A two-stage multi-view learning framework based computer-aided diagnosis of liver tumors with contrast enhanced ultrasound images.
CLINICAL HEMORHEOLOGY AND MICROCIRCULATION(2018)
Abstract
OBJECTIVE: With the fast development of artificial intelligence techniques, we proposed a novel two-stage multi-view learning framework for the contrast-enhanced ultrasound (CEUS) based computer-aided diagnosis for liver tumors, which adopted only three typical CEUS images selected from the arterial phase, portal venous phase and late phase. MATERIALS AND METHODS: In the first stage, the deep canonical correlation analysis (DCCA) was performed on three image pairs between the arterial and portal venous phases, arterial and delayed phases, and portal venous and delayed phases respectively, which then generated total six-view features. While in the second stage, these multi-view features were then fed to a multiple kernel learning (MKL) based classifier to further promote the diagnosis result. Two MKL classification algorithms were evaluated in this MKL-based classification framework. We evaluated proposed DCCA-MKL framework on 93 lesions (47 malignant cancers vs. 46 benign tumors). RESULTS: The proposed DCCA-MKL framework achieved the mean classification accuracy, sensitivity, specificity, Youden index, false positive rate, and false negative rate of 90.41 +/- 5.80%, 93.56 +/- 5.90%, 86.89 +/- 9.38%, 79.44 +/- 11.83%, 13.11 +/- 9.38% and 6.44 +/- 5.90%, respectively, by soft margin MKL classifier. CONCLUSION: The experimental results indicate that the proposed DCCA-MKL framework achieves best performance for discriminating benign liver tumors from malignant liver cancers. Moreover, it is also proved that the three-phase CEUS image based CAD is feasible for liver tumors with the proposed DCCA-MKL framework.
MoreTranslated text
Key words
Contrast-enhanced ultrasound,artificial intelligence,liver tumor,deep canonical correlation analysis,multiple kernel learning
AI Read Science
Must-Reading Tree
Example
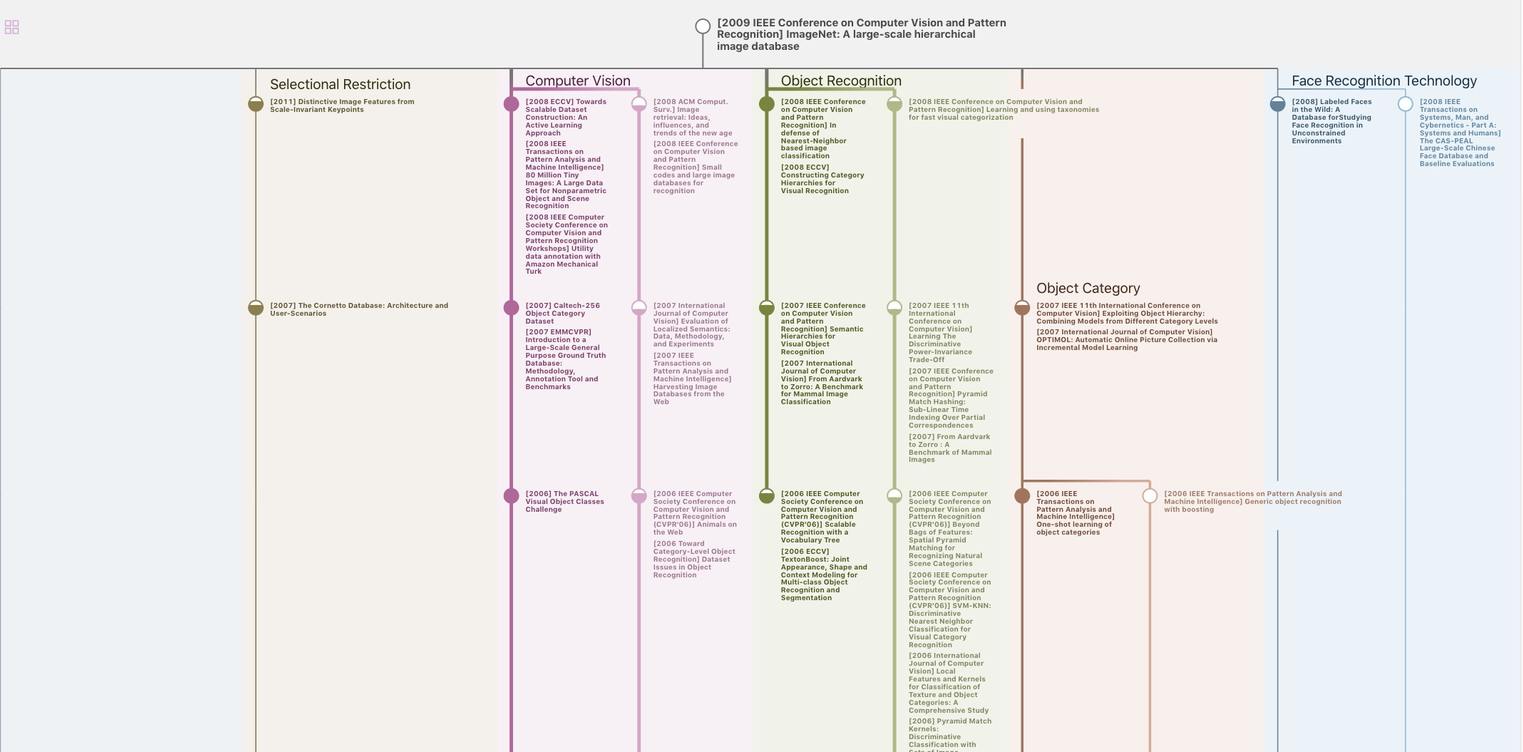
Generate MRT to find the research sequence of this paper
Chat Paper
Summary is being generated by the instructions you defined