Unsupervised Analysis of Transcriptomics in Bacterial Sepsis Across Multiple Datasets Reveals Three Robust Clusters.
CRITICAL CARE MEDICINE(2018)
摘要
Objectives: To find and validate generalizable sepsis subtypes using data-driven clustering. Design: We used advanced informatics techniques to pool data from 14 bacterial sepsis transcriptomic datasets from eight different countries (n = 700). Setting: Retrospective analysis. Subjects: Persons admitted to the hospital with bacterial sepsis. Interventions: None. Measurements and Main Results: A unified clustering analysis across 14 discovery datasets revealed three subtypes, which, based on functional analysis, we termed Inflammopathic, Adaptive, and Coagulopathic. We then validated these subtypes in nine independent datasets from five different countries (n = 600). In both discovery and validation data, the Adaptive subtype is associated with a lower clinical severity and lower mortality rate, and the Coagulopathic subtype is associated with higher mortality and clinical coagulopathy. Further, these clusters are statistically associated with clusters derived by others in independent single sepsis cohorts. Conclusions: The three sepsis subtypes may represent a unifying framework for understanding the molecular heterogeneity of the sepsis syndrome. Further study could potentially enable a precision medicine approach of matching novel immunomodulatory therapies with septic patients most likely to benefit.
更多查看译文
关键词
cluster analysis,gene expression,machine learning,precision medicine,sepsis
AI 理解论文
溯源树
样例
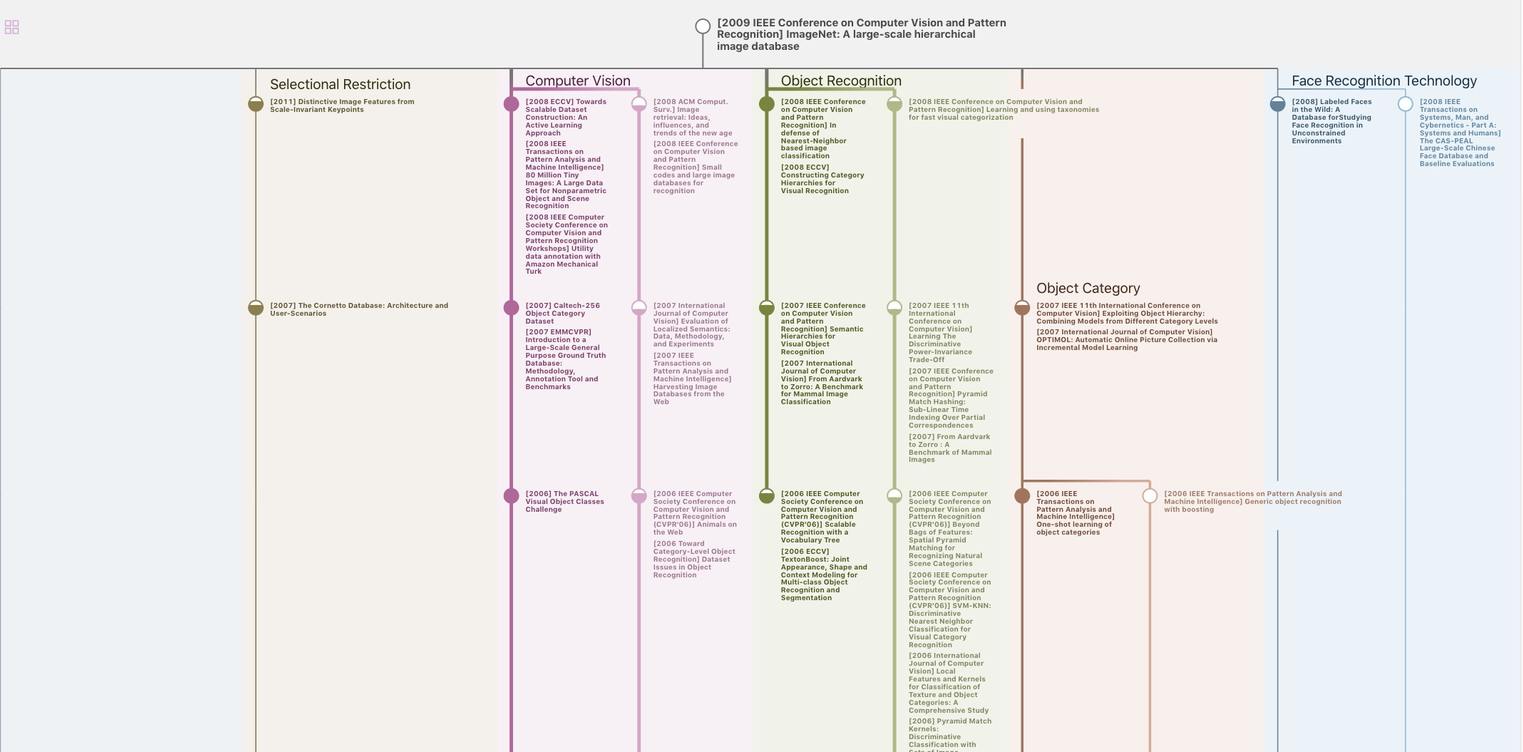
生成溯源树,研究论文发展脉络
Chat Paper
正在生成论文摘要