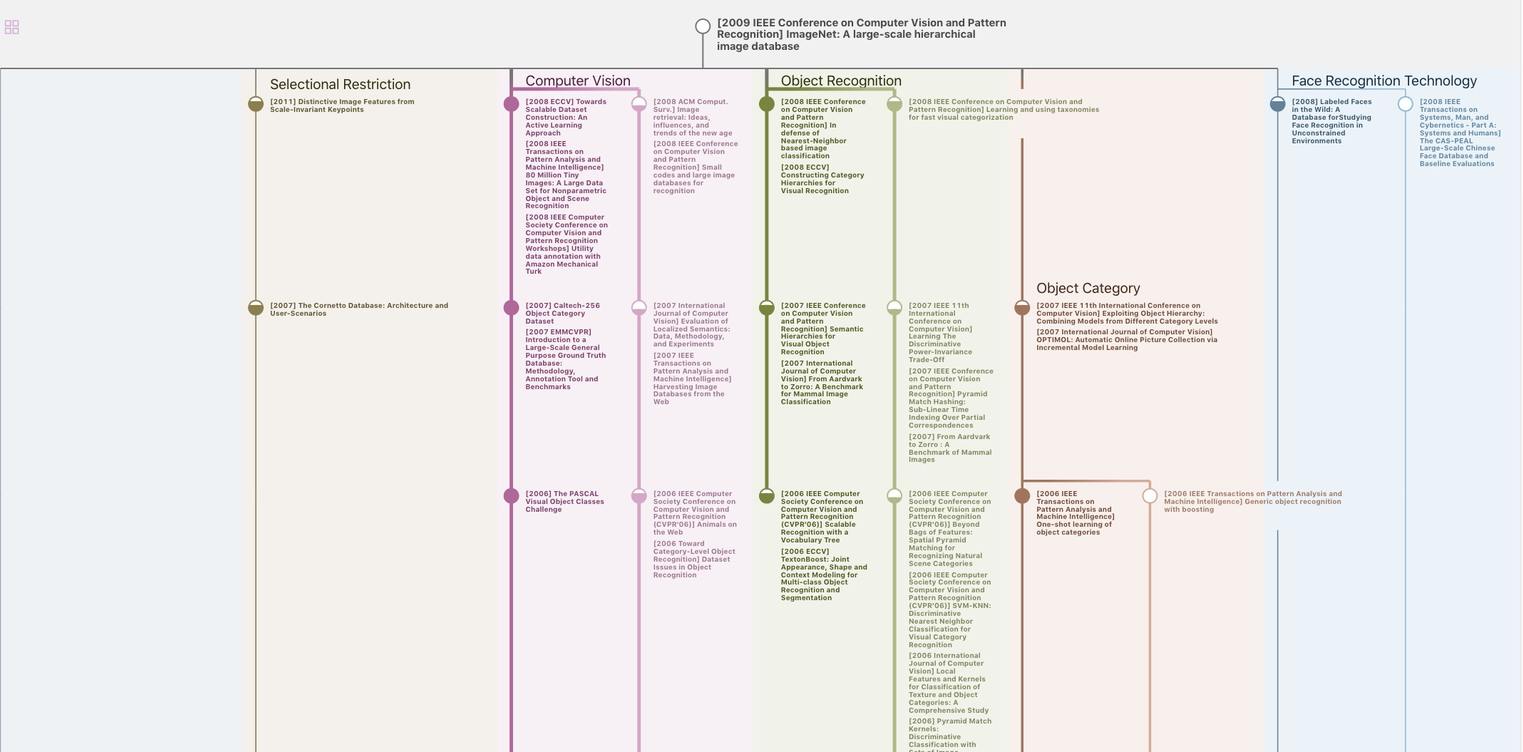
生成溯源树,研究论文发展脉络
Chat Paper
正在生成论文摘要