Classifiers for Accelerometer-Measured Behaviors in Older Women.
Medicine and science in sports and exercise(2017)
摘要
PURPOSE:Machine learning methods could better improve the detection of specific types of physical activities and sedentary behaviors from accelerometer data. No studies in older populations have developed and tested algorithms for walking and sedentary time in free-living daily life. Our goal was to rectify this gap by leveraging access to data from two studies in older women.
METHODS:In study 1, algorithms were developed and tested in a sample of older women (N = 39, age range = 55-96 yr) in the field. Women wore accelerometers and SenseCam (ground truth annotation) devices for 7 d, yielding 3191 h and 320 d of data. Images were annotated and time matched to accelerometer data, and random forest classifiers labeled behaviors (sitting, riding in a vehicle, standing still, standing moving, and walking/running). In study 2, we examined the concurrent validity of the algorithms using accelerometer data from an observed 400-m walk test (2983 min of data available) and 6 d of wearing both accelerometers and global positioning systems devices in a sample of 222 women (age range = 67-100; 313,290 min of data available). Analyses included sensitivity, specificity balanced accuracy, and precision, as appropriate, averaged over each test participant at the minute level for each behavior.
RESULTS:In study 1, the algorithms had 82.2% balanced accuracy. In study 2, the classifier had 87.9% accuracy for predicting walking. Overall machine learning classifiers and global positioning systems had 88.6% agreement.
CONCLUSIONS:Free-living algorithms for walking and sedentary time yielded high levels of accuracy and concurrent validity and can be applied to existing accelerometer data from older women.
更多查看译文
AI 理解论文
溯源树
样例
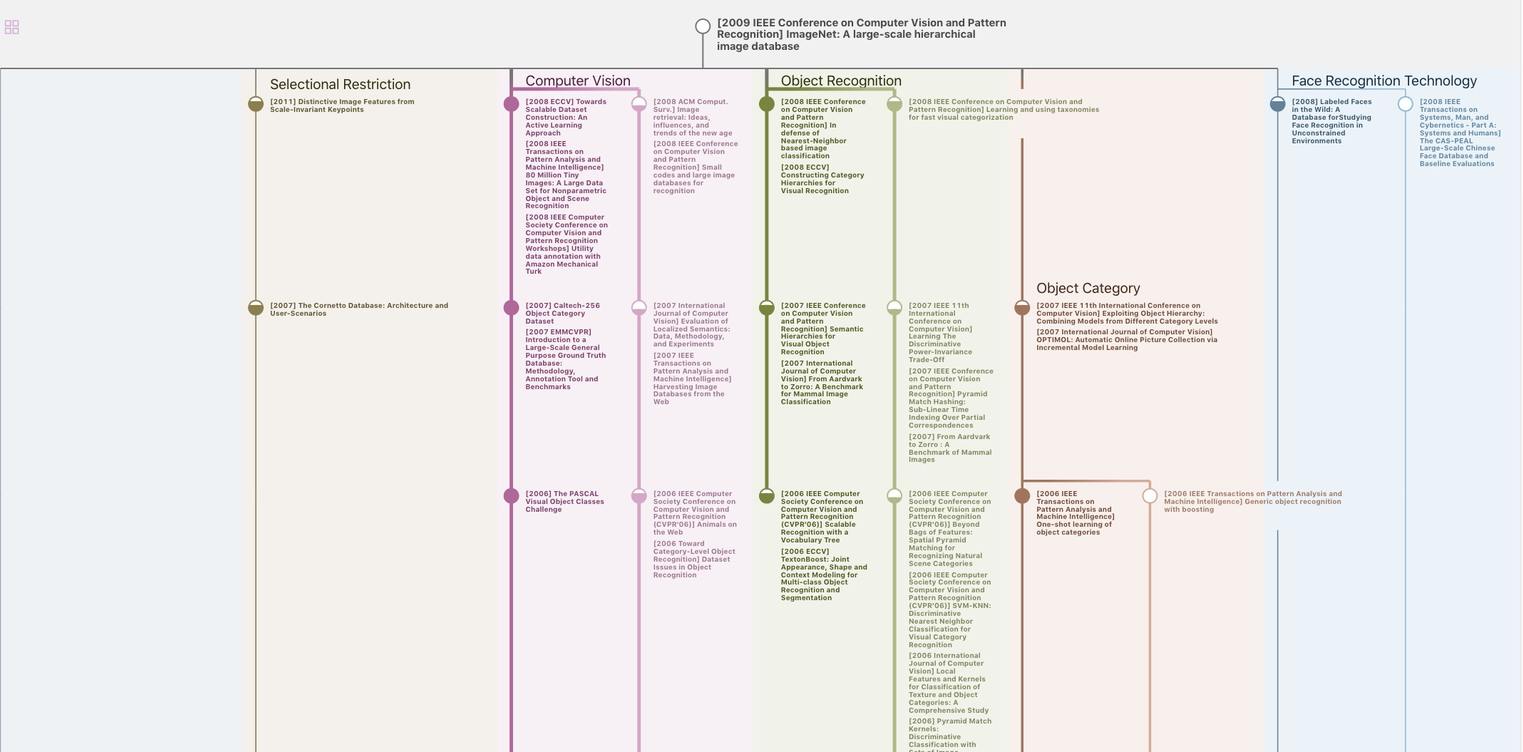
生成溯源树,研究论文发展脉络
Chat Paper
正在生成论文摘要